A Data-Driven Koopman Model Predictive Control Framework For Nonlinear Partial Differential Equations
2018 IEEE CONFERENCE ON DECISION AND CONTROL (CDC)(2018)
摘要
The Koopman operator theory is an increasingly popular formalism of dynamical systems theory which enables analysis and prediction of the state space dynamics from measurement data. Building on the recent development of the Koopman model predictive control framework [14], we propose a methodology for closed-loop feedback control of nonlinear partial differential equations in a fully data-driven and model-free manner. In the first step, we compute a Koopman-linear representation of the system using a variation of the extended dynamic mode decomposition algorithm and then we apply the model predictive control to the constructed linear model. Our methodology is also capable of utilizing sparse measurements by incorporating delay-embedding of the available data into the identification and control processes. We illustrate the application of this methodology for the periodic Burgers' equation and the boundary control of fluid flow governed by the Navier-Stokes equations in a two-dimensional cavity.
更多查看译文
关键词
extended dynamic mode decomposition algorithm,control processes,boundary control,data-driven Koopman model predictive control framework,nonlinear partial differential equations,Koopman operator theory,dynamical systems theory,state space dynamics,closed-loop feedback control,Koopman-linear representation,periodic Burgers equation
AI 理解论文
溯源树
样例
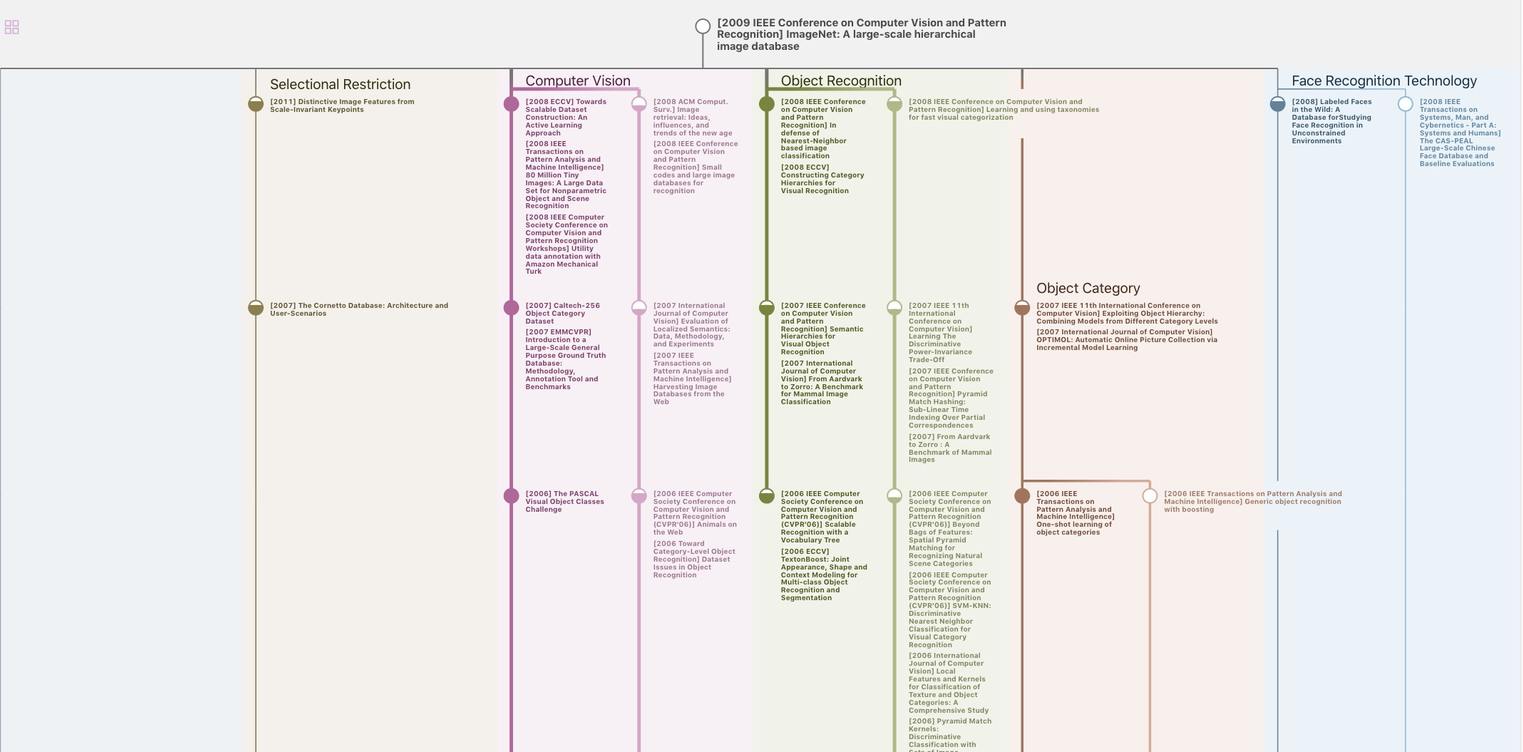
生成溯源树,研究论文发展脉络
Chat Paper
正在生成论文摘要