Regression Models And Ranking Method For P53 Inhibitor Candidates Using Machine Learning
PROCEEDINGS 2018 IEEE INTERNATIONAL CONFERENCE ON BIOINFORMATICS AND BIOMEDICINE (BIBM)(2018)
摘要
Radiation therapy is one of the main treatments for cancer. However, it may cause various side effects owing to the apoptosis activity of the p53 protein in normal cells. Therefore, to avoid the side effects, it is important to protect normal cells against radiation by using p53 inhibitors. It is also expected that p53 inhibitors have low toxicity against patients' bodies. However, the design of p53 inhibitors is not easy because drug discovery requires enormous costs and long time. In this paper, we propose a new method for ranking candidate p53 inhibitors, considering both their radioprotective function and cytotoxicity. We use features of the two-and three-dimensional structures of the compounds, including fingerprints, some machine learning methods such as random forest and SVR (Support Vector Machine), and one method for ranking, i.e., the Pareto ranking method. Therefore, we present the regression models of the cytotoxicity and radioprotective functions of the candidates to determine their ranking. Our proposed methods yield useful rankings for drug discovery.
更多查看译文
关键词
SVR, p53, Pareto ranking, machine learning
AI 理解论文
溯源树
样例
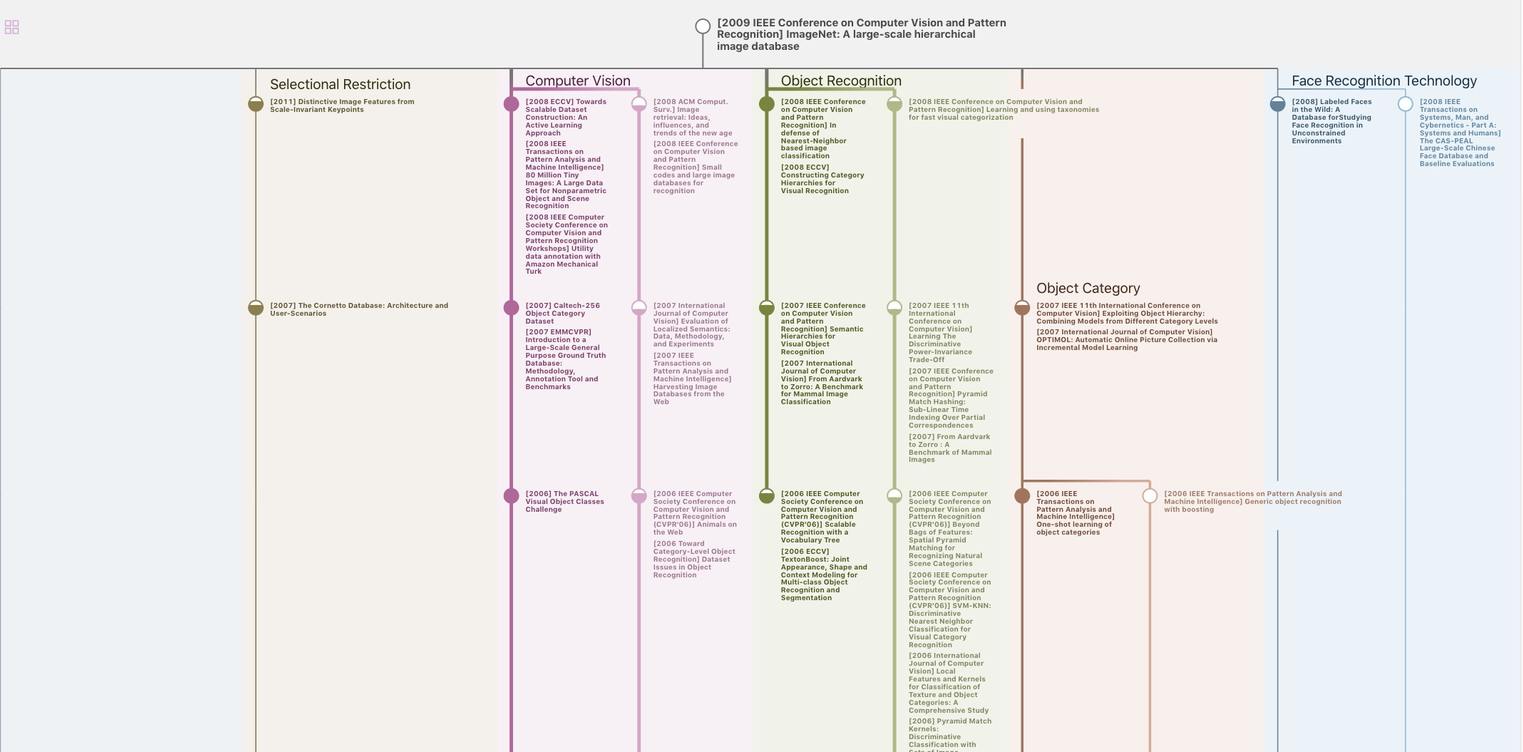
生成溯源树,研究论文发展脉络
Chat Paper
正在生成论文摘要