A Hierarchical Approach to Skin Lesion Classification.
COMAD/CODS(2019)
摘要
Deep Neural Networks (DNNs) are powerful models that can perform extremely complex tasks with a high level of accuracy. Convolutional Neural Networks (CNNs) are extensions of DNNs which specialize in dealing with data possessing spatial representations. We attempt to build a CNN model that can learn to classify a dermoscopic image of skin melanoma into one of seven categories {NV, BCC, BKL, MEL, DF, VASC, AKIEC} belonging to the HAM10000 dataset, each having a varying criticality. We arrange a set of CNN models in a hierarchical structure which, we believe, helps each sub-model gain specific intuitions about the differentiating factors present in a class of images in question without exploiting any false bias. We denote each level in the hierarchy as a 'stage'. In this paper, we present three such hierarchical combinations and conclude that the 5-stage hierarchical classifier yields the best results, followed by the 2-stage hierarchical classifier. We also conclude that, in spite of heavy class imbalance, data augmentation can significantly aid a CNN in extracting a good set of features so as to accurately represent the minority classes.
We have also open-sourced our implementations. All our models and scripts are available on our Gi tHub repository.
更多查看译文
关键词
Convolutional Neural Networks, Deep Learning, ISIC 2018, Skin Melanoma, Transfer Learning
AI 理解论文
溯源树
样例
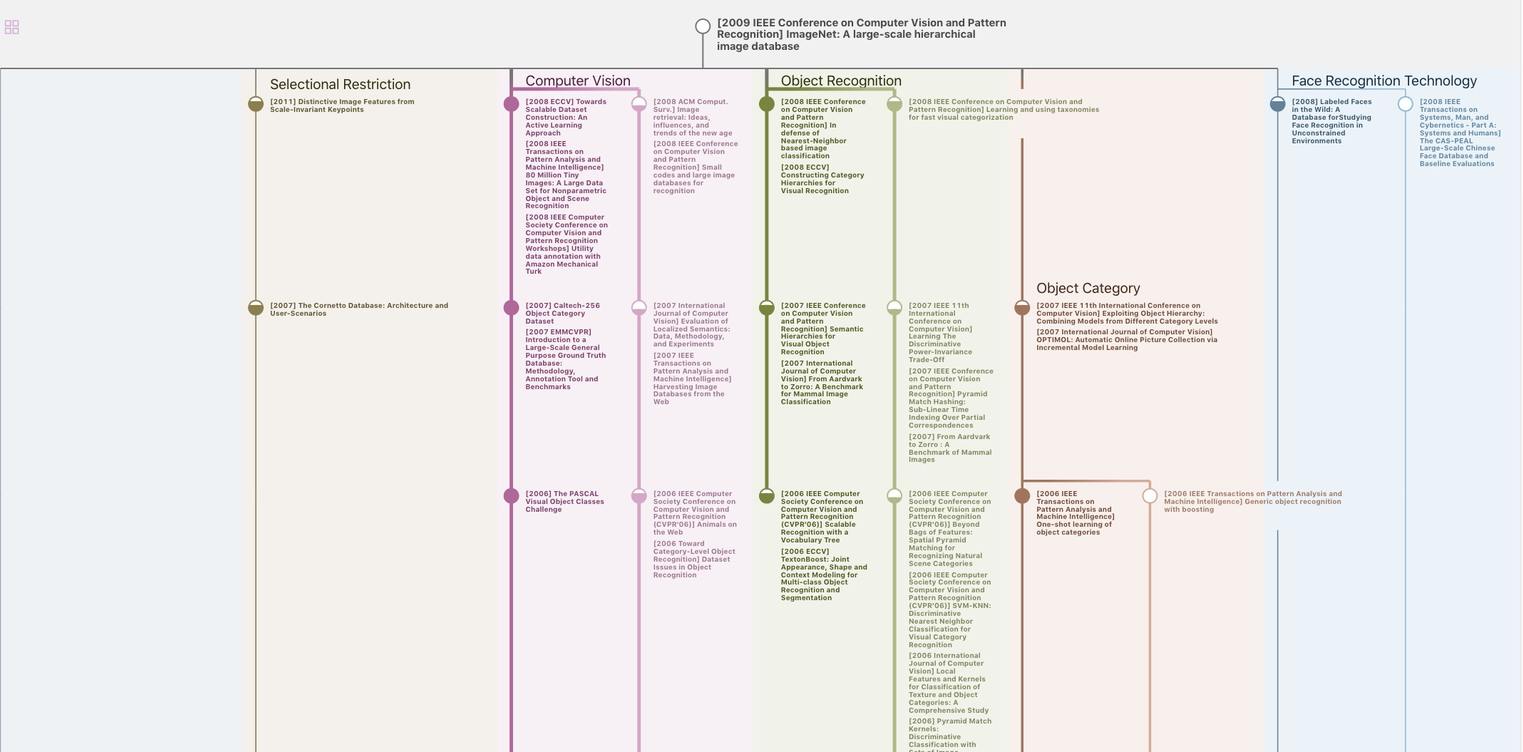
生成溯源树,研究论文发展脉络
Chat Paper
正在生成论文摘要