A Method to Build Multi-Scene Datasets for CNN for Camera Pose Regression
2018 IEEE International Conference on Artificial Intelligence and Virtual Reality (AIVR)(2018)
摘要
Convolutional neural networks (CNN) have shown to be useful for camera pose regression, and They have robust effects against some challenging scenarios such as lighting changes, motion blur, and scenes with lots of textureless surfaces. Additionally, PoseNet shows that the deep learning system can interpolate the camera pose in space between training images. In this paper, we explore how different strategies for processing datasets will affect the pose regression and propose a method for building multi-scene datasets for training such neural networks. We demonstrate that the location of several scenes can be remembered using only one neural network. By combining multiple scenes, we found that the position errors of the neural network do not decrease significantly as the distance between the cameras increases, which means that we do not need to train several models for the increase number of scenes. We also explore the impact factors that influence the accuracy of models for multi-scene camera pose regression, which can help us merge several scenes into one dataset in a better way. We opened our code and datasets to the public for better researches.
更多查看译文
关键词
visual localization,camera pose estimation,convolutional neural network,dataset
AI 理解论文
溯源树
样例
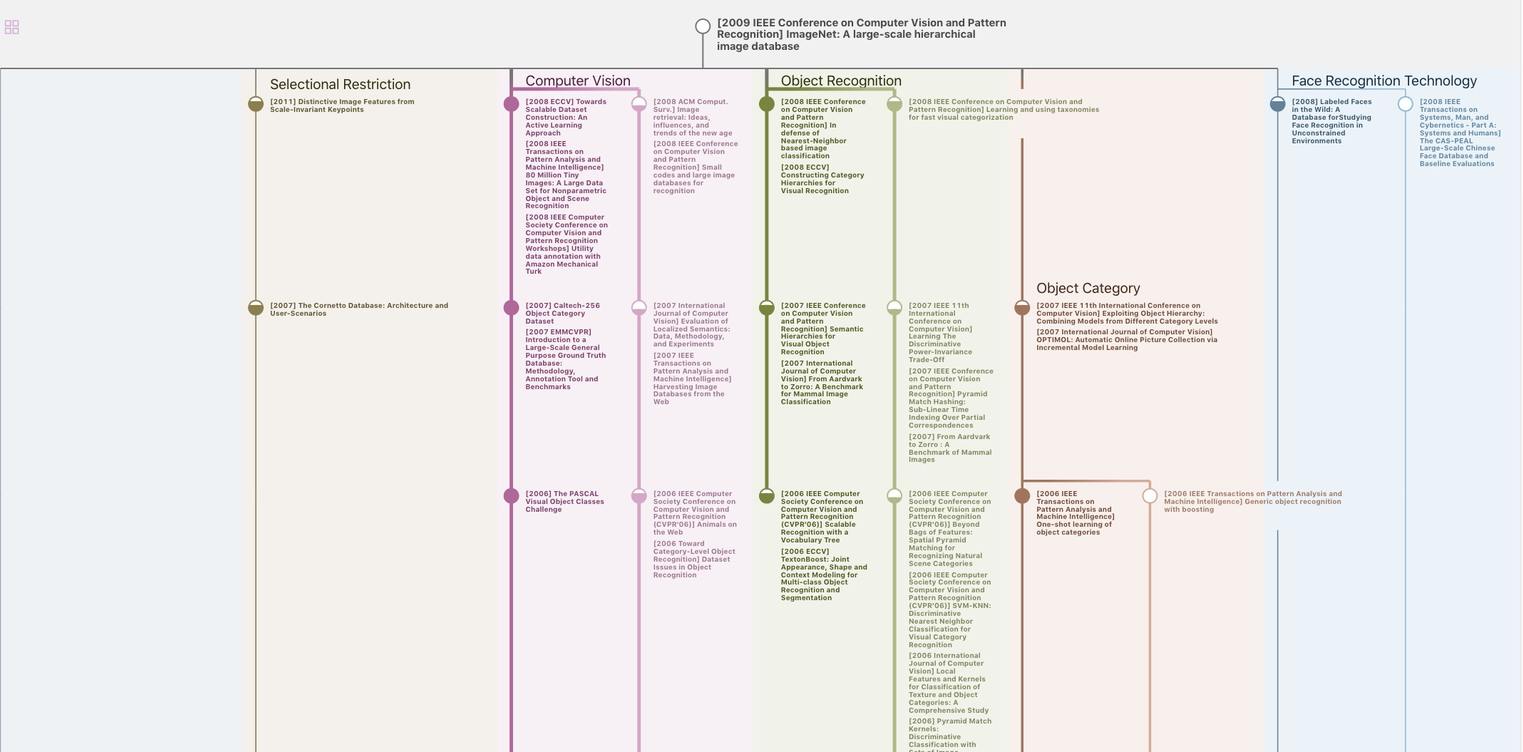
生成溯源树,研究论文发展脉络
Chat Paper
正在生成论文摘要