Derivative-based acceleration of general vector machine
Soft Comput.(2017)
摘要
General vector machine (GVM) is one of supervised learning machine, which is based on three-layer neural network. It is capable of constructing a learning model with limited amount of data. Generally, it employs Monte Carlo algorithm (MC) to adjust weights of the underlying network. However, GVM is time-consuming at training and is not efficient when compared with other learning algorithm based on gradient descent learning. In this paper, we present a derivative-based Monte Carlo algorithm (DMC) to accelerate the training of GVM. Our experimental results indicate that DMC algorithm is faster than the original MC method. Specifically, the training time of our DMC algorithm in GVM for function fitting is also less than some gradient descent-based methods, in which we compare DMC with back-propagation neural network. Experimental results indicate that our algorithm is promising for training GVM.
更多查看译文
关键词
General vector machine,Neural network,Gradient descent,Derivative,Back-propagation
AI 理解论文
溯源树
样例
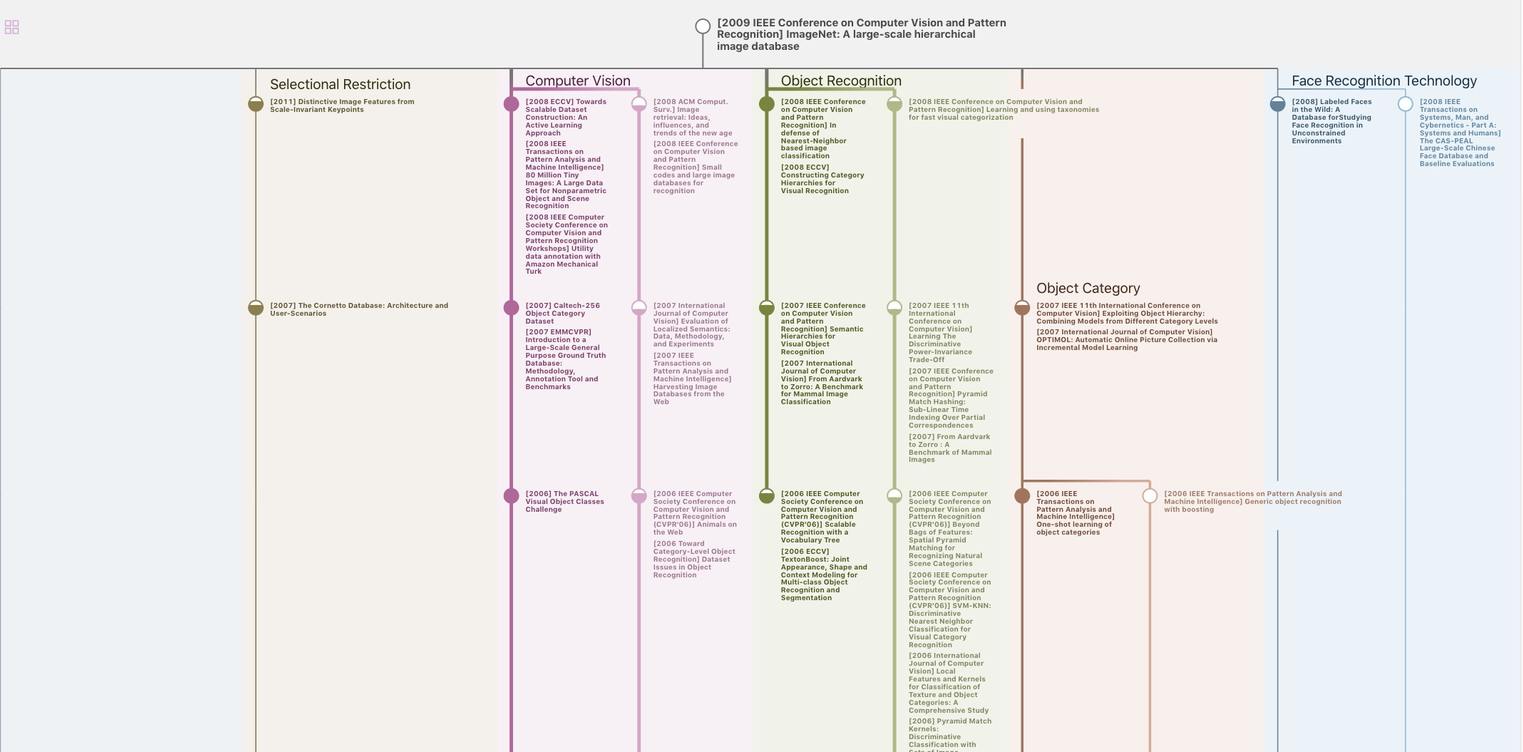
生成溯源树,研究论文发展脉络
Chat Paper
正在生成论文摘要