A Benchmark for Building Footprint Classification Using Orthorectified RGB Imagery and Digital Surface Models from Commercial Satellites
2017 IEEE Applied Imagery Pattern Recognition Workshop (AIPR)(2017)
摘要
Identifying building footprints is a critical and challenging problem in many remote sensing applications. Solutions to this problem have been investigated using a variety of sensing modalities as input. In this work, we consider the detection of building footprints from 3D Digital Surface Models (DSMs) created from commercial satellite imagery along with RGB orthorectified imagery. Recent public challenges (SpaceNet 1 and 2, DSTL Satellite Imagery Feature Detection Challenge, and the ISPRS Test Project on Urban Classification) approach this problem using other sensing modalities or higher resolution data. As a result of these challenges and other work, most publically available automated methods for building footprint detection using 2D and 3D data sources as input are meant for high-resolution 3D lidar and 2D airborne imagery, or make use of multispectral imagery as well to aid detection. Performance is typically degraded as the fidelity and post spacing of the 3D lidar data or the 2D imagery is reduced. Furthermore, most software packages do not work well enough with this type of data to enable a fully automated solution. We describe a public benchmark dataset consisting of 50 cm DSMs created from commercial satellite imagery, as well as coincident 50 cm RGB orthorectified imagery products. The dataset includes ground truth building outlines and we propose representative quantitative metrics for evaluating performance. In addition, we provide lessons learned and hope to promote additional research in this field by releasing this public benchmark dataset to the community.
更多查看译文
关键词
automated building footprint detection,benchmark dataset,public prize challenge,Urban 3D Challenge,remote sensing,commercial satellite imagery,2D RGB orthorectified imagery,3D digital surface models,urban 3D scene modeling,multi-view stereo imagery
AI 理解论文
溯源树
样例
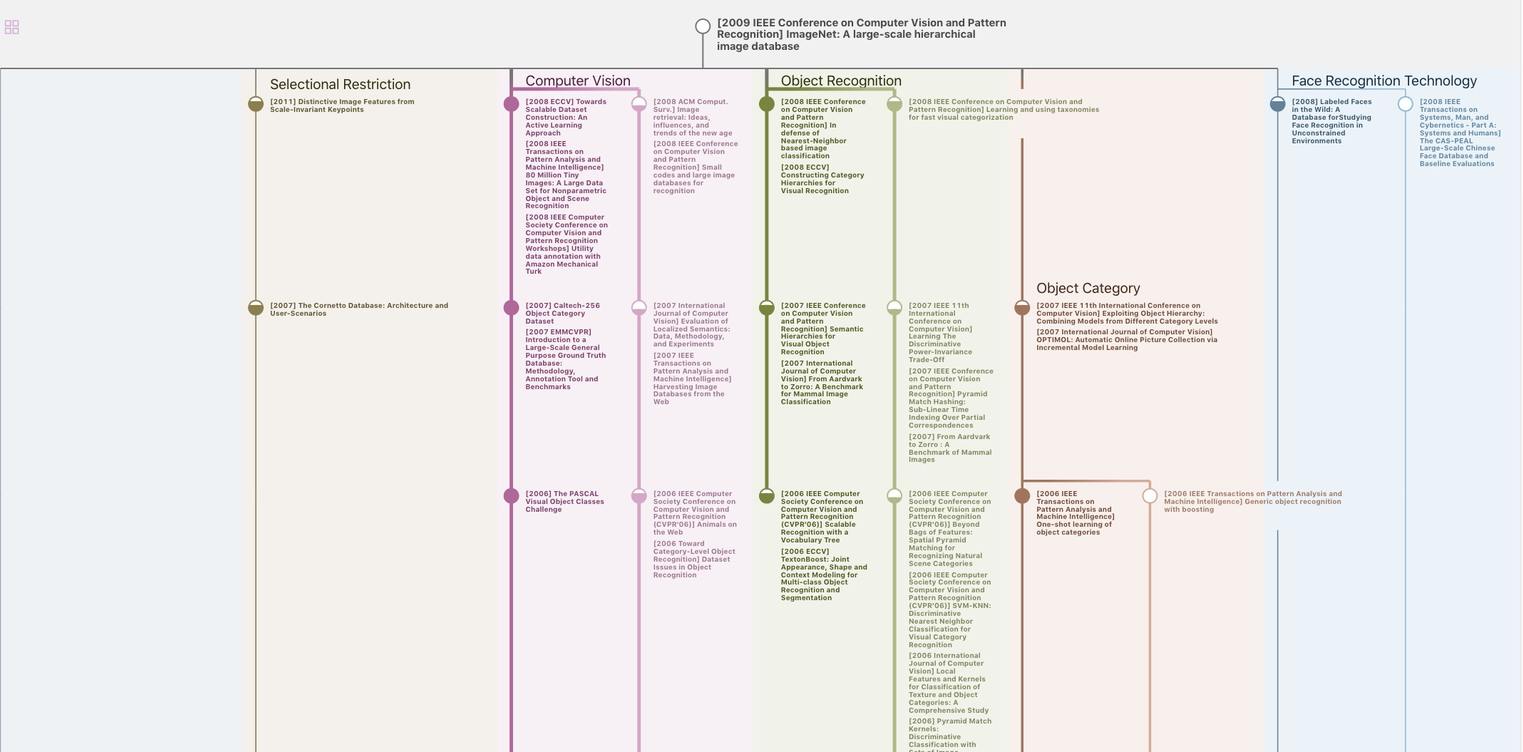
生成溯源树,研究论文发展脉络
Chat Paper
正在生成论文摘要