Learning-Based Prediction Of Package Power Delivery Network Quality
24TH ASIA AND SOUTH PACIFIC DESIGN AUTOMATION CONFERENCE (ASP-DAC 2019)(2019)
摘要
Power Delivery Network (PDN) is a critical component in modern System-on-Chip (SoC) designs. With the rapid development in applications, the quality of PDN, especially Package (PKG) PDN, determines whether a sufficient amount of power can be delivered to critical computing blocks. In conventional PKG design, PDN design typically takes multiple weeks including many manual iterations for optimization. Also, there is a large discrepancy between (i) quick simulation tools used for quick PDN quality assessment during the design phase, and (ii) the golden extraction tool used for signoff. This discrepancy may introduce more iterations. In this work, we propose a learning-based methodology to perform PKG PDN quality assessment both before layout (when only bump/ball maps, but no package routing, are available) and after layout (when routing is completed but no signoff analysis has been launched). Our contributions include (i) identification of important parameters to estimate the achievable PKG PDN quality in terms of bump inductance; (ii) the avoidance of unnecessary manual trial and error overheads in PKG PDN design; and (iii) more accurate design-phase PKG PDN quality assessment. We validate accuracy of our predictive models on PKG designs from industry. Experimental results show that, across a testbed of 17 industry PKG designs, we can predict bump inductance with an average absolute percentage error of 21.2% or less, given only pinmap and technology information. We improve prediction accuracy to achieve an average absolute percentage error of 17.5% or less when layout information is considered.
更多查看译文
关键词
Power Delivery Network,Prediction Model,Prediction Accuracy,Average Error,Design Software,Simulation Tool,Average Absolute Error,Quick Tool,Average Percentage Error,Model Parameters,Accuracy Of Model,Training Dataset,Support Vector Machine,Artificial Neural Network,Test Dataset,Trial Design,Coupling Coefficient,Activity Prediction,Nonlinear Algorithm,Multivariate Adaptive Regression Splines,Early Design Stages,Selection Of Model Parameters,Flip-chip,Commercial Tools,Electromagnetic Simulation,Lumped Model,Prediction Methodology,Loop Inductance,Design Cycle
AI 理解论文
溯源树
样例
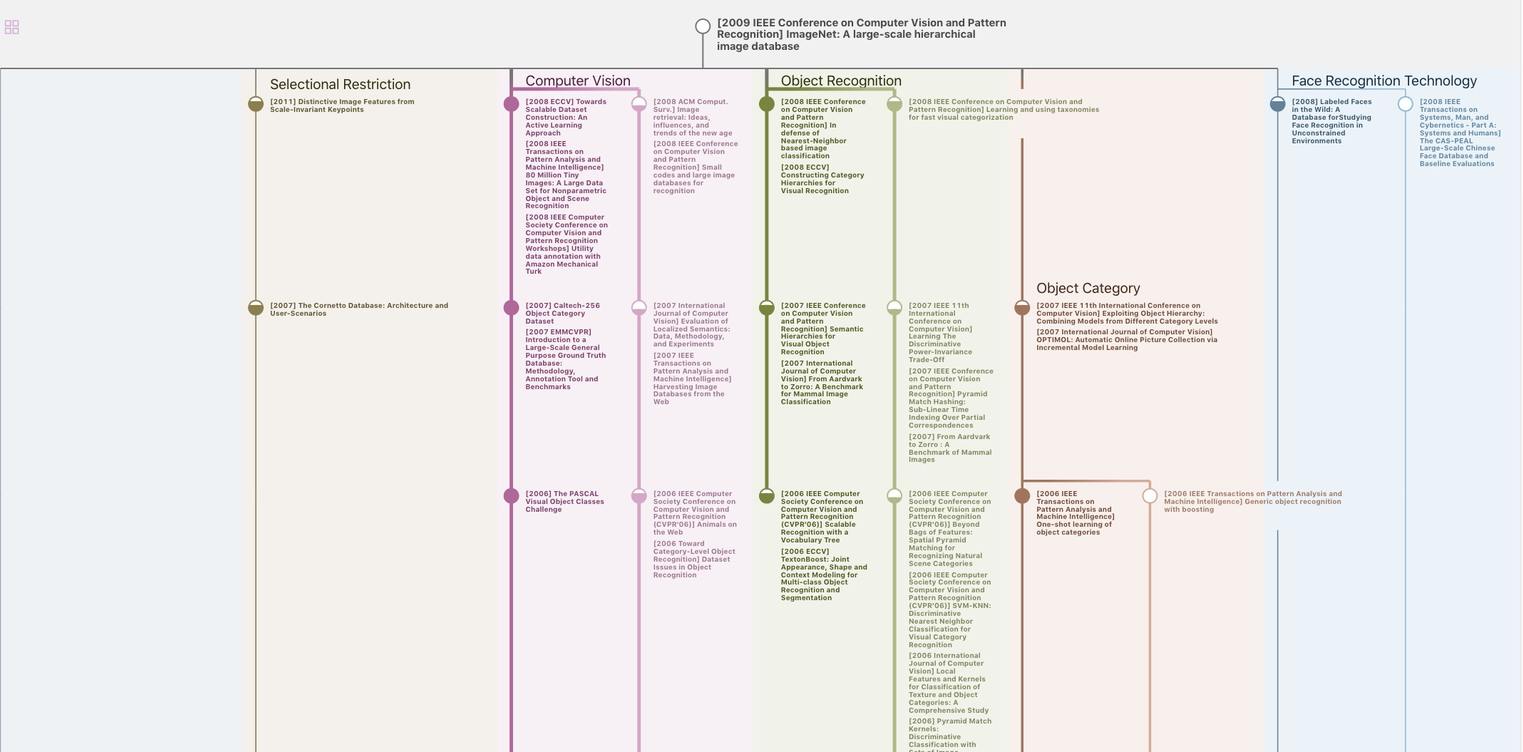
生成溯源树,研究论文发展脉络
Chat Paper
正在生成论文摘要