Determining the Optimal Window Parameters for Accurate and Reliable Decoding of Multiple Classes of Upper Limb Motor Imagery Tasks
2018 IEEE International Conference on Cyborg and Bionic Systems (CBS)(2018)
摘要
Individuals with high-level amputation or neuromuscular disorder basically lack sufficient residual arm muscles from which adequate myoelectric signals could be obtained for accurate decoding of their limb movement intents for prosthesis control. In this regard, electroencephalogram (EEG) signal associated with their limb movements could be used to provide alternative control input to multiple degrees of freedom prosthesis for such individuals. The feature extraction method and analysis window parameters (window size and window increment) used to characterize the EEG signal patterns plays an important role in determining the accuracy and stability of the entire prosthetics. Therefore, we studied the effect of different analysis windowing parameters on classification accuracy and stability of frequency-domain features using 64-channel EEG recordings obtained from three amputees. Experimental results shows that the motor imagery patterns associated with the five classes of upper limb movement were decoded with an average accuracy of 99.79% when a window length of 100ms and increment of 25ms was utilized. Regardless of the window increment used, the classification accuracy of the decoded limb movements increased steadily with a decrease in the window length, indicating an inverse relationship between accuracy and window length. Thus, the outcome of this study may provide valuable information for the development of accurate and robust control mechanism for multifunctional neuroprosthesis.
更多查看译文
关键词
optimal window parameters,reliable decoding,multiple classes,upper limb motor imagery tasks,high-level amputation,neuromuscular disorder,sufficient residual arm muscles,adequate myoelectric signals,accurate decoding,prosthesis control,electroencephalogram signal,alternative control input,feature extraction method,window size,window increment,EEG signal patterns,stability,classification accuracy,frequency-domain features,motor imagery patterns,upper limb movement,window length,decoded limb movements,robust control mechanism,multiple degrees-of-freedom prosthesis,64-channel EEG recordings,multifunctional neuroprosthesis,time 100.0 ms,time 25.0 ms
AI 理解论文
溯源树
样例
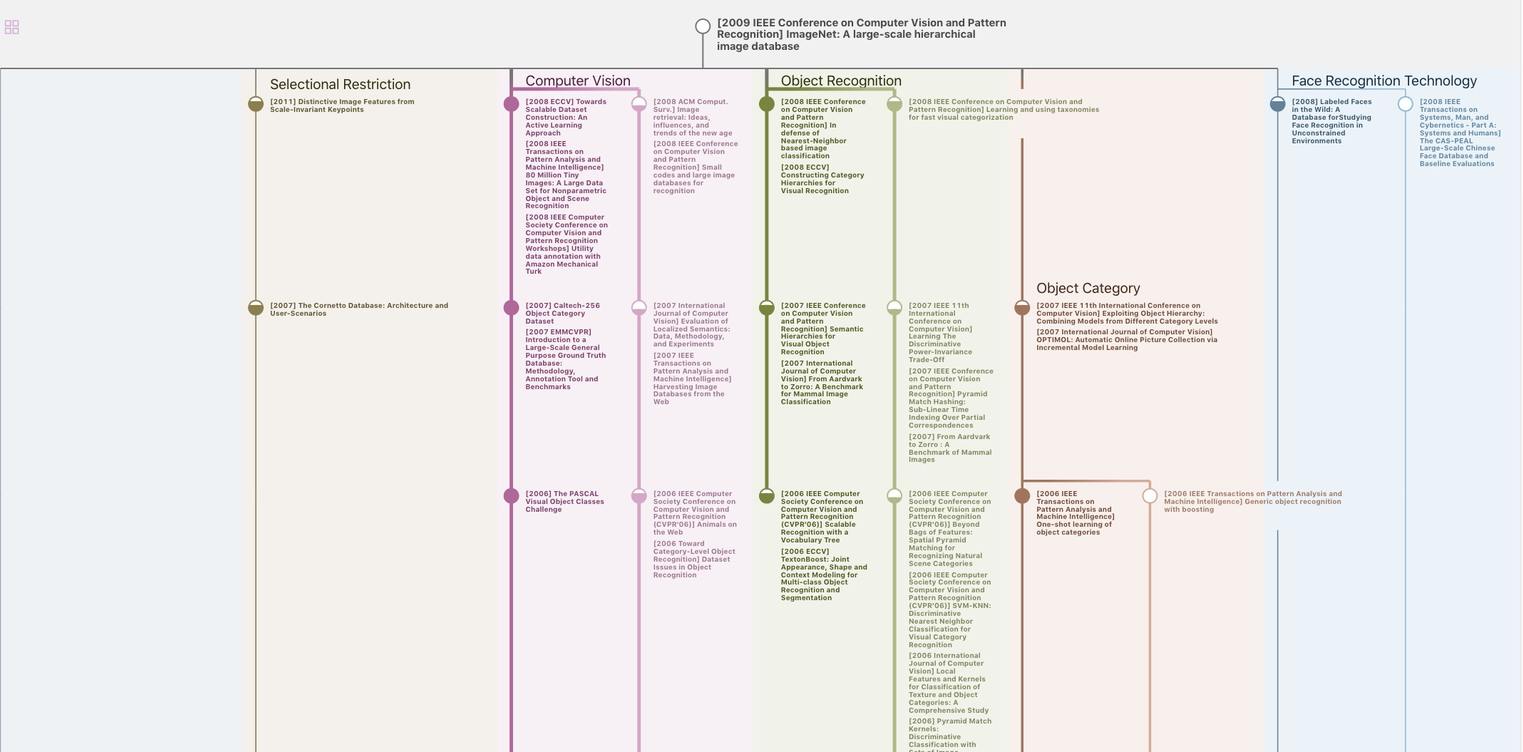
生成溯源树,研究论文发展脉络
Chat Paper
正在生成论文摘要