A Recurrent Neural Network Survival Model: Predicting Web User Return Time
MACHINE LEARNING AND KNOWLEDGE DISCOVERY IN DATABASES, ECML PKDD 2018, PT III(2018)
摘要
The size of a website's active user base directly affects its value. Thus, it is important to monitor and influence a user's likelihood to return to a site. Essential to this is predicting when a user will return. Current state of the art approaches to solve this problem come in two flavors: (1) Recurrent Neural Network (RNN) based solutions and (2) survival analysis methods. We observe that both techniques are severely limited when applied to this problem. Survival models can only incorporate aggregate representations of users instead of automatically learning a representation directly from a raw time series of user actions. RNNs can automatically learn features, but can not be directly trained with examples of non-returning users who have no target value for their return time. We develop a novel RNN survival model that removes the limitations of the state of the art methods. We demonstrate that this model can successfully be applied to return time prediction on a large e-commerce dataset with a superior ability to discriminate between returning and non-returning users than either method applied in isolation.
更多查看译文
关键词
User return time,Web browse sessions,Recurrent neural network,Marked temporal point process,Survival analysis
AI 理解论文
溯源树
样例
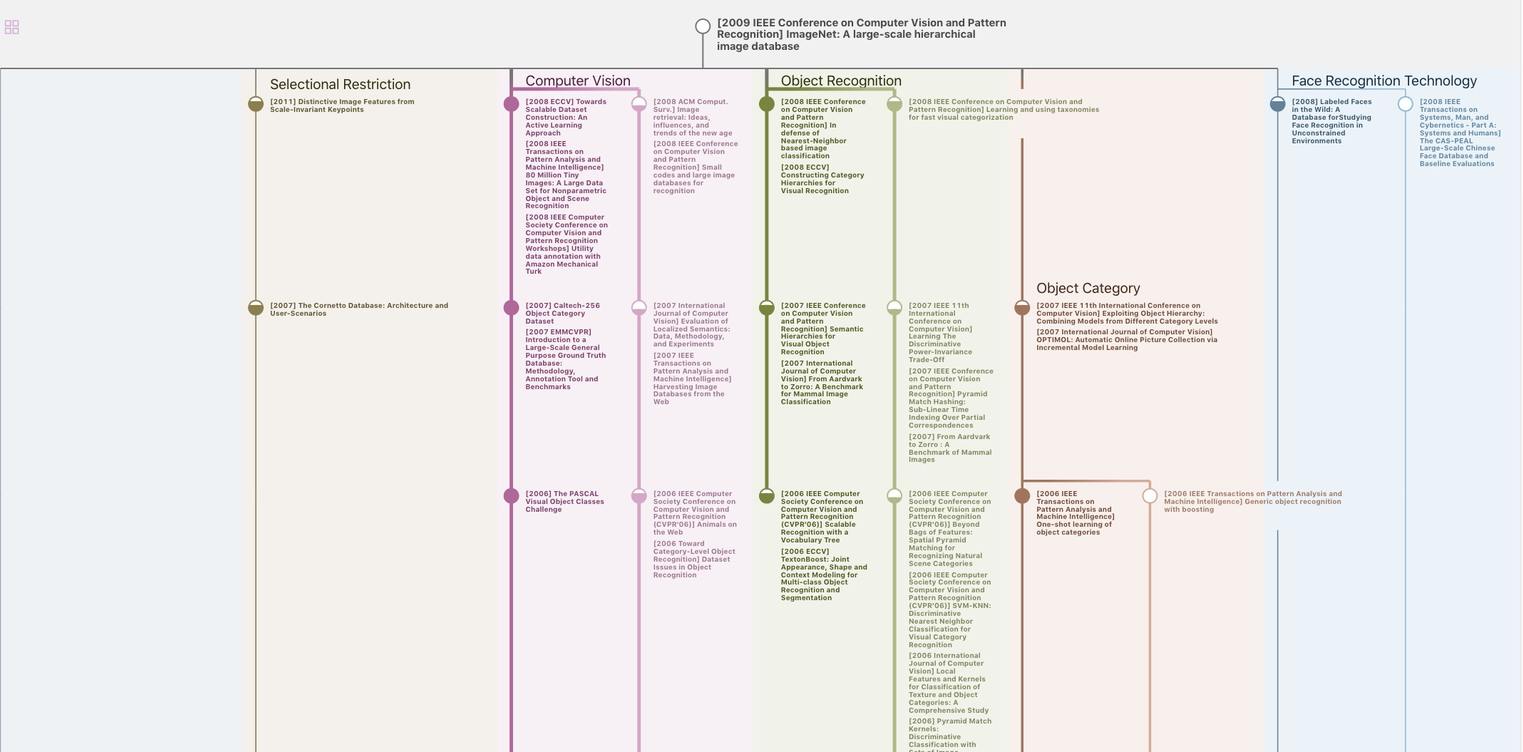
生成溯源树,研究论文发展脉络
Chat Paper
正在生成论文摘要