Task Duration Estimation.
WSDM(2019)
摘要
Estimating how long a task will take to complete (i.e., the task duration) is important for many applications, including calendaring and project management. Population-scale calendar data contains distributional information about time allocated by individuals for tasks that may be useful to build computational models for task duration estimation. This study analyzes anonymized large-scale calendar appointment data from hundreds of thousands of individuals and millions of tasks to understand expected task durations and the longitudinal evolution in these durations. Machine-learned models are trained using the appointment data to estimate task duration. Study findings show that task attributes, including content (anonymized appointment subjects), context, and history, are correlated with time allocated for tasks. We also show that machine-learned models can be trained to estimate task duration, with multiclass classification accuracies of almost 80%. The findings have implications for understanding time estimation in populations, and in the design of support in digital assistants and calendaring applications to find time for tasks and to help people, especially those who are new to a task, block sufficient time for task completion.
更多查看译文
关键词
intelligent scheduling, task duration, time estimation
AI 理解论文
溯源树
样例
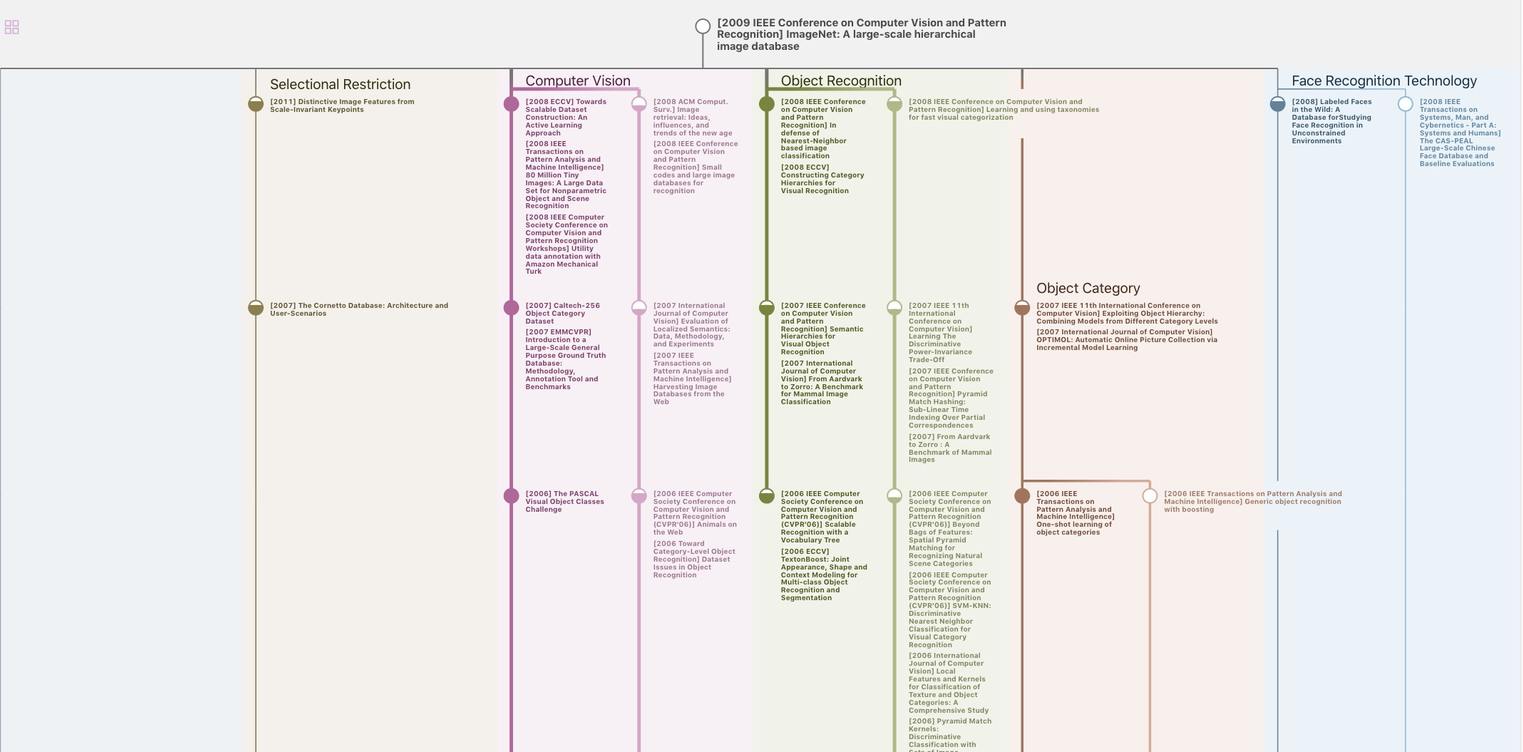
生成溯源树,研究论文发展脉络
Chat Paper
正在生成论文摘要