DenseNet model combined with Haralick’s handcrafted features for texture classification
2018 IEEE Latin American Conference on Computational Intelligence (LA-CCI)(2018)
摘要
Texture is an important characteristic of many types of objects, and texture representation is an important part of computer vision challenges. Several solutions to features representation have been proposed such as handcrafted features, Bag of Words (BoW) and Convolutional Neural Networks (CNN). Different CNN architectures have achieved outstanding performance in several fields of computer vision, however, when appearing textures, the problem is far from being solved. A recent research has shown that texture databases have a high dimensionality manifold and that the traditional CNN are not able to represent all the necessary information to achieve a very good classification. In this paper, we show that combining DenseNet model with Haralick's handcrafted in intermediate layers is a way to overcome this problem. The solution was validated with two datasets DTD and KTH-tips2-b which have 47 and 11 classes respectively. It was achieved a near performance to the state of the art in the first database obtaining an accuracy of 70.5% and overcoming the state of art in the second one, obtaining an accuracy of 84.42% respect to 82.6%.
更多查看译文
关键词
Texture representation,Convolutional Neural Network,DenseNet,handcrafted features
AI 理解论文
溯源树
样例
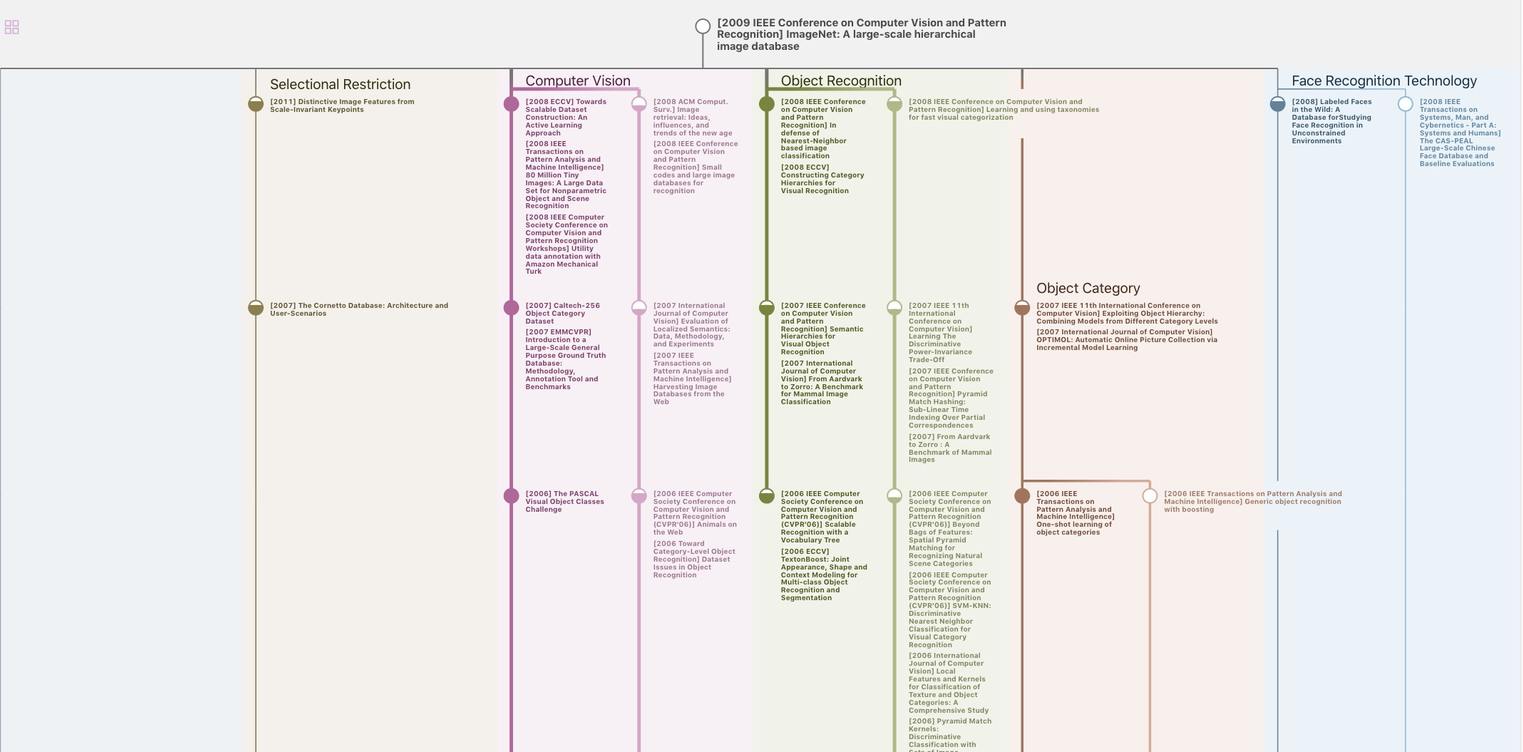
生成溯源树,研究论文发展脉络
Chat Paper
正在生成论文摘要