Deep Neural Networks For Forecasting Single-Trial Event-Related Neural Activity
2018 IEEE INTERNATIONAL CONFERENCE ON SYSTEMS, MAN, AND CYBERNETICS (SMC)(2018)
摘要
In this work, we propose a deep neural network to forecast single-trial event-related electroencephalographic (EEG) activity using observed pre-event EEG data. Forecasting event-related potentials (ERPs) have a number of potential benefits for brain-computer interface (BCI) systems. Accurate predictions of neural responses can reduce the latencies of neural signal classification algorithms and closed-loop feedback systems. Various models of ERPs propose that there exists a causal dependency between post-event neural responses and ongoing pre-event neural dynamics. Accordingly, we implement a deep neural network that extracts features from pre-event data in order to predict a single-trial ERP. To capture the variability of a single trial, the network constructs the post-event waveform in two parts: 1) generating ongoing neural activity and 2) generating event-related components comprising the ERP. We evaluate our model by forecasting 500 milliseconds of single channel post-event data from a Rapid Series Visual Presentation (RSVP) task. Our results indicate a significant increase in forecasting performance compared to baseline methods, suggesting that deep neural networks can extract informative features from pre-event EEG data in order to generate a prediction of the post-event waveform.
更多查看译文
关键词
deep neural network,single-trial event-related electroencephalographic activity,neural signal classification algorithms,post-event neural responses,single-trial ERP,post-event waveform,single channel post-event data,event-related potentials,single-trial event-related neural activity,preevent EEG data,preevent neural dynamics,brain-computer interface systems,brain-computer interface systems,closed-loop feedback systems,feature extraction,event-related components,rapid series visual presentation task
AI 理解论文
溯源树
样例
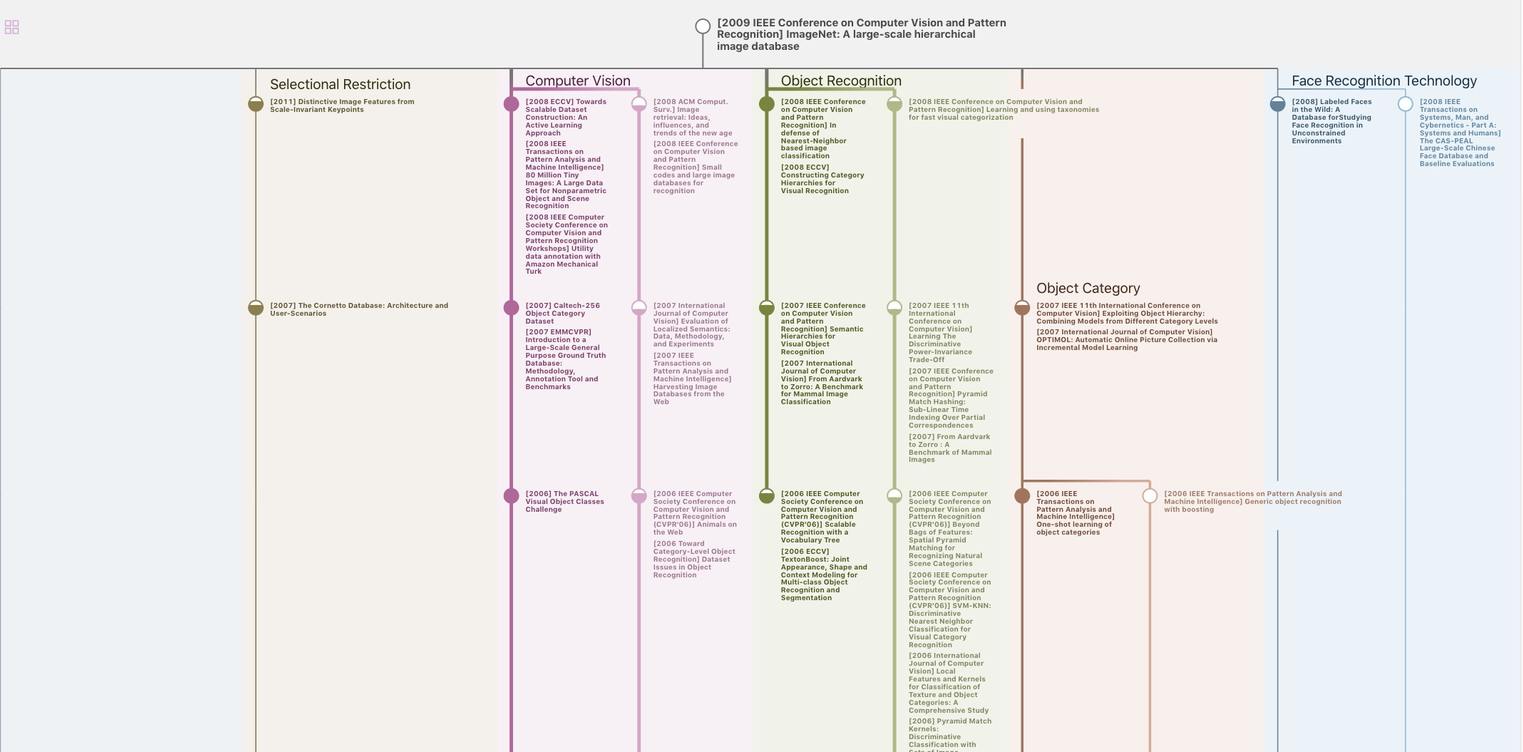
生成溯源树,研究论文发展脉络
Chat Paper
正在生成论文摘要