Gaussian Processes for Unconstraining Demand.
arXiv (Cornell University)(2017)
摘要
One of the key challenges in revenue management is unconstraining demand data. Existing state of the art single-class unconstraining methods make restrictive assumptions about the form of the underlying demand and can perform poorly when applied to data which breaks these assumptions. In this paper, we propose a novel unconstraining method that uses Gaussian process (GP) regression. We develop a novel GP model by constructing and implementing a new non-stationary covariance function for the GP which enables it to learn and extrapolate the underlying demand trend. We show that this method can cope with important features of realistic demand data, including nonlinear demand trends, variations in total demand, lengthy periods of constraining, non-exponential inter-arrival times, and discontinuities/changepoints in demand data. In all such circumstances, our results indicate that GPs outperform existing single-class unconstraining methods.
更多查看译文
关键词
Revenue management,OR in airlines,Demand unconstraining,Gaussian process regression
AI 理解论文
溯源树
样例
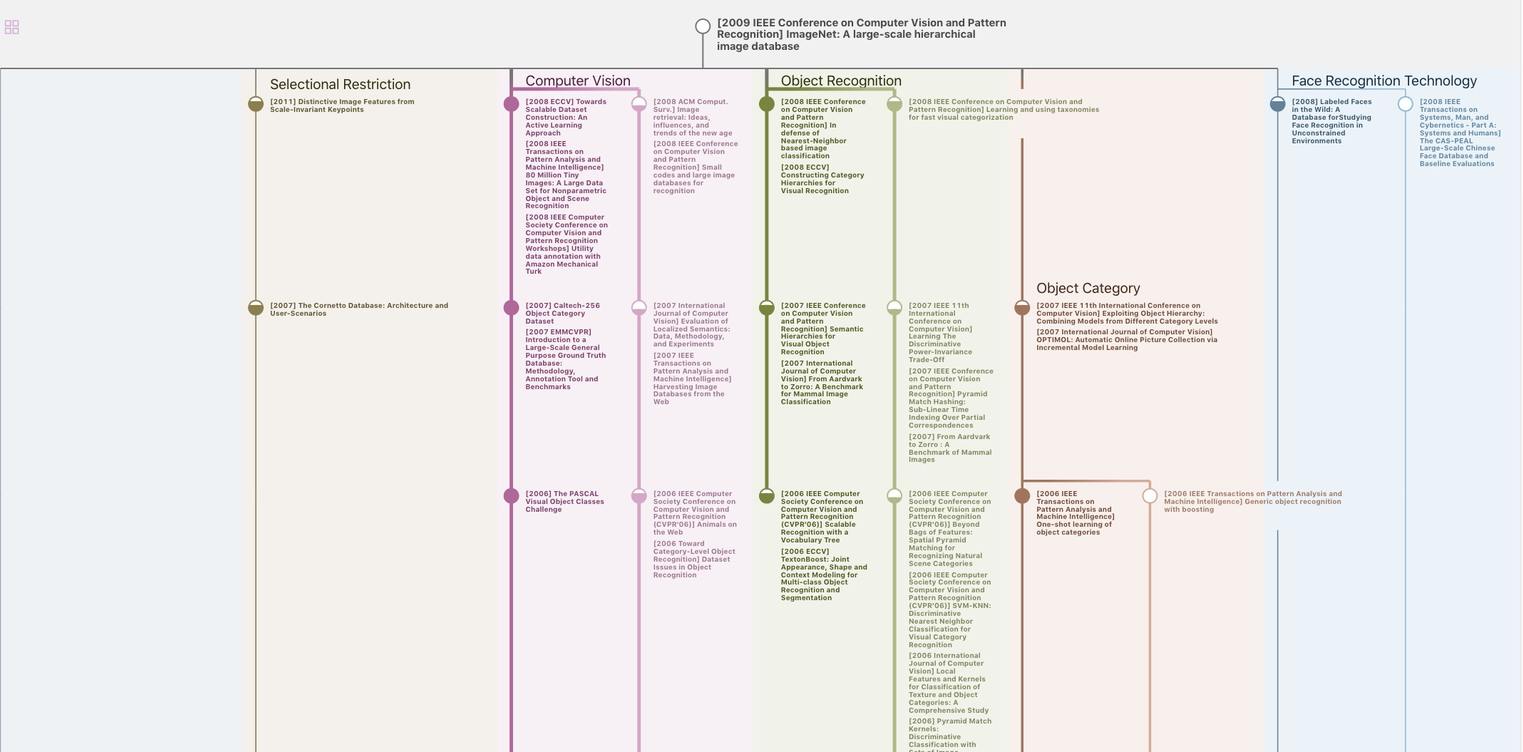
生成溯源树,研究论文发展脉络
Chat Paper
正在生成论文摘要