DrawInAir: A Lightweight Gestural Interface Based on Fingertip Regression
COMPUTER VISION - ECCV 2018 WORKSHOPS, PT VI(2019)
摘要
Hand gestures form a natural way of interaction on Head-Mounted Devices (HMDs) and smartphones. HMDs such as the Microsoft HoloLens and ARCore/ARKit platform enabled smartphones are expensive and are equipped with powerful processors and sensors such as multiple cameras, depth and IR sensors to process hand gestures. To enable mass market reach via inexpensive Augmented Reality (AR) headsets without built-in depth or IR sensors, we propose a real-time, in-air gestural framework that works on monocular RGB input, termed, DrawInAir. DrawInAir uses fingertip for writing in air analogous to a pen on paper. The major challenge in training egocentric gesture recognition models is in obtaining sufficient labeled data for end-to-end learning. Thus, we design a cascade of networks, consisting of a CNN with differentiable spatial to numerical transform (DSNT) layer, for fingertip regression, followed by a Bidirectional Long Short-Term Memory (Bi-LSTM), for a real-time pointing hand gesture classification. We highlight how a model, that is separately trained to regress fingertip in conjunction with a classifier trained on limited classification data, would perform better over end-to-end models. We also propose a dataset of 10 egocentric pointing gestures designed for AR applications for testing our model. We show that the framework takes 1.73 s to run end-to-end and has a low memory footprint of 14MB while achieving an accuracy of 88.0% on egocentric video dataset.
更多查看译文
关键词
Egocentric gestures,Coordinate regression,Augmented reality
AI 理解论文
溯源树
样例
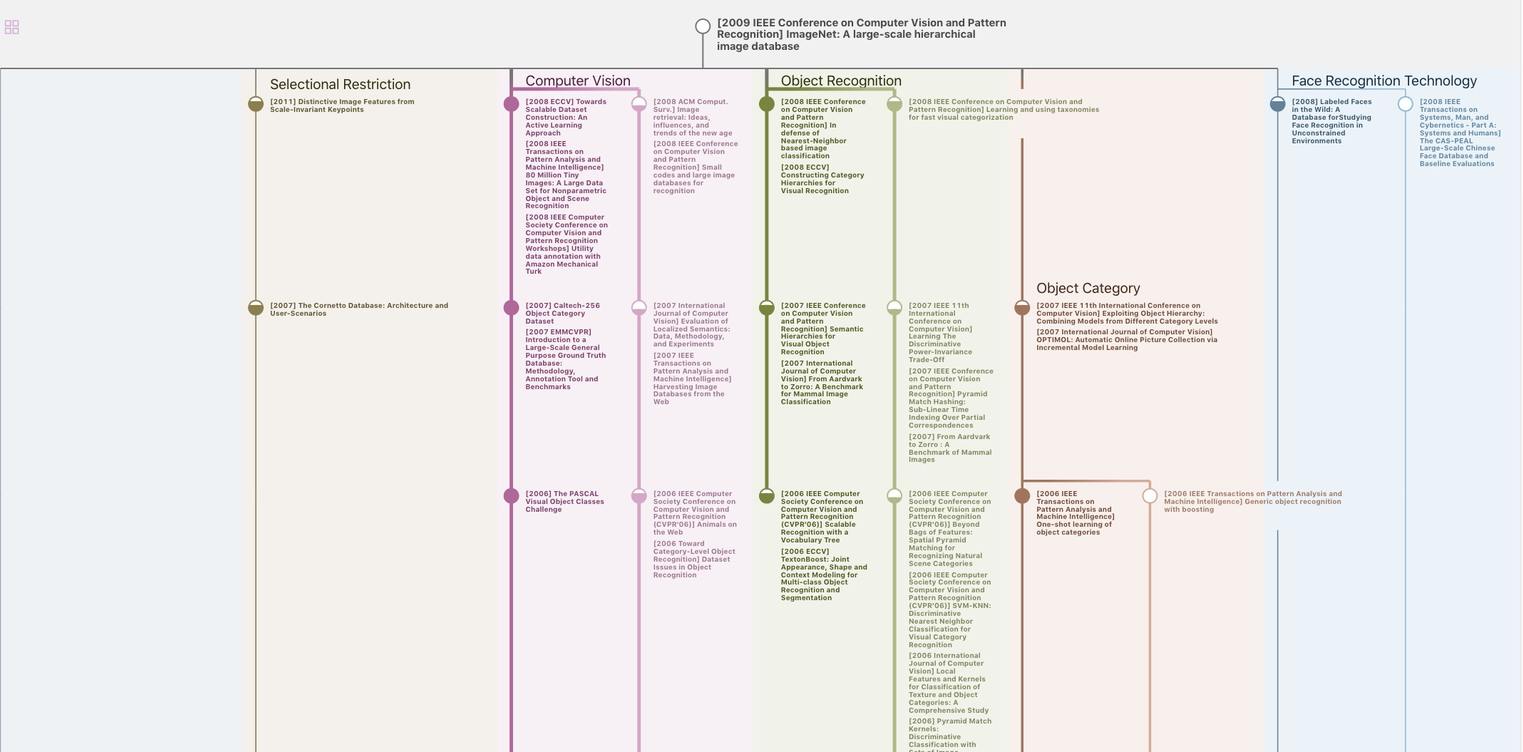
生成溯源树,研究论文发展脉络
Chat Paper
正在生成论文摘要