Finding The Optimal Cross-Subject Eeg Data Alignment Method For Analysis And Bci
2018 IEEE INTERNATIONAL CONFERENCE ON SYSTEMS, MAN, AND CYBERNETICS (SMC)(2018)
摘要
"Zero-training" classification, or the ability of a Brain-Computer Interface (BCI) model to perform well on a new subject without obtaining any labeled EEG data from the subject, is an active area of BCI research. We define data alignment as a procedure that reduces subject-dependent variability while preserving aspects of data studied in a particular cross-session analysis or utilized in a zero-training BCI application. We investigated the hypothesis that data alignment methods that reduce subject-dependent variability will simultaneously result in more stable, i.e. less variable across recordings, EEG features and better BCI classifiers. Exploring different families of linear transformations of EEG sensor data, derived from statistical measures computed on five minutes of unlabeled data at the start of test sessions, we investigated whether they produced less variable event-related potentials (ERPs), and whether they increased the zero-training performance of a state-of-the-art deep learning based BCI applied to the task of classifying target versus non-target visual stimuli from EEG data. Our results indicate that zero-phase component analysis (ZCA) sphering-based data alignment methods consistently outperform baseline (no data alignment) and purely channel amplitude-based data alignment methods. We also observed statistically significant improvements in BCI performance on visual target detection tasks after sphering-based data alignment.
更多查看译文
关键词
EEG, ERP, Deep Learning, BCI, Sphering, RSVP, Rapid Serial Visual Presentation, EEGNET
AI 理解论文
溯源树
样例
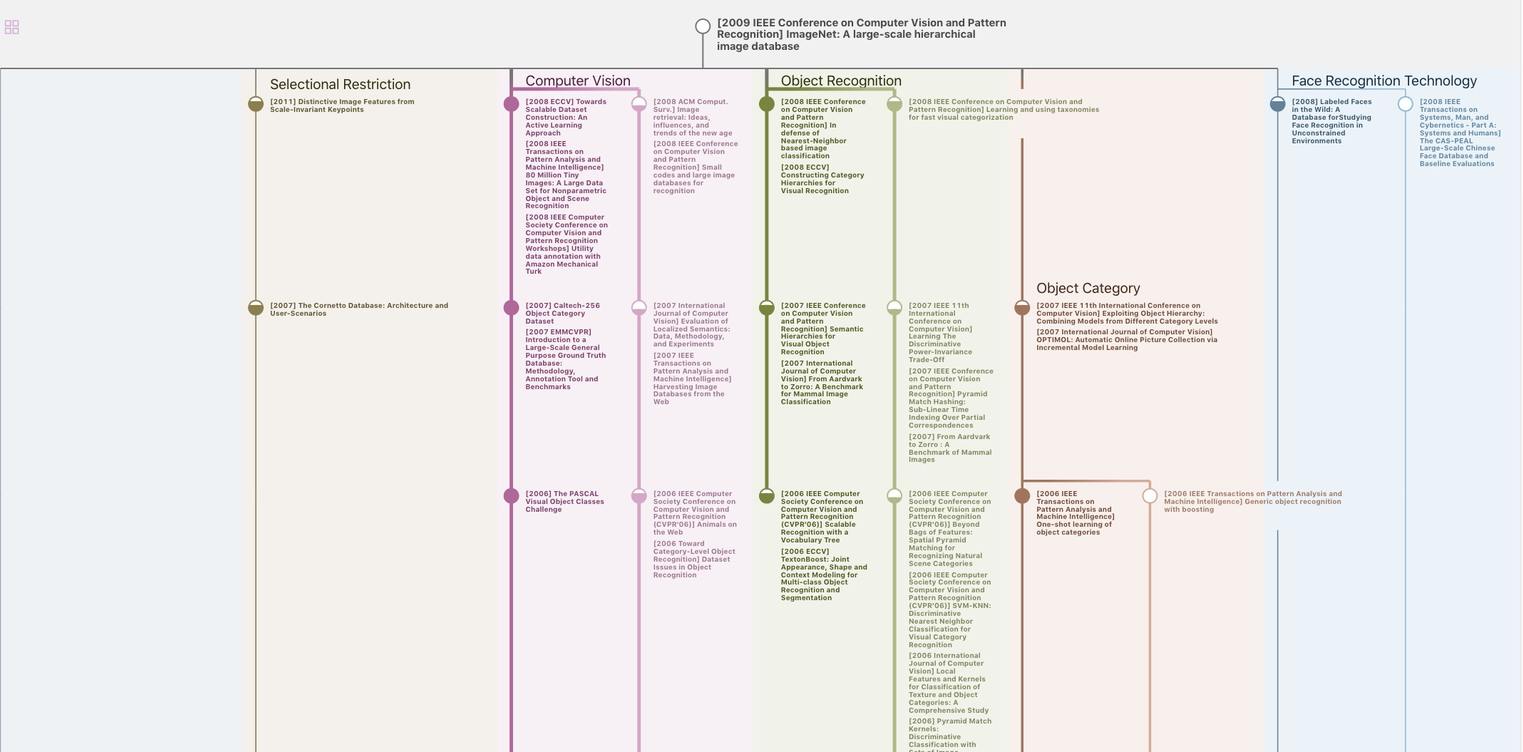
生成溯源树,研究论文发展脉络
Chat Paper
正在生成论文摘要