Towards Precise Vehicle-Free Point Cloud Mapping: An On-Vehicle System With Deep Vehicle Detection And Tracking
2018 IEEE INTERNATIONAL CONFERENCE ON SYSTEMS, MAN, AND CYBERNETICS (SMC)(2018)
摘要
While 3D LiDAR has become a common practice for more and more autonomous driving systems, precise 3D mapping and robust localization is of great importance. However, current 3D map is always noisy and unreliable due to the existence of moving objects, leading to worse localization. In this paper, we propose a general vehicle-free point cloud mapping framework for better on-vehicle localization. For each laser scan, vehicle points are detected, tracked and then removed. Simultaneously, 3D map is reconstructed by registering each vehicle-free laser scan to global coordinate based on GPS/INS data.Instead of direct 3D object detection from point cloud, we first detect vehicles from RGB images using the proposed YVDN (YOLOv2 Vehicle Detection Network). In case of false or missing detection, which may result in the existence of vehicles in the map, we propose the K-Frames forward-backward object tracking algorithm to link detection from neighborhood images. Laser scan points falling into the detected bounding boxes are then removed. We conduct our experiments on the Oxford RobotCar Dataset [1] and show the qualitative results to validate the feasibility of our vehicle-free 3D mapping system. Besides, our vehicle-free mapping system can be generalized to any autonomous driving system equipped with LiDAR, camera and/or GPS.
更多查看译文
关键词
Vehicle-free 3D mapping, Point Cloud, object detection, YOLOv2, Lucas-Kanade tracker
AI 理解论文
溯源树
样例
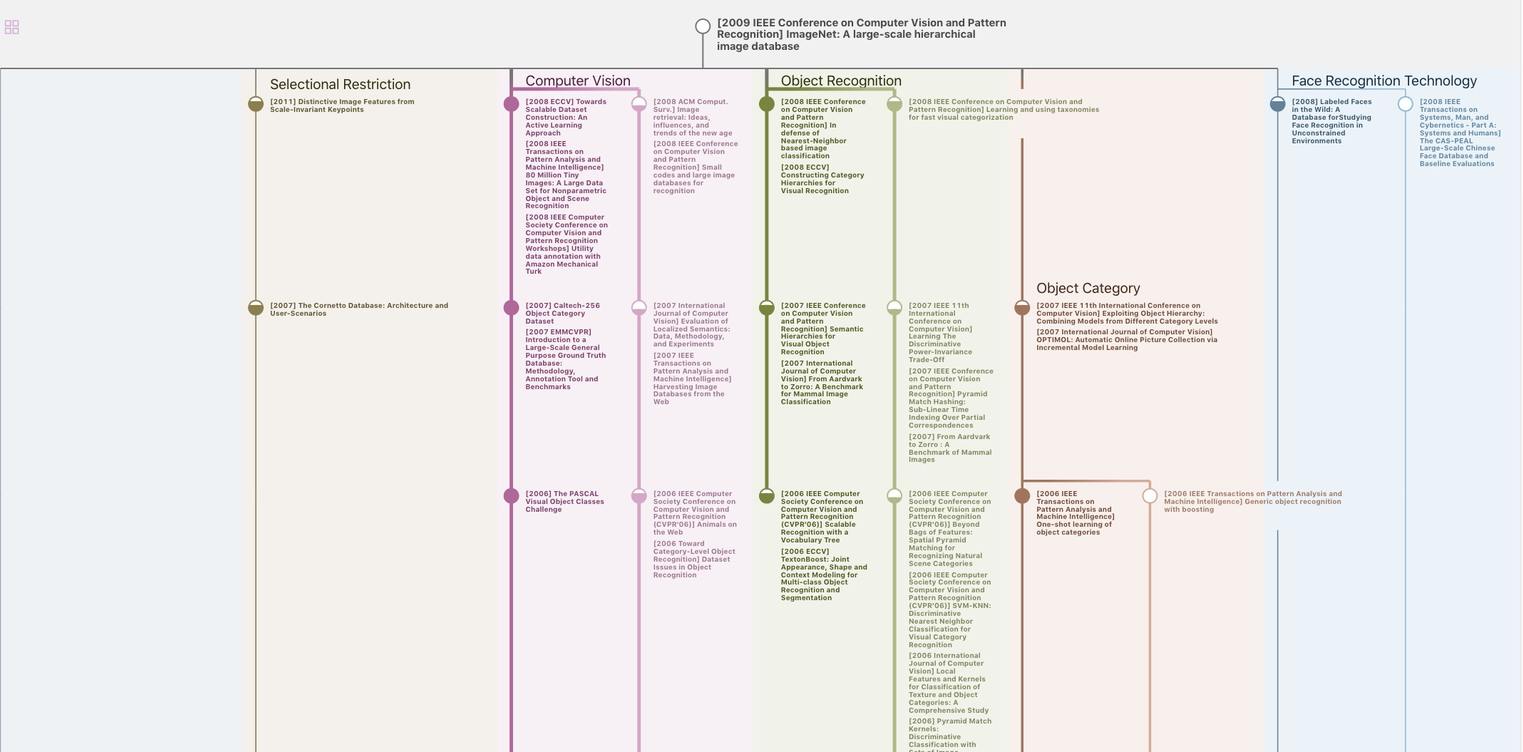
生成溯源树,研究论文发展脉络
Chat Paper
正在生成论文摘要