Pedestrian Detection based on Reduced High-Dimensional Distinctive Feature using Deep Neural Network
Proceedings of the 2018 International Conference on Sensors, Signal and Image Processing(2018)
摘要
Pedestrian detection is an essential and significant research topic due to its diverse applications in the area of safety systems. The distinctiveness is detected by the following three steps. Firstly, images are represented by their respective co-occurrence matrices, which are vectorized by using the bag of visual words (BoVW) framework. Secondly, their weights are calculated from the histograms of visual words of each class. Finally, computed weights are applied to the testing image set as the distinctiveness of visual words. The fully connected Multi-Layer Perceptron (MLP) is used as classification method in our system, which has limited performance. This paper introduces a combination of principle component analysis (PCA), guided filtering, deeplearning architecture into visual data classification. In detail, as a mature dimension reduction architecture, PCA is capable of reducing the redundancy of multi-dimensional information. The proposed method is compared with the MLP using the Caltech 256 datasets, with the following classes: pedestrians, cars, motorbikes and airplanes. The experimental results show that the proposed method outperforms current methods in predicting pedestrians and transportation objects. It yields an average accuracy of 96.50%, which is approximately 8.5% more than the compared state-of-the-art.
更多查看译文
关键词
Bag of visual words, Multi-Layer Perceptron, Pedestrian detection, Principle Component Analysis, Scale-invariant feature transform, Weighting scheme
AI 理解论文
溯源树
样例
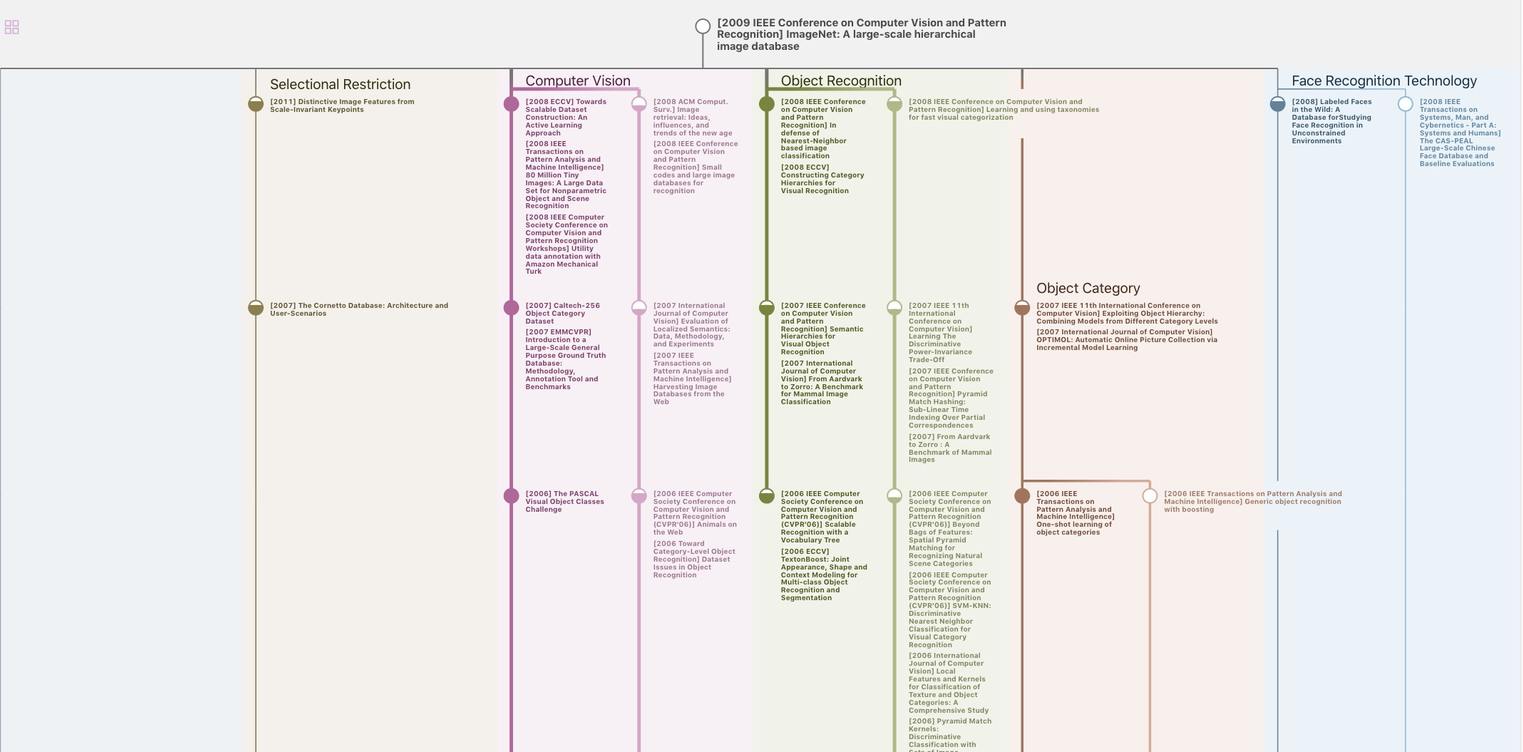
生成溯源树,研究论文发展脉络
Chat Paper
正在生成论文摘要