Syntax-Directed Hybrid Attention Network for Aspect-Level Sentiment Analysis
IEEE Access(2018)SCI 3区SCI 4区
Chinese Acad Sci
Abstract
Aspect-level sentiment analysis is a fine-grained task in sentiment analysis that aims at detecting sentiment polarity towards a specific target in a sentence. Previous studies focus on using global attention mechanism that attends to all words in the context to model the interaction between target and sentence. However, global attention suffers from assigning high-attention score to irrelevant sentiment words in the cases where sentence contains noisy words or multiple targets. To address this problem, we propose a novel syntax-directed hybrid attention network (SHAN). In SHAN, a global attention is employed to capture coarse information about the target, and a syntax-directed local attention is used to take a look at words syntactically close to the target. An information gate is then utilized to synthesize the information from local and global attention results and adaptively generate a less-noisy and more sentiment-oriented representation. The experimental results on SemEval 2014 Datasets demonstrate the effectiveness of the proposed method.
MoreTranslated text
Key words
Aspect-level sentiment analysis,hybrid attention,syntactic information,gating mechanism
求助PDF
上传PDF
View via Publisher
AI Read Science
AI Summary
AI Summary is the key point extracted automatically understanding the full text of the paper, including the background, methods, results, conclusions, icons and other key content, so that you can get the outline of the paper at a glance.
Example
Background
Key content
Introduction
Methods
Results
Related work
Fund
Key content
- Pretraining has recently greatly promoted the development of natural language processing (NLP)
- We show that M6 outperforms the baselines in multimodal downstream tasks, and the large M6 with 10 parameters can reach a better performance
- We propose a method called M6 that is able to process information of multiple modalities and perform both single-modal and cross-modal understanding and generation
- The model is scaled to large model with 10 billion parameters with sophisticated deployment, and the 10 -parameter M6-large is the largest pretrained model in Chinese
- Experimental results show that our proposed M6 outperforms the baseline in a number of downstream tasks concerning both single modality and multiple modalities We will continue the pretraining of extremely large models by increasing data to explore the limit of its performance
Upload PDF to Generate Summary
Must-Reading Tree
Example
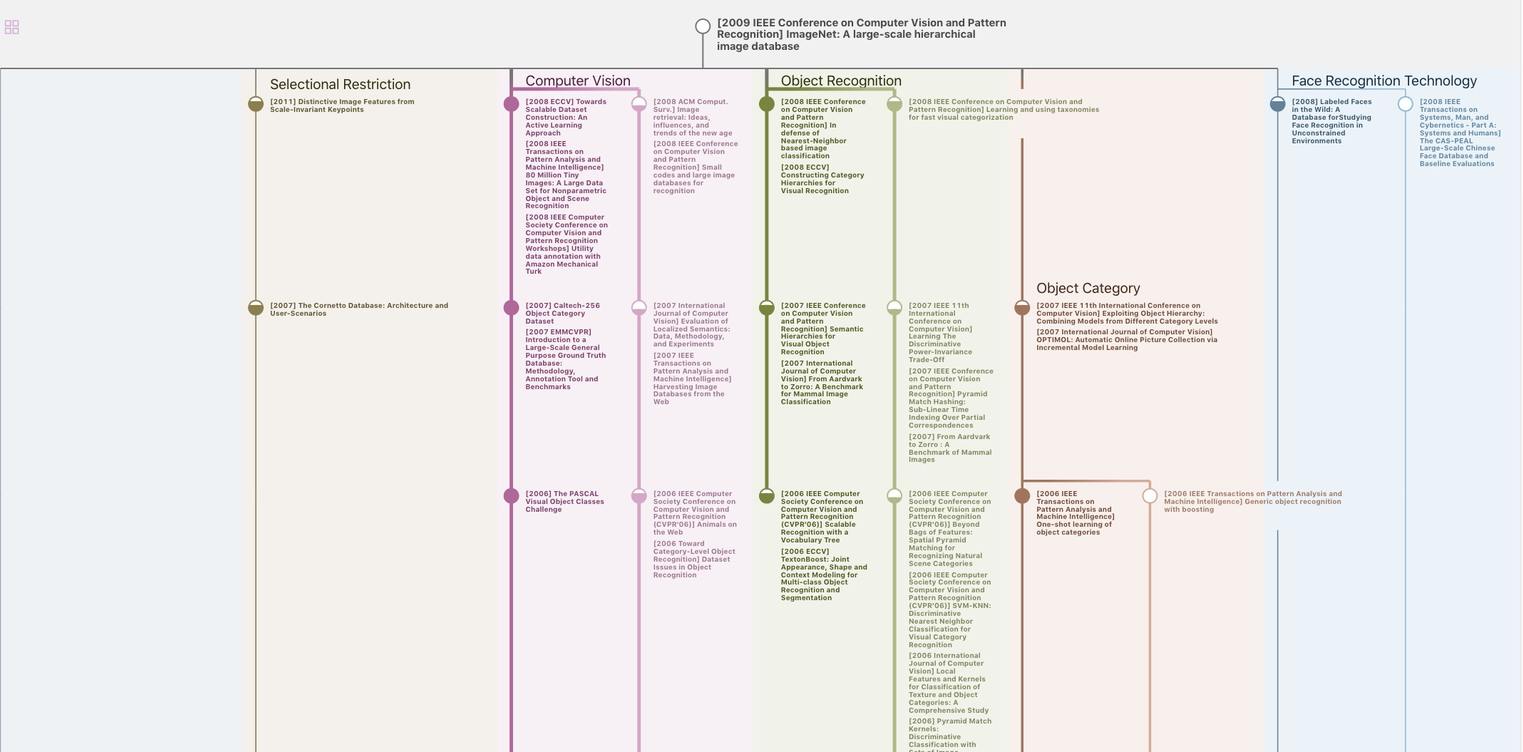
Generate MRT to find the research sequence of this paper
Related Papers
2014
被引用1283 | 浏览
2016
被引用2607 | 浏览
2018
被引用26 | 浏览
2018
被引用358 | 浏览
Data Disclaimer
The page data are from open Internet sources, cooperative publishers and automatic analysis results through AI technology. We do not make any commitments and guarantees for the validity, accuracy, correctness, reliability, completeness and timeliness of the page data. If you have any questions, please contact us by email: report@aminer.cn
Chat Paper