Predicting Consumer Level Brand Preferences Using Persistent Mobility Patterns
2018 IEEE INTERNATIONAL CONFERENCE ON BIG DATA (BIG DATA)(2018)
摘要
In the era of digital marketing, it is imperative for brands to reach target segment precisely to maximize their marketing ROI. In most cases, the target segments are created based on broad demographic and psychographic traits without any knowledge of the consumer brand preferences. In this paper, we propose a methodology to predict the individual level consumer brand preference based on the historical brand visitation patterns. We believe that this is the first attempt and a successful deployment to predict individual consumer level brand preference at large scale. In general, it is very hard to accumulate longitudinal location data of consumers. As Mobile Ad ecosystem is one of the major source in generating geospatial and temporal data which provides rich information about the mobility patterns of the mobile devices (addressed as consumers hereafter). We harnessed the power of spatio-temporal data received in our Demand Side Platform which is accumulated over a period of more than 2 years to predict brand preferences. Further, we carefully curated the brands' POI data across different countries to derive the historical brand visitation patterns of consumers. Then, this visitation pattern is used to predict propensity score for a given brand for a given consumer. We have employed a recommender system approach using distributed Alternating Least Squares - Weighted Regularization (ALS-WR) based matrix factorization to predict the brand propensities at scale. Rigorous experiments are conducted to validate the model's performance against the benchmark. Our model showed twice as much lift compared to the benchmark with 40% average recall across the brands for the Indonesian market. The full pipeline is developed and deployed in production for the countries of our business's interest such as Indonesia, Thailand, Philippines, Singapore and Malaysia in our AWS EMR1 cloud environment.
更多查看译文
关键词
Recommender Systems, Brand Propensity, Large Scale Distributed Machine Learning, Audience targeting
AI 理解论文
溯源树
样例
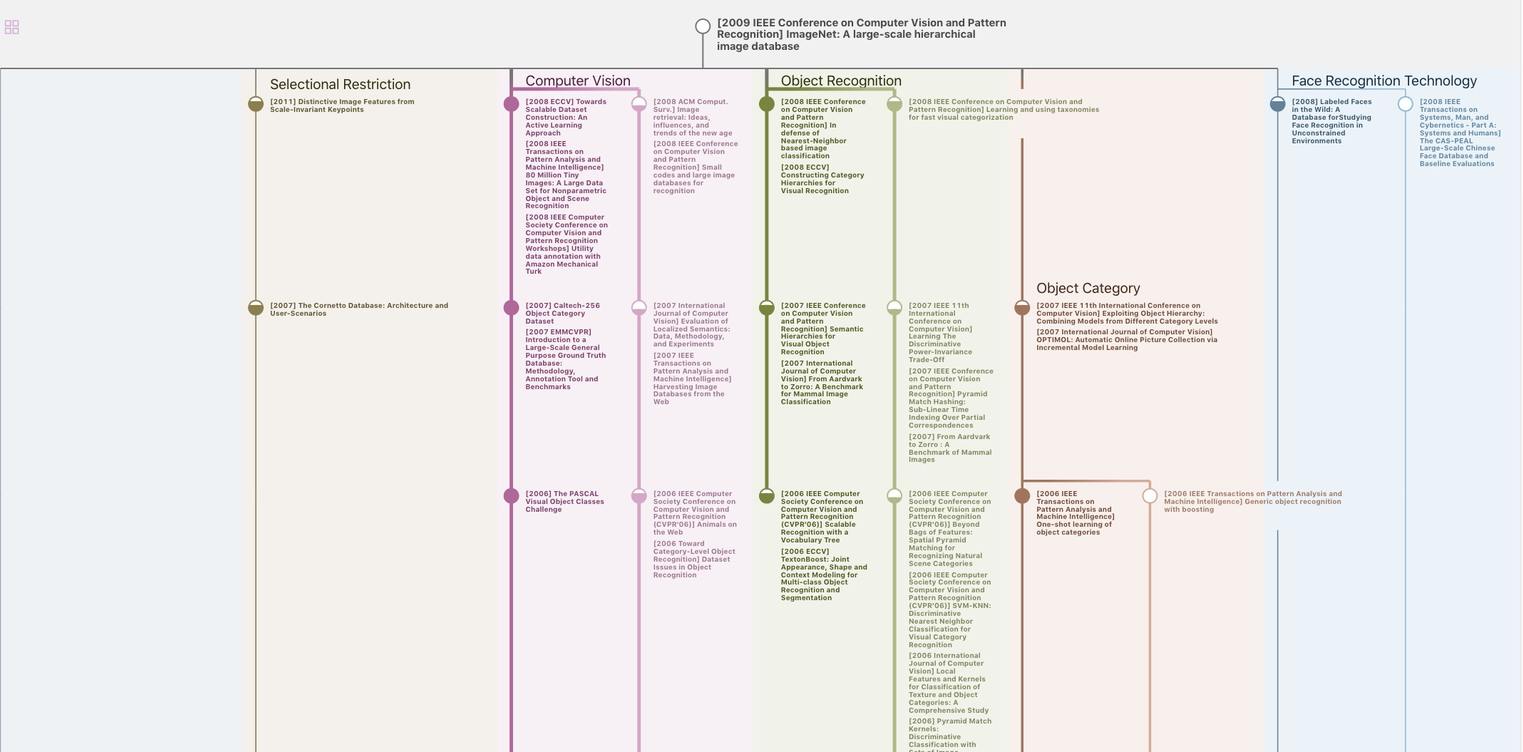
生成溯源树,研究论文发展脉络
Chat Paper
正在生成论文摘要