Influence-Directed Explanations for Deep Convolutional Networks
2018 IEEE International Test Conference (ITC)(2018)
摘要
We study the problem of explaining a rich class of behavioral properties of deep neural networks. Distinctively, our influence-directed explanations approach this problem by peering inside the network to identify neurons with high influence on a quantity and distribution of interest, using an axiomatically-justified influence measure, and then providing an interpretation for the concepts these neurons represent. We evaluate our approach by demonstrating a number of its unique capabilities on convolutional neural networks trained on ImageNet. Our evaluation demonstrates that influence-directed explanations (1) identify influential concepts that generalize across instances, (2) can be used to extract the "essence" of what the network learned about a class, and (3) isolate individual features the network uses to make decisions and distinguish related classes.
更多查看译文
关键词
axiomatically-justified influence measure,convolutional neural networks,deep convolutional networks,deep neural networks,influence-directed explanations approach,network learning
AI 理解论文
溯源树
样例
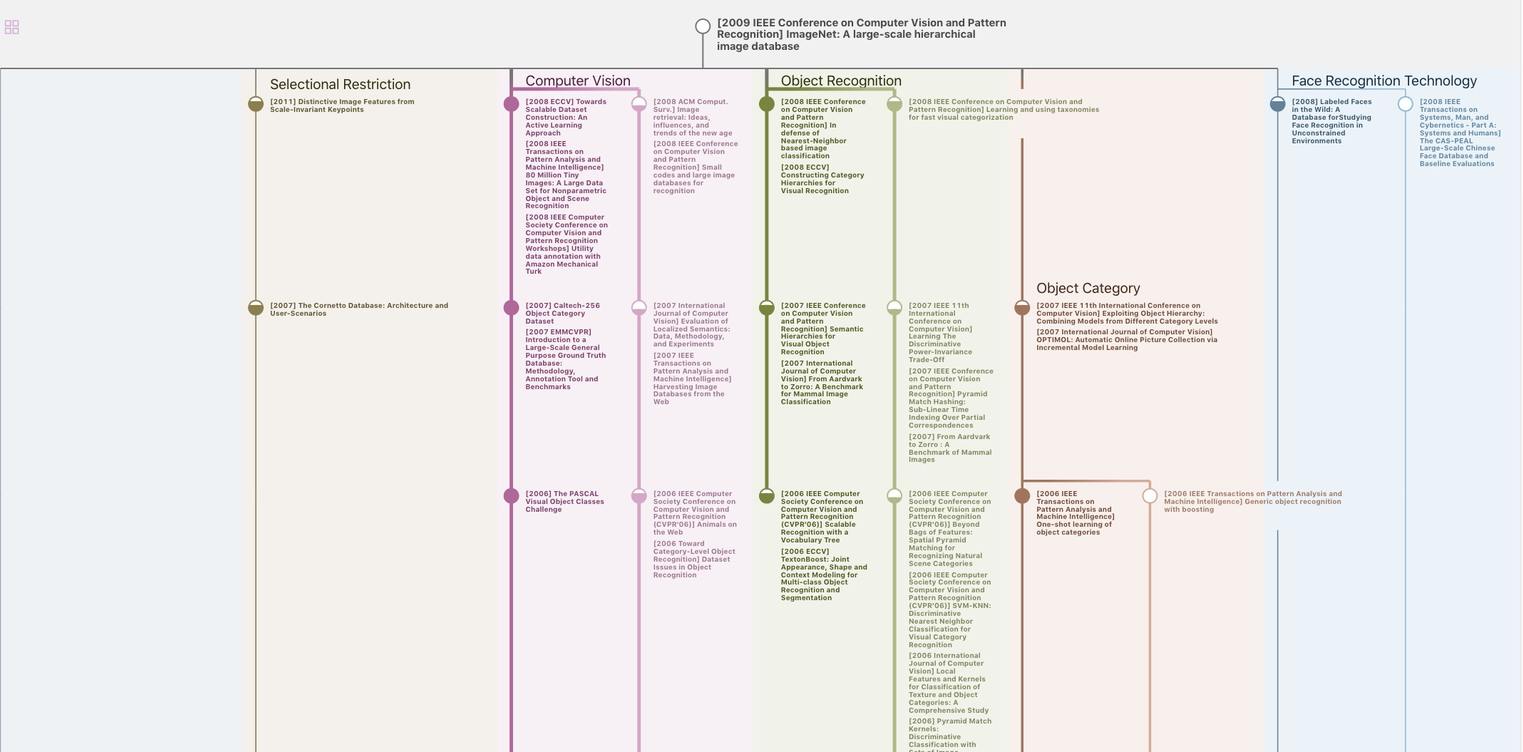
生成溯源树,研究论文发展脉络
Chat Paper
正在生成论文摘要