Dimensionality Reduction of Hyperspectral Imagery Based on Spatial-Spectral Manifold Learning
IEEE Transactions on Cybernetics(2020)
摘要
The graph embedding (GE) methods have been widely applied for dimensionality reduction of hyperspectral imagery (HSI). However, a major challenge of GE is how to choose the proper neighbors for graph construction and explore the spatial information of HSI data. In this paper, we proposed an unsupervised dimensionality reduction algorithm called spatial-spectral manifold reconstruction preserving embedding (SSMRPE) for HSI classification. At first, a weighted mean filter (WMF) is employed to preprocess the image, which aims to reduce the influence of background noise. According to the spatial consistency property of HSI, SSMRPE utilizes a new spatial-spectral combined distance (SSCD) to fuse the spatial structure and spectral information for selecting effective spatial-spectral neighbors of HSI pixels. Then, it explores the spatial relationship between each point and its neighbors to adjust the reconstruction weights to improve the efficiency of manifold reconstruction. As a result, the proposed method can extract the discriminant features and subsequently improve the classification performance of HSI. The experimental results on the PaviaU and Salinas hyperspectral data sets indicate that SSMRPE can achieve better classification results in comparison with some state-of-the-art methods.
更多查看译文
关键词
Manifolds,Hyperspectral imaging,Feature extraction,Dimensionality reduction,Germanium,Image reconstruction,Dimensionality reduction,discriminant features,hyperspectral remote sensing,manifold learning,spatial-spectral combined distance (SSCD)
AI 理解论文
溯源树
样例
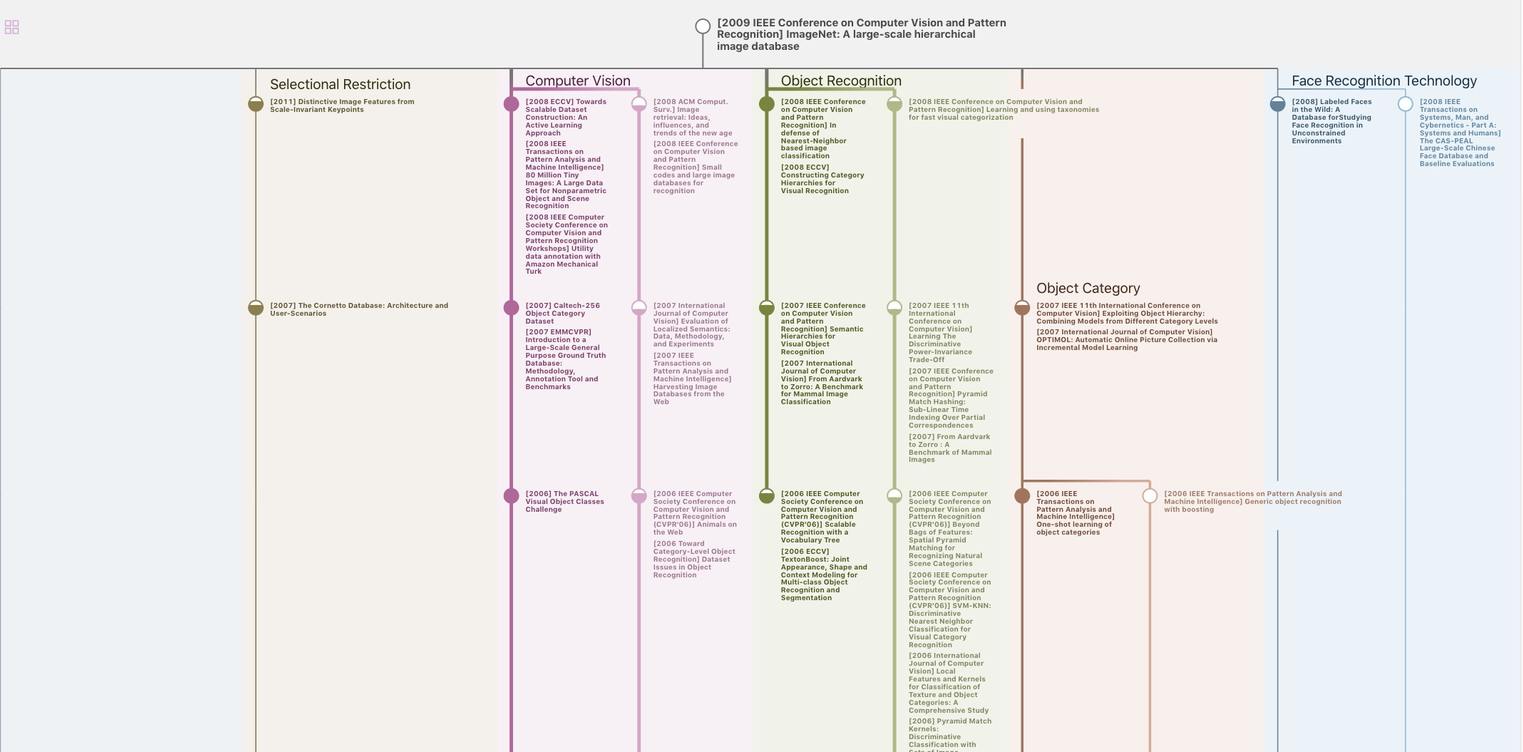
生成溯源树,研究论文发展脉络
Chat Paper
正在生成论文摘要