Utility Balanced Classification for Automatic Electronic Medical Record Analysis
2018 5TH INTERNATIONAL CONFERENCE ON SYSTEMS AND INFORMATICS (ICSAI)(2018)
摘要
Imbalanced data classification is a critical issue and plays an important role in data analysis, especially in automatic clinical diagnosis and treatment. However, since practical applications, for example, clinical data analysis usually have high complexity and diversity, conventional classification method suffers from huge cost and high unreliability while facing complex clinical data. Therefore it is challenging to obtain an effective, reliable, and precise method. In this paper, we propose a utility balanced classifier (UBC) for diagnostic prediction from electronic medical record automatically. Our UBC introduces two novel innovations to handle imbalanced data: (1) the concept of utility describing the effectiveness of the data during classification, which effectively handles the nonlinear relationship between medical record features and quantitative evaluation parameters. (2) the application of the focal loss processing imbalanced data, which plays an important role to correct the mislabeled data. Experiments show our method achieves high accuracy on a comprehensive clinical dataset, which indicates its huge practical value in clinical diagnosis and treatment.
更多查看译文
关键词
Imbalanced data, electronic medical record, classification, utility
AI 理解论文
溯源树
样例
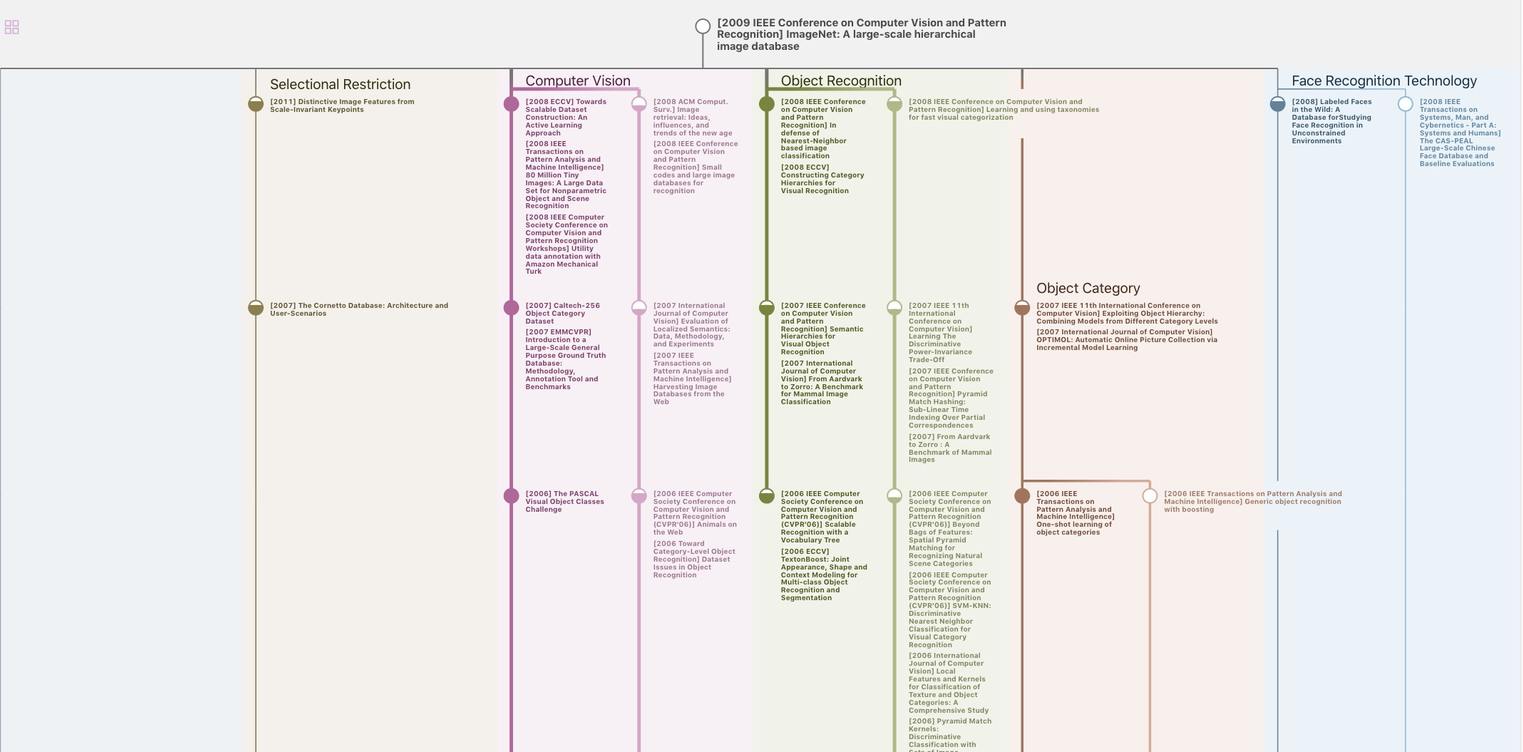
生成溯源树,研究论文发展脉络
Chat Paper
正在生成论文摘要