Bio-inspired Analysis of Deep Learning on Not-So-Big Data Using Data-Prototypes.
FRONTIERS IN COMPUTATIONAL NEUROSCIENCE(2019)
摘要
Deep artificial neural networks are feed-forward architectures capable of very impressive performances in diverse domains. Indeed stacking multiple layers allows a hierarchical composition of local functions, providing efficient compact mappings. Compared to the brain, however, such architectures are closer to a single pipeline and require huge amounts of data, while concrete cases for either human or machine learning systems are often restricted to not-so-big data sets. Furthermore, interpretability of the obtained results is a key issue: since deep learning applications are increasingly present in society, it is important that the underlying processes be accessible and understandable to every one. In order to target these challenges, in this contribution we analyze how considering prototypes in a rather generalized sense (with respect to the state of the art) allows to reasonably work with small data sets while providing an interpretable view of the obtained results. Some mathematical interpretation of this proposal is discussed. Sensitivity to hyperparameters is a key issue for reproducible deep learning results, and is carefully considered in our methodology. Performances and limitations of the proposed setup are explored in details, under different hyperparameter sets, in an analogous way as biological experiments are conducted. We obtain a rather simple architecture, easy to explain, and which allows, combined with a standard method, to target both performances and interpretability.
更多查看译文
关键词
deep learning,not so big data,data prototypes,interpretability,transfer learning
AI 理解论文
溯源树
样例
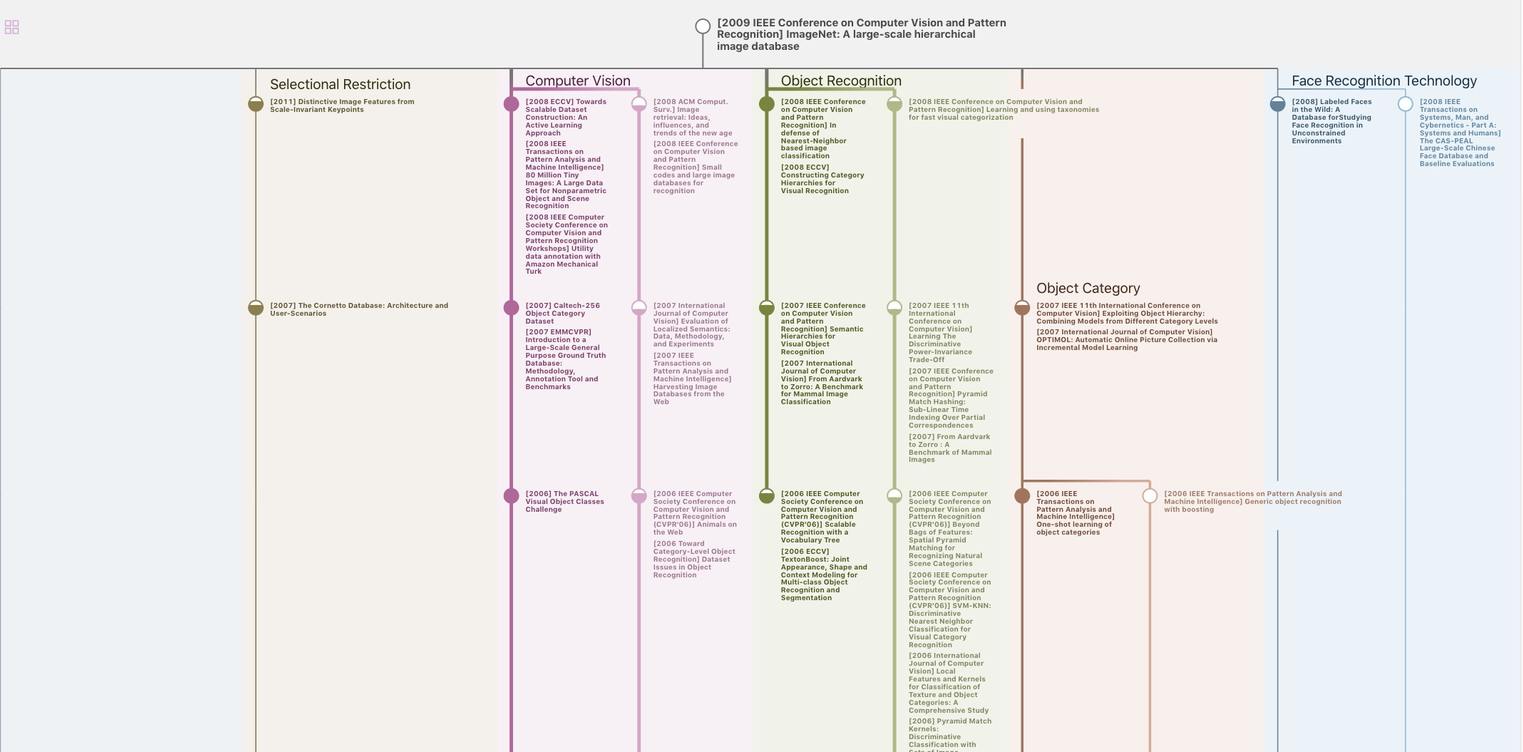
生成溯源树,研究论文发展脉络
Chat Paper
正在生成论文摘要