Exact Recovery for a Family of Community-Detection Generative Models
2019 IEEE International Symposium on Information Theory (ISIT)(2019)
摘要
Generative models for networks with communities have been studied extensively for being a fertile ground to establish information-theoretic and computational thresholds. In this paper we propose a new toy model for planted generative models called planted Random Energy Model (REM), inspired by Derrida's REM. For this model we provide the asymptotic behaviour of the probability of error for the maximum likelihood estimator and hence the exact recovery threshold. As an application, we further consider the 2 non-equally sized community Weighted Stochastic Block Model (2-WSBM) on h uniform hypergraphs, that is equivalent to the P-REM on both sides of the spectrum, for high and low edge cardinality h. We provide upper and lower bounds for the exact recoverability for any h, mapping these problems to the aforementioned P-REM. To the best of our knowledge these are the first consistency results for the 2-WSBM on graphs and on hypergraphs with non-equally sized community.
更多查看译文
关键词
planted generative models,maximum likelihood estimator,2-WSBM,community-detection generative models,computational thresholds,random energy model,weighted stochastic block model,information-theoretic threshold,high edge cardinality,low edge cardinality,P-REM
AI 理解论文
溯源树
样例
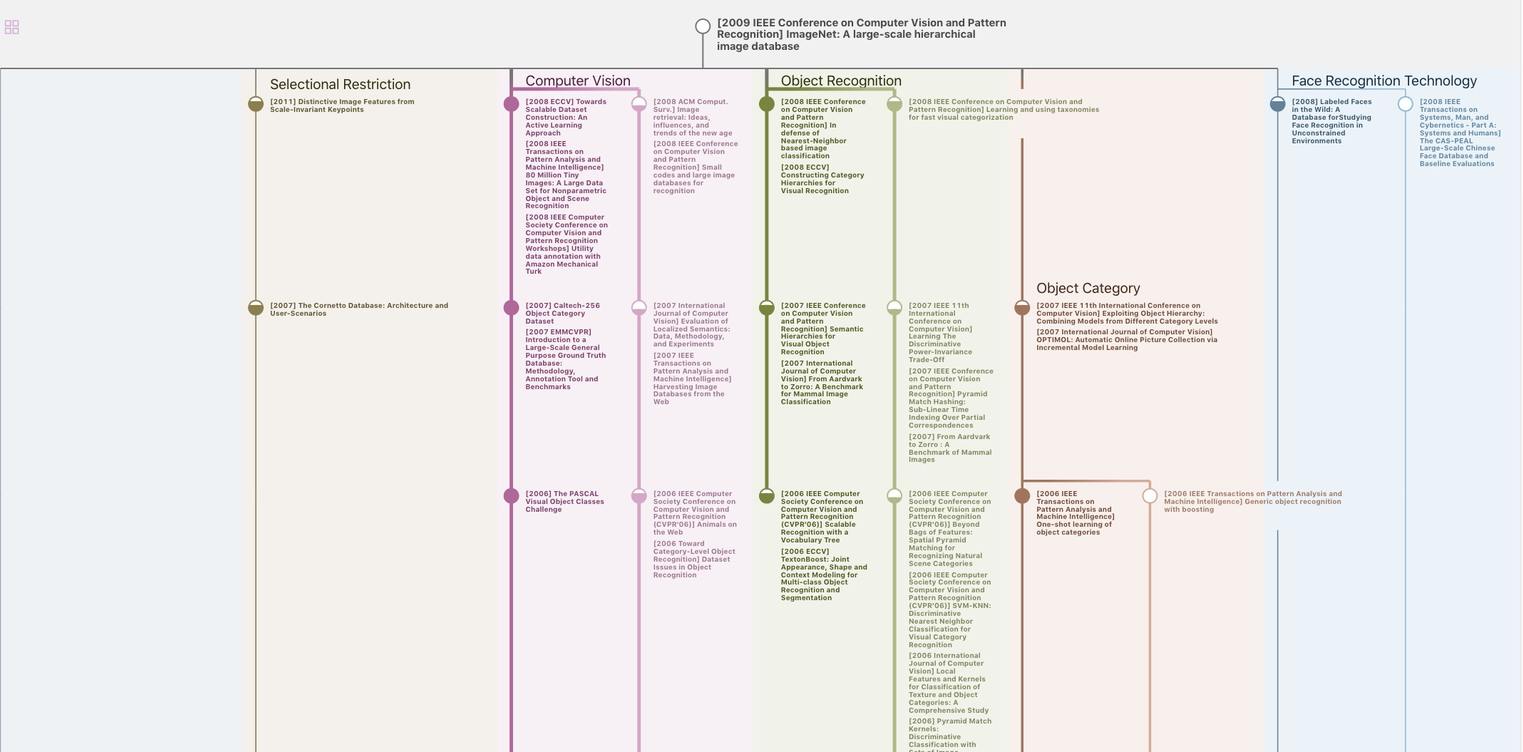
生成溯源树,研究论文发展脉络
Chat Paper
正在生成论文摘要