Stanza: Distributed Deep Learning with Small Communication Footprint.
CoRR(2018)
摘要
The parameter server architecture is prevalently used for distributed deep learning. Each worker machine in a parameter server system trains the complete model, which leads to a hefty amount of network data transfer between workers and servers. We empirically observe that the data transfer has a non-negligible impact on training time. To tackle the problem, we design a new distributed training system called Stanza. Stanza exploits the fact that in many models such as convolution neural networks, most data exchange is attributed to the fully connected layers, while most computation is carried out in convolutional layers. Thus, we propose layer separation in distributed training: the majority of the nodes just train the convolutional layers, and the rest train the fully connected layers only. Gradients and parameters of the fully connected layers no longer need to be exchanged across the cluster, thereby substantially reducing the data transfer volume. We implement Stanza on PyTorch and evaluate its performance on Azure and EC2. Results show that Stanza accelerates training significantly over current parameter server systems: on EC2 instances with Tesla V100 GPU and 10Gb bandwidth for example, Stanza is 1.34x--13.9x faster for common deep learning models.
更多查看译文
关键词
Backpropagation Learning,Deep Learning,Feedforward Neural Networks,Stochastic Gradient Descent,Self-Organizing Maps
AI 理解论文
溯源树
样例
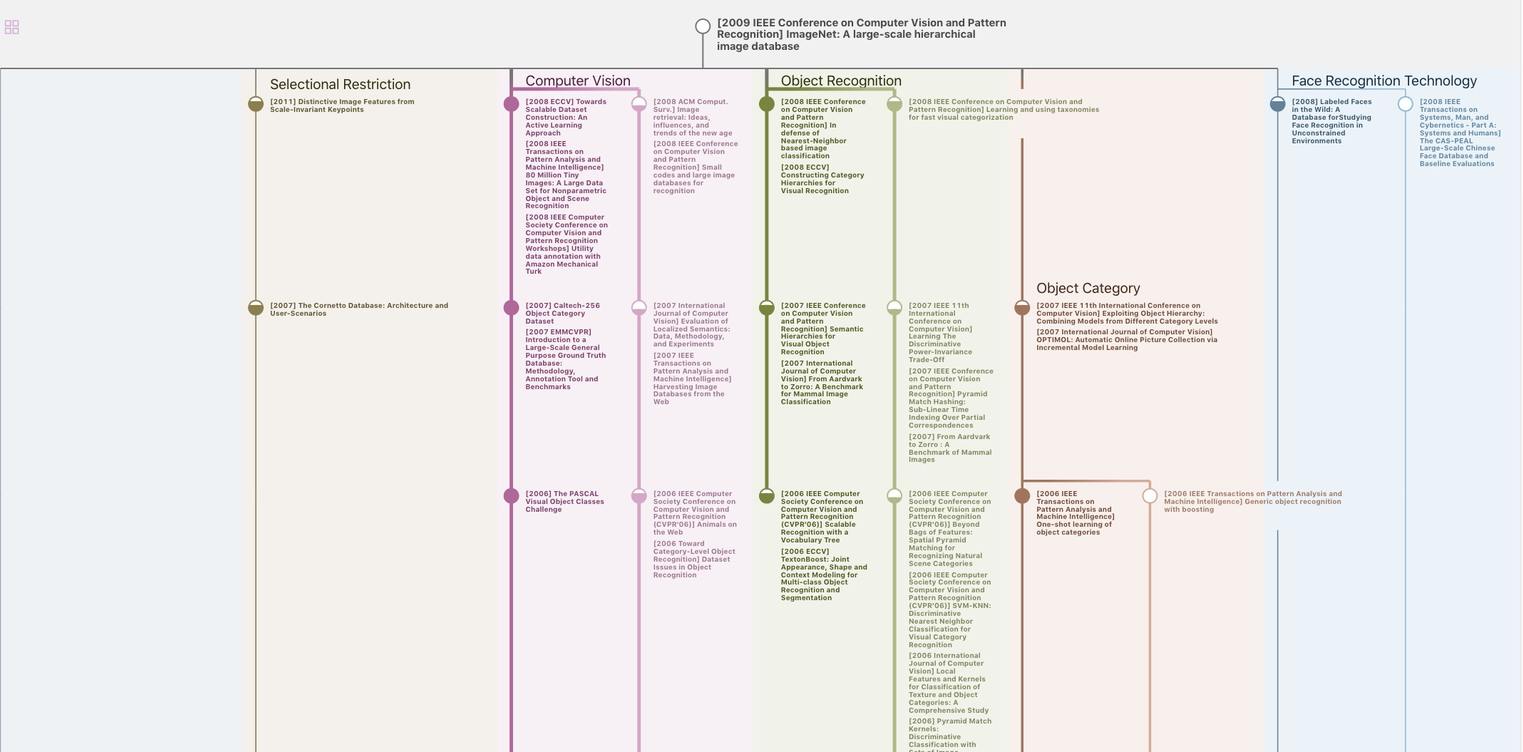
生成溯源树,研究论文发展脉络
Chat Paper
正在生成论文摘要