Face Hallucination Revisited: An Exploratory Study on Dataset Bias
2019 IEEE/CVF Conference on Computer Vision and Pattern Recognition Workshops (CVPRW)(2018)
摘要
Contemporary face hallucination (FH) models exhibit considerable ability to reconstruct high-resolution (HR) details from low-resolution (LR) face images. This ability is commonly learned from examples of corresponding HR-LR image pairs, created by artificially down-sampling the HR ground truth data. This down-sampling (or degradation) procedure not only defines the characteristics of the LR training data, but also determines the type of image degradations the learned FH models are eventually able to handle. If the image characteristics encountered with real-world LR images differ from the ones seen during training, FH models are still expected to perform well, but in practice may not produce the desired results. In this paper we study this problem and explore the bias introduced into FH models by the characteristics of the training data. We systematically analyze the generalization capabilities of several FH models in various scenarios, where the image the degradation function does not match the training setup and conduct experiments with synthetically downgraded as well as real-life low-quality images. We make several interesting findings that provide insight into existing problems with FH models and point to future research directions.
更多查看译文
关键词
real-world LR images,training setup,real-life low-quality images,face hallucination revisited,dataset bias,contemporary face hallucination models,low-resolution face images,HR-LR image pairs,LR training data,image degradations,learned FH models,image characteristics,high-resolution detail reconstruction,HR ground truth data downsampling,degradation function
AI 理解论文
溯源树
样例
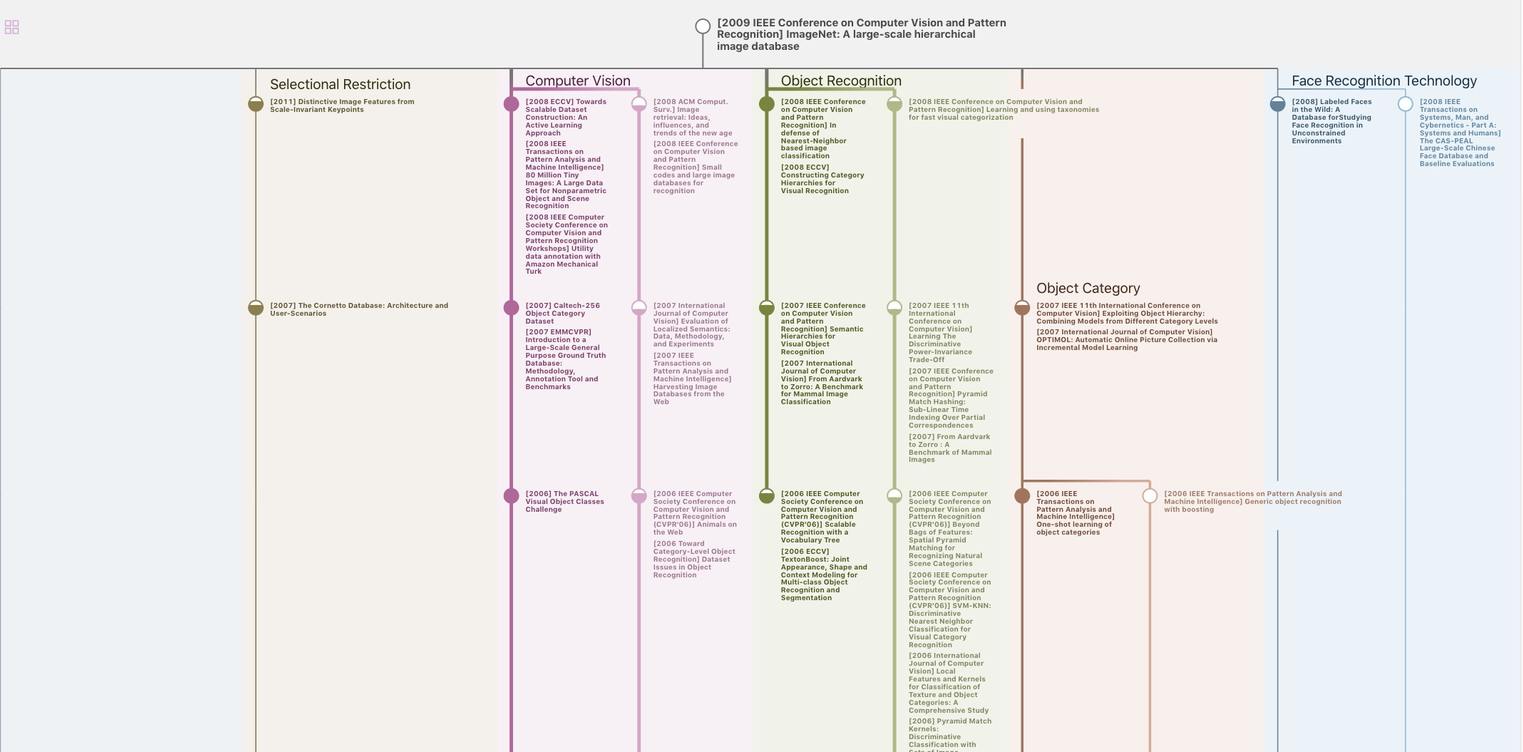
生成溯源树,研究论文发展脉络
Chat Paper
正在生成论文摘要