Residual Stacked Rnns For Action Recognition
COMPUTER VISION - ECCV 2018 WORKSHOPS, PT II(2018)
摘要
Action recognition pipelines that use Recurrent Neural Networks (RNN) are currently 5-10% less accurate than Convolutional Neural Networks (CNN). While most works that use RNNs employ a 2D CNN on each frame to extract descriptors for action recognition, we extract spatiotemporal features from a 3D CNN and then learn the temporal relationship of these descriptors through a stacked residual recurrent neural network (Res-RNN). We introduce for the first time residual learning to counter the degradation problem in multi-layer RNNs, which have been successful for temporal aggregation in two-stream action recognition pipelines. Finally, we use a late fusion strategy to combine RGB and optical flow data of the two-stream Res-RNN. Experimental results show that the proposed pipeline achieves competitive results on UCF-101 and state of-the-art results for RNN-like architectures on the challenging HMDB-51 dataset.
更多查看译文
关键词
Action recognition, Deep residual learning, Two-stream RNN
AI 理解论文
溯源树
样例
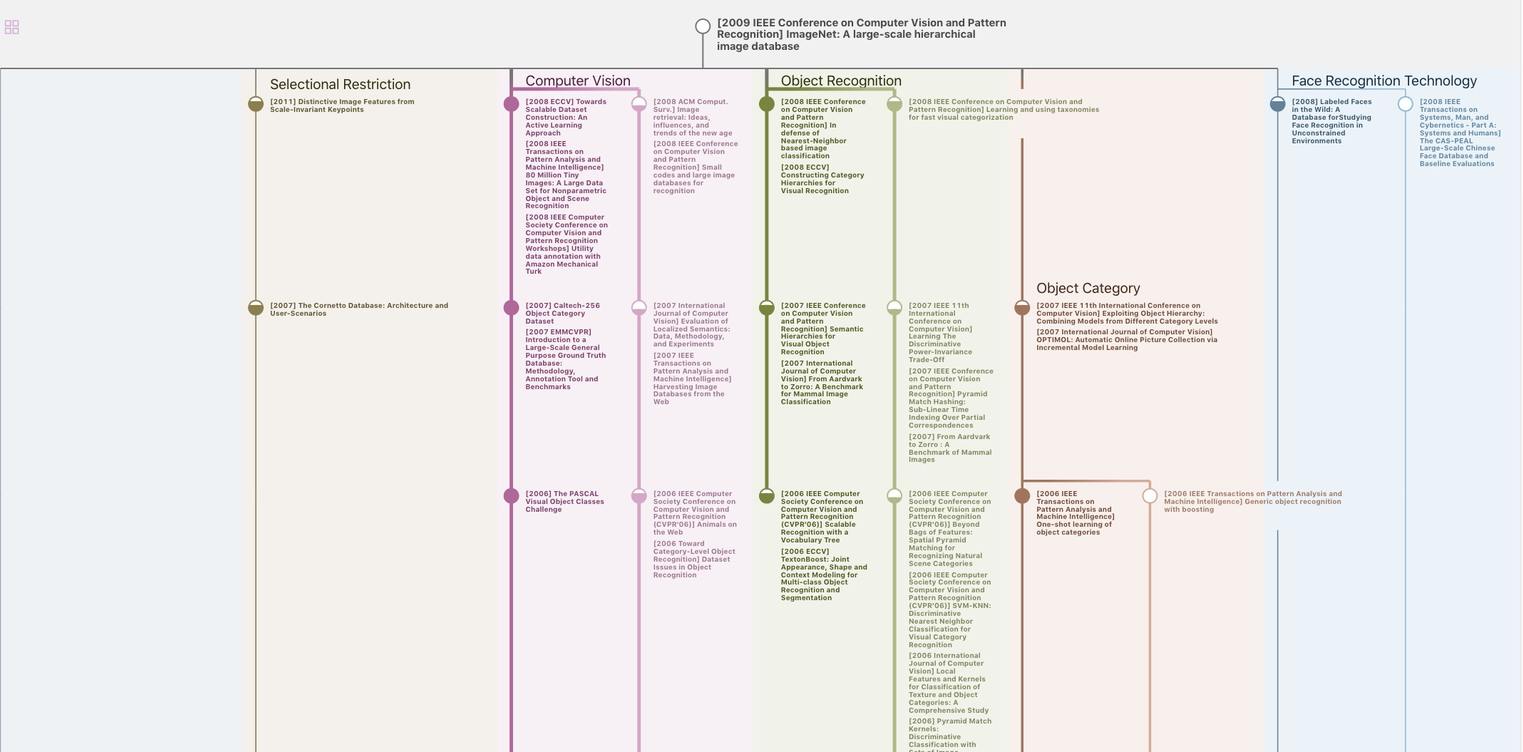
生成溯源树,研究论文发展脉络
Chat Paper
正在生成论文摘要