Alpha Model Domination in Multiple Choice Learning
2018 17TH IEEE INTERNATIONAL CONFERENCE ON MACHINE LEARNING AND APPLICATIONS (ICMLA)(2018)
摘要
Multiple Choice Learning (MCL) algorithms involve an 'oracle' user with unmodeled biases that selects a preferred output among several possible hypotheses. We identify a shortcoming and training obstacle of the recent Stochastic Multiple Choice Learning (sMCL) algorithm. When an ensemble of neural networks trains under sMCL, the best performing model receives the majority of parameter updates at the expense of other ensemble members. We refer to this sMCL training issue as Alpha Model Domination (AMD) and empirically demonstrate that AMD does not resolve itself with longer training time. We introduce several novel MCL loss functions that both avoid AMD and yield statistically significant improvements in oracle accuracy (OA) compared to sMCL. Using the MNIST, CIFAR-10, and ImageNet classification datasets, we empirically demonstrate the superior performance of our proposed loss functions.
更多查看译文
关键词
Multiple Choice Learning, ensembles, neural networks
AI 理解论文
溯源树
样例
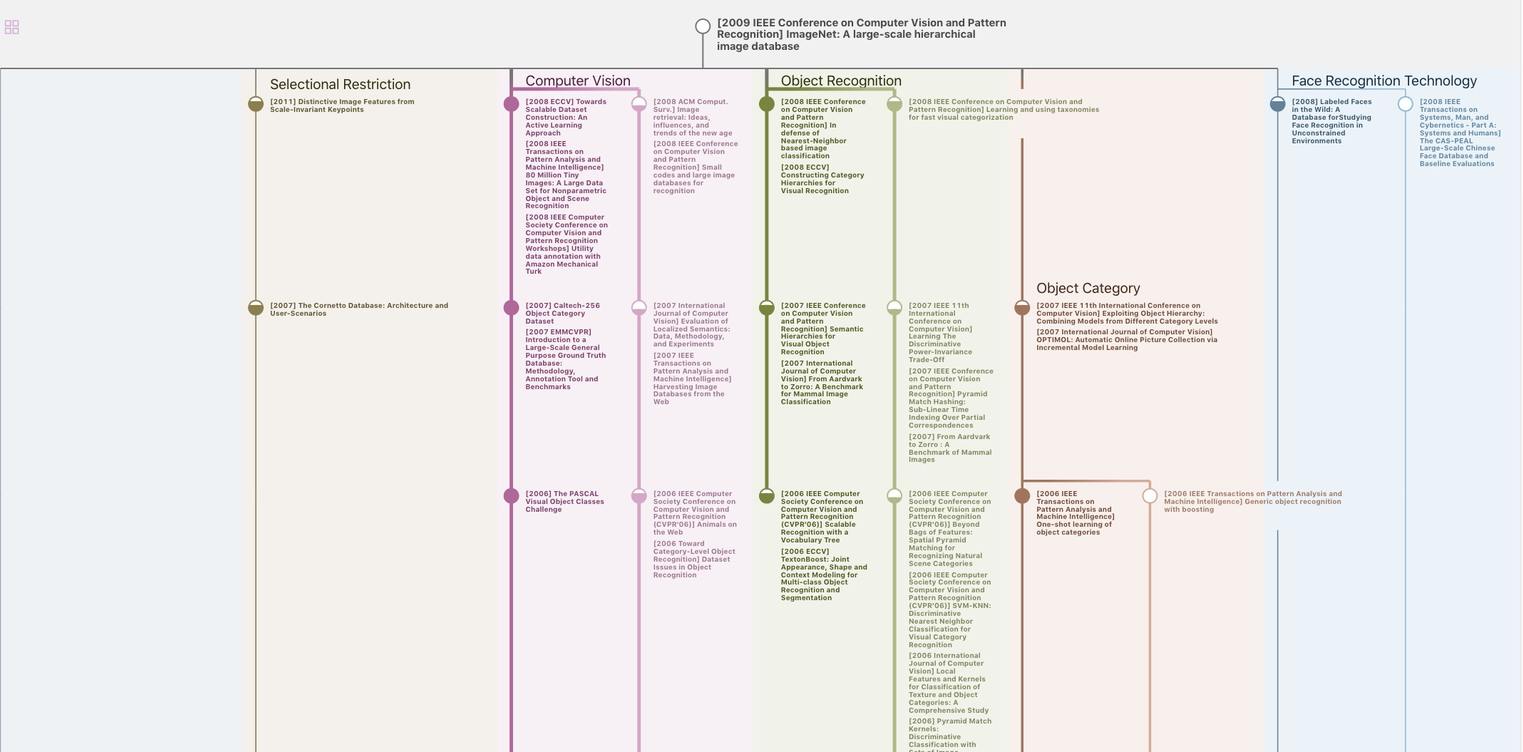
生成溯源树,研究论文发展脉络
Chat Paper
正在生成论文摘要