Fast Clustering with Flexible Balance Constraints
2018 IEEE International Conference on Big Data (Big Data)(2018)
摘要
Balanced clustering aims at partitioning a dataset with roughly even cluster sizes while exploiting the intrinsic structure of the data. Despite attracting increased attention recently in both the academia and the industry, most existing balanced clustering algorithms still have high run time complexities that prevent them from being applied to large datasets. To cope with this challenge, we propose a Fast Clustering with Flexible balance Constraints FCFC, a simple, fast and effective clustering algorithm that can deal with flexible balance constraints. In essence, FCFC employs K-means as the core clustering algorithm and the cluster size variances as the penalty for imbalance. The objective function consists of the combined classical K-means clustering cost as well as the imbalance penalty. By exploiting a new insight of the second term, FCFC is able to employ an efficient K-means-like optimization procedure that can scale to big datasets. Furthermore, we also extend our model for multiple balance constraints with theoretical supports. Extensive experimental results show that our method exceeds several state-of-the-art methods by large margins in terms of efficiency and clustering quality. Finally, a real-world application for Bing search is provided, where data are organized in multiple machines with data size and query frequency balancing objectives. In the simulated scenario, our solution achieves the same fidelity score while reduces cost by 75% compared to the baseline method.
更多查看译文
关键词
Clustering,K-means,Balance Constraints
AI 理解论文
溯源树
样例
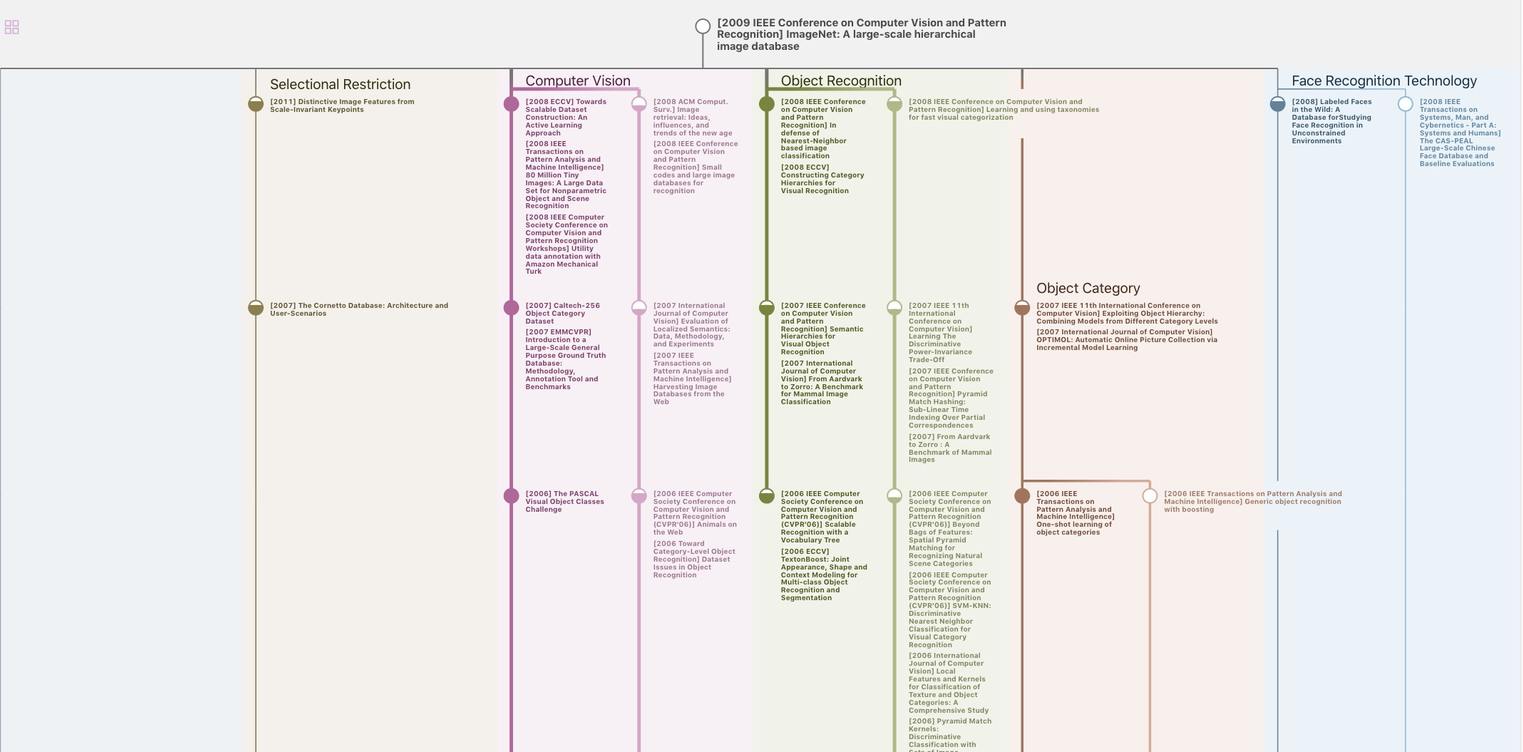
生成溯源树,研究论文发展脉络
Chat Paper
正在生成论文摘要