A Coarse-to-Fine Model for Rail Surface Defect Detection
IEEE Transactions on Instrumentation and Measurement(2019)
摘要
Computer vision systems have attracted much attention in recent years for use in detecting surface defects on rails; however, accurate and efficient recognition of possible defects remains challenging due to the variations shown by defects and also noise. This paper proposes a coarse-to-fine model (CTFM) to identify defects at different scales. The model works on three scales from coarse to fine: subimage level, region level, and pixel level. At the subimage level, the background subtraction model exploits row consistency in the longitudinal direction, and strongly filters the defect-free range, leaving roughly identified subimages within which defects may exist. At the next level, the region extraction model, inspired by visual saliency models, locates definite defect regions using phase-only Fourier transforms. At the finest level, the pixel subtraction model uses pixel consistency to refine the shape of each defect. The proposed method is evaluated using Type-I and Type-II rail surface defect detection data sets and an actual rail line. The experimental results show that CTFM outperforms state-of-the-art methods according to both the pixel-level index and the defect-level index.
更多查看译文
关键词
Rails,Inspection,Shape,Computational modeling,Indexes,Testing,Gallium nitride
AI 理解论文
溯源树
样例
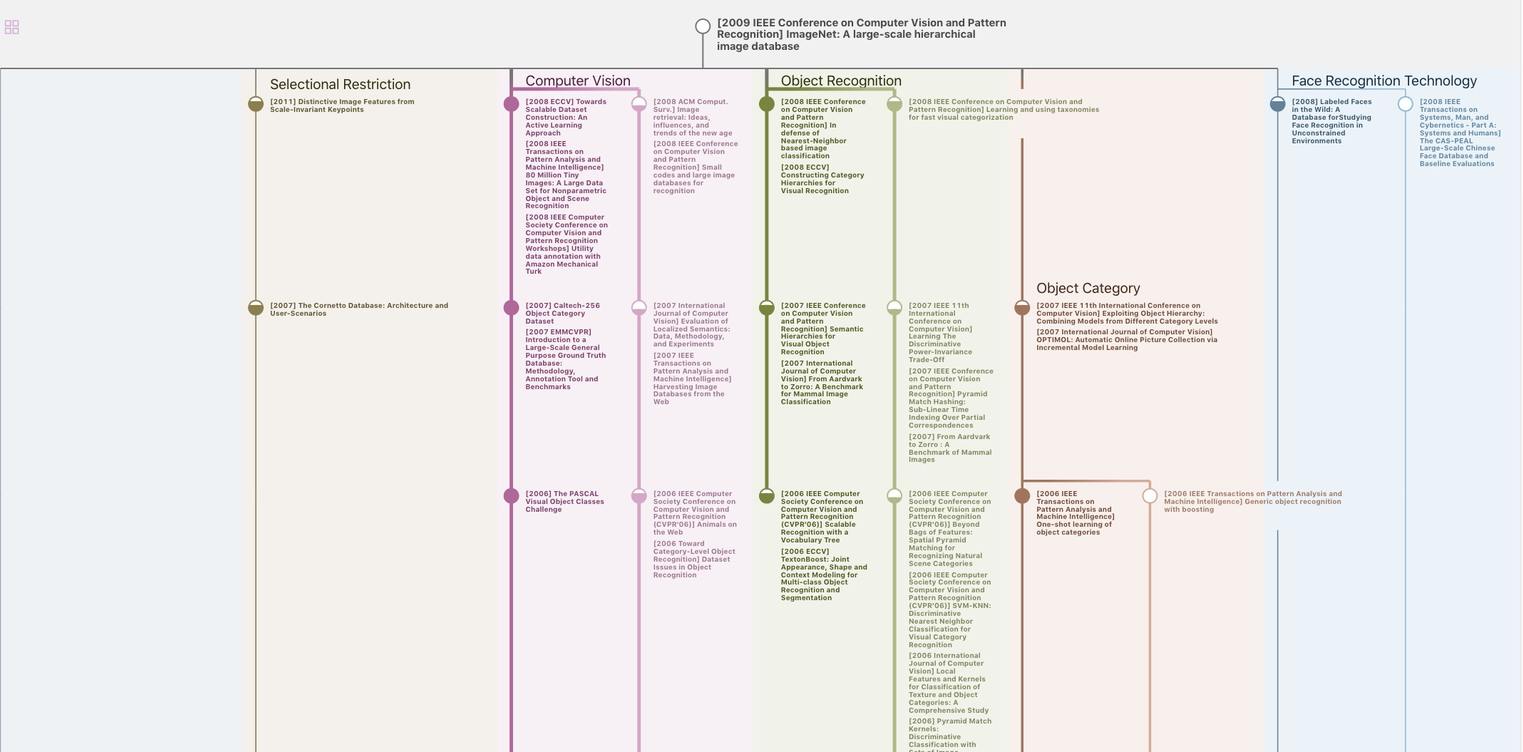
生成溯源树,研究论文发展脉络
Chat Paper
正在生成论文摘要