Differentiating and Predicting Cyberattack Behaviors Using LSTM
2018 IEEE Conference on Dependable and Secure Computing (DSC)(2018)
摘要
Classifying and predicting cyberattack behaviors are outstanding challenges due to the changing and broad attack surfaces as attackers penetrate into enterprise networks. The rise of Recurrent Neural Networks (RNNs) for temporally structured data in machine learning presents an opportunity to address these challenges, though it would require sufficient data and reasonable labels indicative of attack behaviors. This paper presents the use of RNNs to model penetration behaviors exhibited by ten teams in the 2017 Collegiate Penetration Testing Competition (CPTC'17). The Long-Short-Term-Memory (LSTM) models obtained by training on the CPTC data enable the assessment of the differentiability of attack behaviors across teams and the predictability of future actions. This first-of-its kind attempt presents observations and insights for how earlier attack actions may or may not be indicative of future behaviors. The paper concludes with future considerations to integrate the LSTM models and enable predictive analytics to defend against complex, multistage cyberattacks.
更多查看译文
关键词
cyberattack behaviors,broad attack surfaces,enterprise networks,RNNs,temporally structured data,machine learning,attack behaviors,penetration behaviors,predictability,future behaviors,LSTM models,predictive analytics,complex cyberattacks,multistage cyberattacks,recurrent neural networks,long short term memory models
AI 理解论文
溯源树
样例
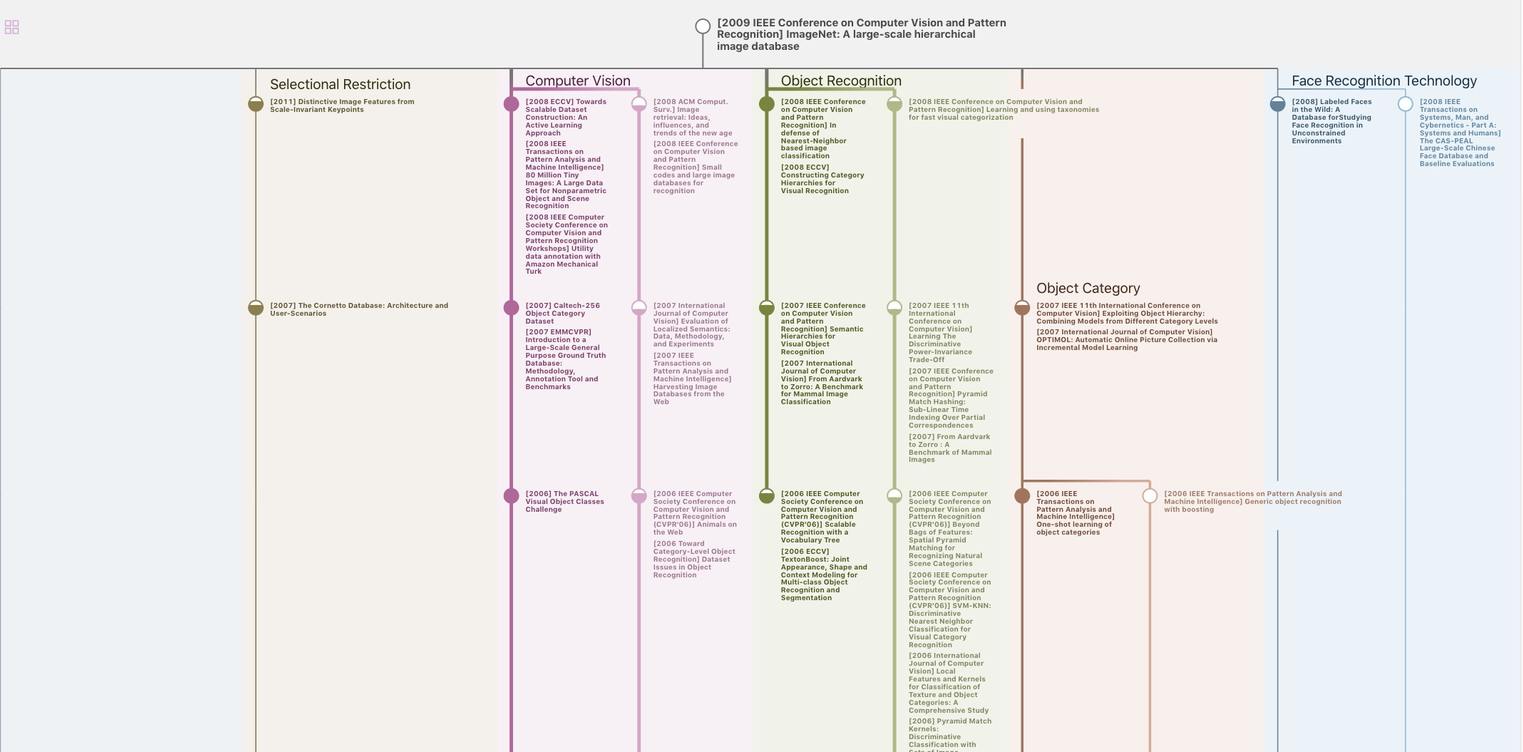
生成溯源树,研究论文发展脉络
Chat Paper
正在生成论文摘要