Switching Between Metamodeling Frameworks For Efficient Multi-Objective Optimization
2018 IEEE SYMPOSIUM SERIES ON COMPUTATIONAL INTELLIGENCE (IEEE SSCI)(2018)
摘要
Evaluating computationally expensive objective and constraint functions is one of the main challenges faced when solving real-world optimization problems. For handling such problems, it is common to use a metamodeling approach. A metamodel is initially formed using a few exact solution evaluations and then optimized the metamodel to find a few in-fill solutions. The in-fill solutions are then evaluated exactly and another metamodel is formed. This procedure is continued in a progressive manner until all allocated budget of solutions are evaluated. In multi-objective optimization, there are several ways to build and utilize metamodeling approaches. Authors have previously proposed a taxonomy of different metamodeling approaches for multi-objective optimization and provided a comparative study highlighting the advantages and disadvantages of them. In this paper, we argue that it is more efficient to use different metamodeling approaches at different stages of the optimization process and than propose several manual switching strategies between the metamodeling methods. We also introduce a trust region based method to achieve a better convergence behavior. We use the well-known Kriging approach as the core metamodeling method in this study. Our results show the efficacy of the proposed approach on challenging multi-objective optimization problems using a limited budget of high-fidelity evaluations.
更多查看译文
关键词
Metamodeling, Evolutionary multi-objective optimization, Kriging, Trust Region, Expensive optimization
AI 理解论文
溯源树
样例
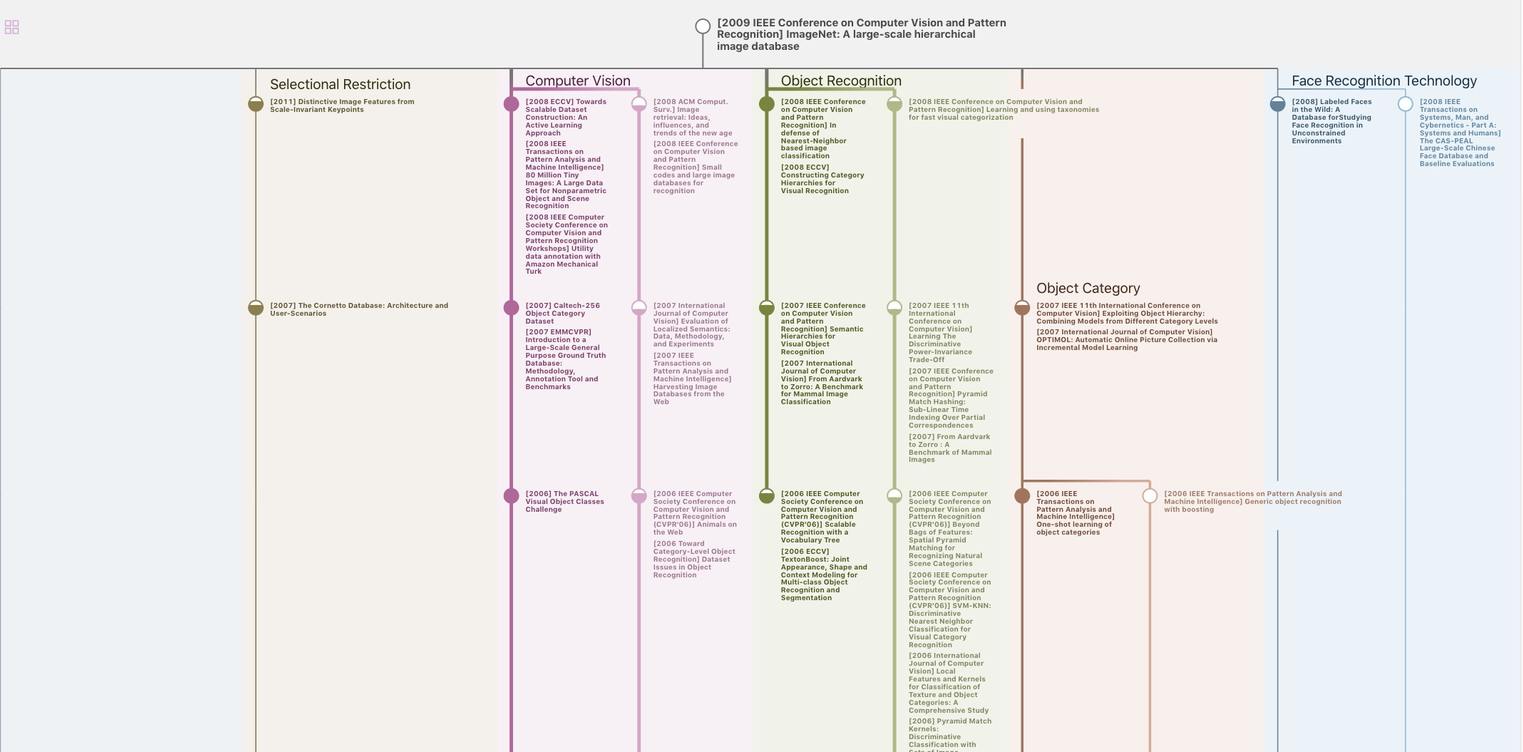
生成溯源树,研究论文发展脉络
Chat Paper
正在生成论文摘要