A Twin Memristor Synapse for Spike Timing Dependent Learning in Neuromorphic Systems
2018 31st IEEE International System-on-Chip Conference (SOCC)(2018)
摘要
Neuromorphic systems consist of a framework of spiking neurons interconnected via plastic synaptic junctures. The discovery of a two terminal passive nanoscale memristive device has spurred great interest in the realization of memristive plastic synapses in neural networks. In this work, a synapse structure is presented that utilizes a pair of memristors, to implement both positive and negative weights. The working scheme of this synapse as an electrical interlink between neurons is explained, and the relative timing of their spiking events is analyzed, which leads to a modulation of the synaptic weight in accordance with the spike-timing-dependent plasticity (STDP) rule. A digital pulse width modulation technique is proposed to achieve these variable changes to the synaptic weight. The synapse architecture presented is shown to have high accuracy when used in neural networks for classification tasks. Lastly, the energy requirement of the system during various phases of operation is presented.
更多查看译文
关键词
synapse architecture,modulation technique,spike-timing-dependent plasticity rule,synaptic weight,spiking events,relative timing,electrical interlink,working scheme,negative weights,positive weights,synapse structure,neural networks,memristive plastic synapses,terminal passive nanoscale memristive device,plastic synaptic junctures,spiking neurons,neuromorphic systems,spike timing dependent learning,twin memristor synapse
AI 理解论文
溯源树
样例
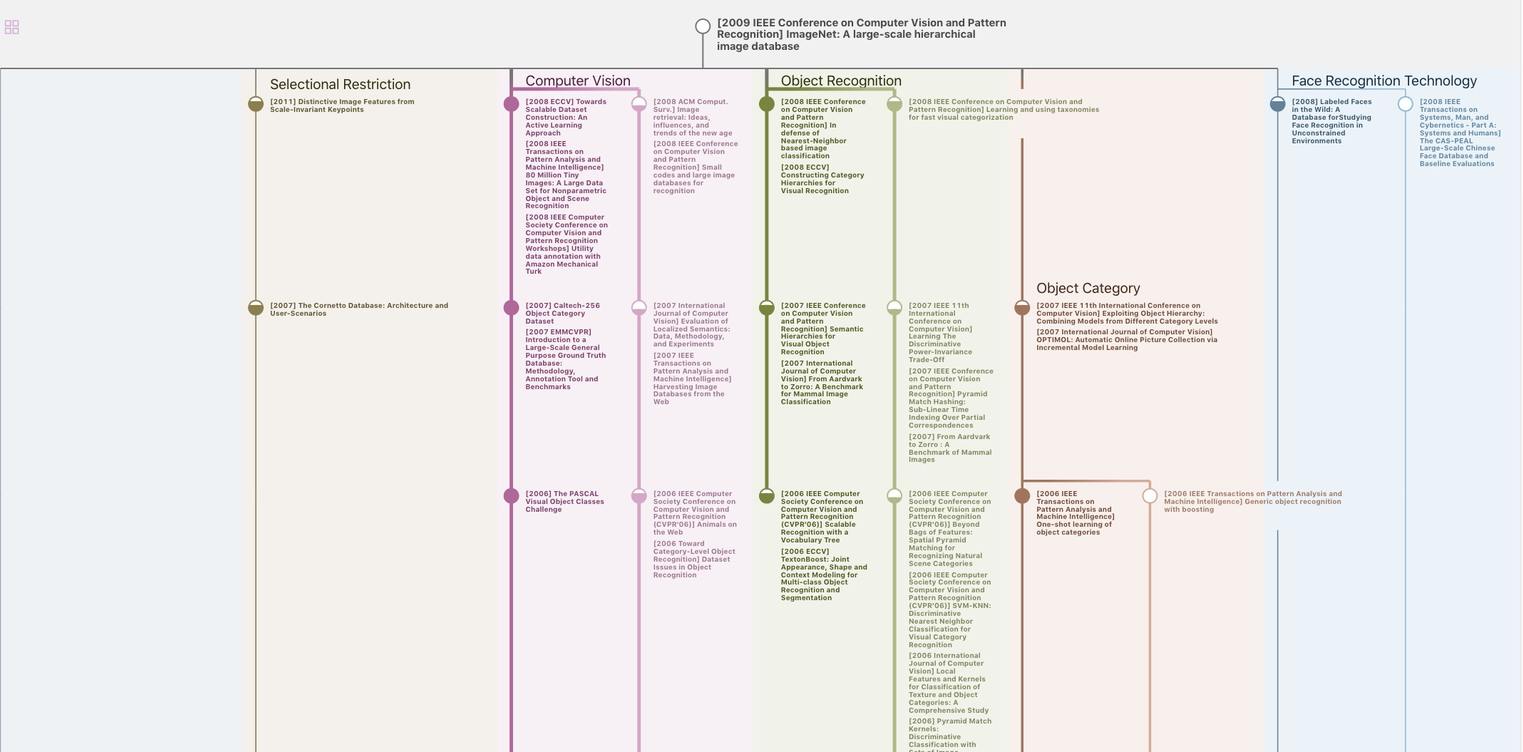
生成溯源树,研究论文发展脉络
Chat Paper
正在生成论文摘要