Drift Calibration for Machine Olfaction with an Online Active Learning Approach
2018 5th International Conference on Systems and Informatics (ICSAI)(2018)
摘要
Drift counteraction of electronic nose (E-nose) systems has become a challenging topic in machine olfaction. It is considered to be an emerging and promising analysis approach for complicated gas compounds. Many studies incline to solve the drift problem by algorithms which should be heavily dependent on calibrations. Common calibration procedure requires sufficient amount of samples with entire kinds of labels to demonstrate the drifting distributions. However, such requirement is costly and laborious to achieve, even unattainable in continuous online scenarios. We therefore make two contributions to online drift calibrations. Primarily we redesign an active learning (AL) framework oriented online mode with limited storage resources. Secondly, a novel instance-selection method, named min-max confidence (MMC) strategy, for AL framework is proposed to handle online data. Compared with conventional strategies, the MMC method emphasizes the balance of selected instances on data distribution, which helps to choose the most valuable instance to label under drift condition. We adopt two E-nose drift databases to assess the proposed framework and strategy. The results of experiments have indicated that the satisfactory recognition accuracies could be obtained from the active AL framework rather than other drift-counteraction frameworks. The MMC strategy coupled with the active AL framework reaches the highest accuracy in comparison.
更多查看译文
关键词
e-nose,drift counteraction,active learning,online working
AI 理解论文
溯源树
样例
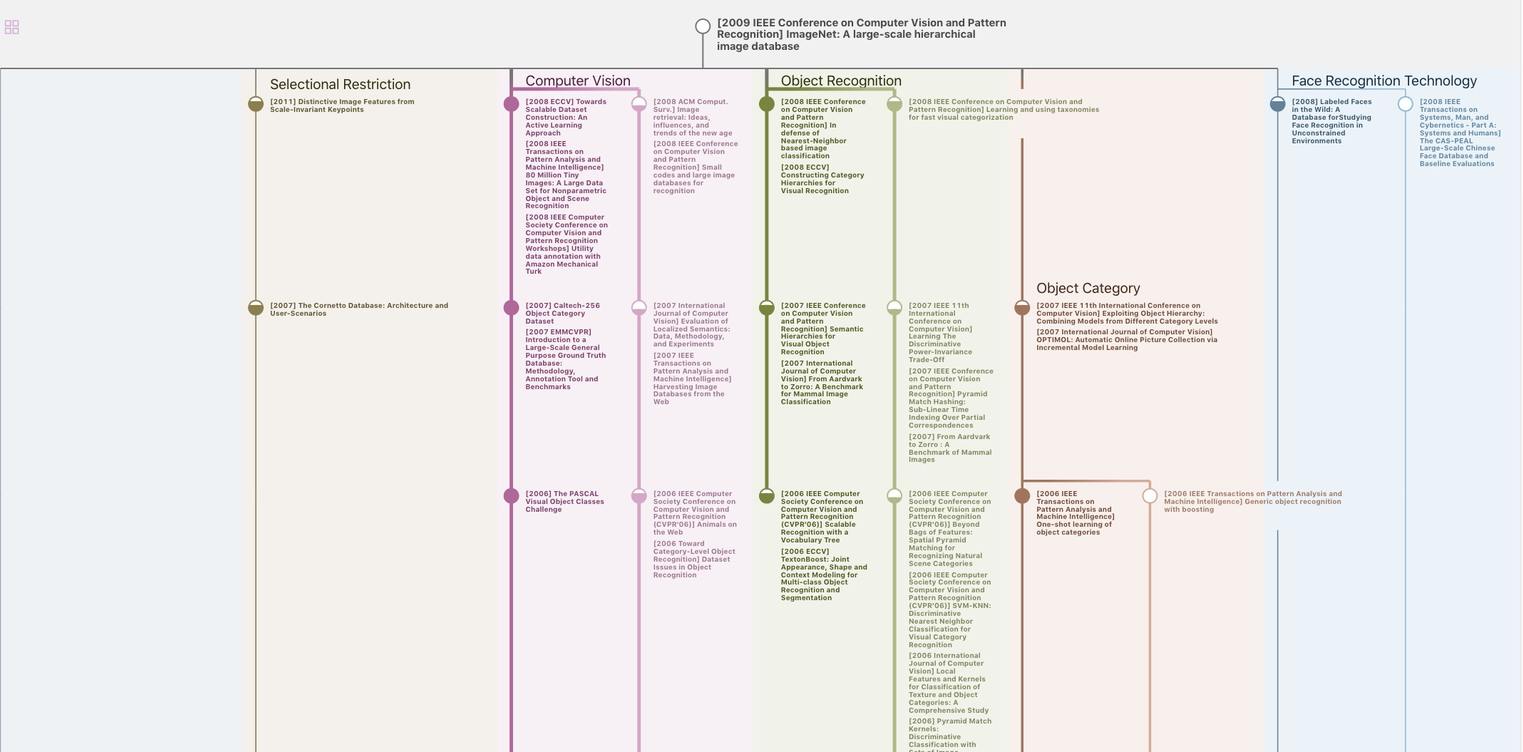
生成溯源树,研究论文发展脉络
Chat Paper
正在生成论文摘要