Self-Optimization Strategy For Io Accelerator Parameterization
HIGH PERFORMANCE COMPUTING, ISC HIGH PERFORMANCE 2018(2018)
摘要
Exascale reaching imposes a high automation level on HPC supercomputers. In this paper, a self-optimization strategy is proposed to improve application IO performance using statistical and machine learning based methods.The proposed method takes advantage of collected IO data through an off-line analysis to infers the most relevant parameterization of an IO accelerator that should be used for the next launch of a similar job. This is thus a continuous improvement process that will converge toward an optimal parameterization along iterations.The inference process uses a numerical optimization method to propose the parameterization that minimizes the execution time of the considered application. A regression method is used to model the objective function to be optimized from a sparse set of collected data from the past runs.Experiments on different artificial parametric spaces show that the convergence speed of the proposed method requires less than 20 runs to converge toward a parameterization of the IO accelerator.
更多查看译文
关键词
HPC, Supercomputing, IO, Optimization, Regression, Inference, Machine learning, Auto-tuning, Parameterization, Data management
AI 理解论文
溯源树
样例
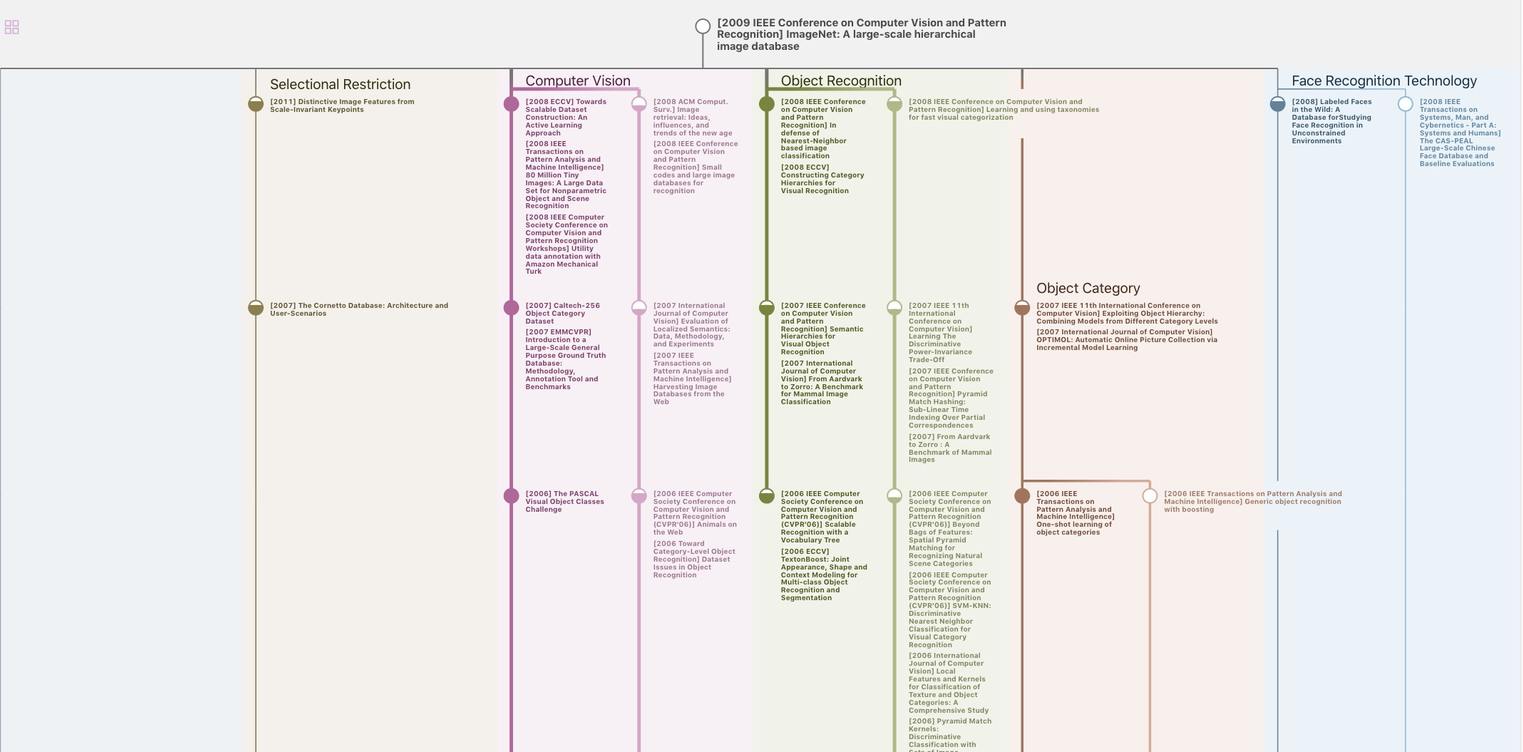
生成溯源树,研究论文发展脉络
Chat Paper
正在生成论文摘要