Shaping Feedback Data in Recommender Systems with Interventions Based on Information Foraging Theory.
WSDM(2019)
摘要
Recommender systems rely heavily on the predictive accuracy of the learning algorithm. Most work on improving accuracy has focused on the learning algorithm itself. We argue that this algorithmic focus is myopic. In particular, since learning algorithms generally improve with more and better data, we propose shaping the feedback generation process as an alternate and complementary route to improving accuracy. To this effect, we explore how changes to the user interface can impact the quality and quantity of feedback data -- and therefore the learning accuracy. Motivated by information foraging theory, we study how feedback quality and quantity are influenced by interface design choices along two axes: information scent and information access cost. We present a user study of these interface factors for the common task of picking a movie to watch, showing that these factors can effectively shape and improve the implicit feedback data that is generated while maintaining the user experience.
更多查看译文
关键词
human-in-the-loop, implicit feedback signals, machine learning, usability
AI 理解论文
溯源树
样例
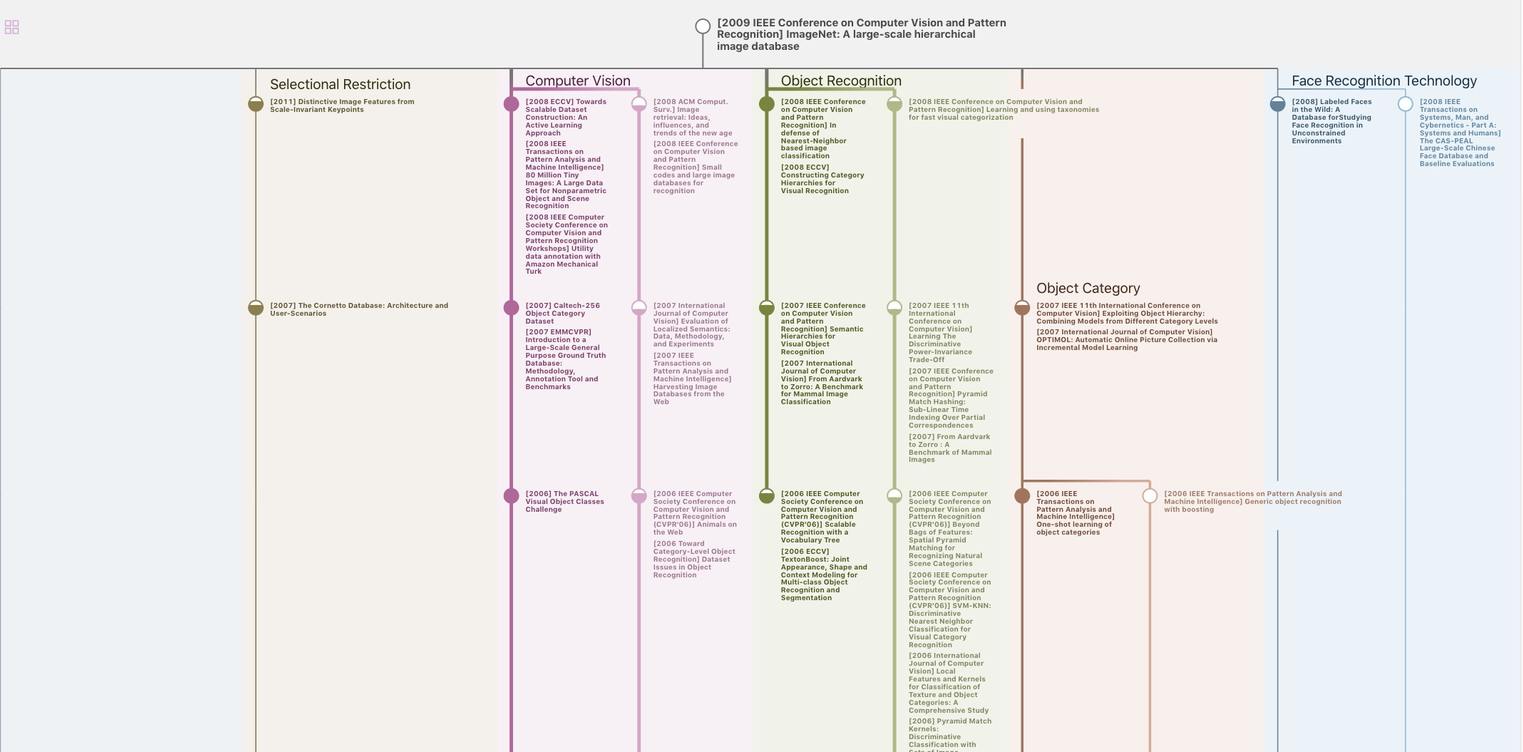
生成溯源树,研究论文发展脉络
Chat Paper
正在生成论文摘要