Multi-Scale Processing Of Noisy Images Using Edge Preservation Losses
2020 25TH INTERNATIONAL CONFERENCE ON PATTERN RECOGNITION (ICPR)(2021)
摘要
Noisy image processing is a fundamental task of computer vision. The first example is the detection of faint edges in noisy images, a challenging problem studied in the last decades. A recent study introduced a fast method to detect faint edges in the highest accuracy among all the existing approaches. Their complexity is nearly linear in the image's pixels and their runtime is seconds for a noisy image. Their approach utilizes a multi-scale binary partitioning of the image. By utilizing the multi-scale U-net architecture, we show in this paper that their method can be dramatically improved in both aspects of run time and accuracy. By training the network on a dataset of binary images, we developed an approach for faint edge detection that works in linear complexity. Our runtime of a noisy image is milliseconds on a GPU. Even though our method is orders of magnitude faster, we still achieve higher accuracy of detection under many challenging scenarios. In addition, we show that our approach to performing multi-scale preprocessing of noisy images using U-net improves the ability to perform other vision tasks under the presence of noise. We prove it on the problems of noisy objects classification and classical image denoising. We show that multi-scale denoising can be carried out by a novel edge preservation loss. As our experiments show, we achieve high-quality results in the three aspects of faint edge detection, noisy image classification, and natural image denoising.
更多查看译文
关键词
Training,Runtime,Image edge detection,Noise reduction,Graphics processing units,Robustness,Complexity theory
AI 理解论文
溯源树
样例
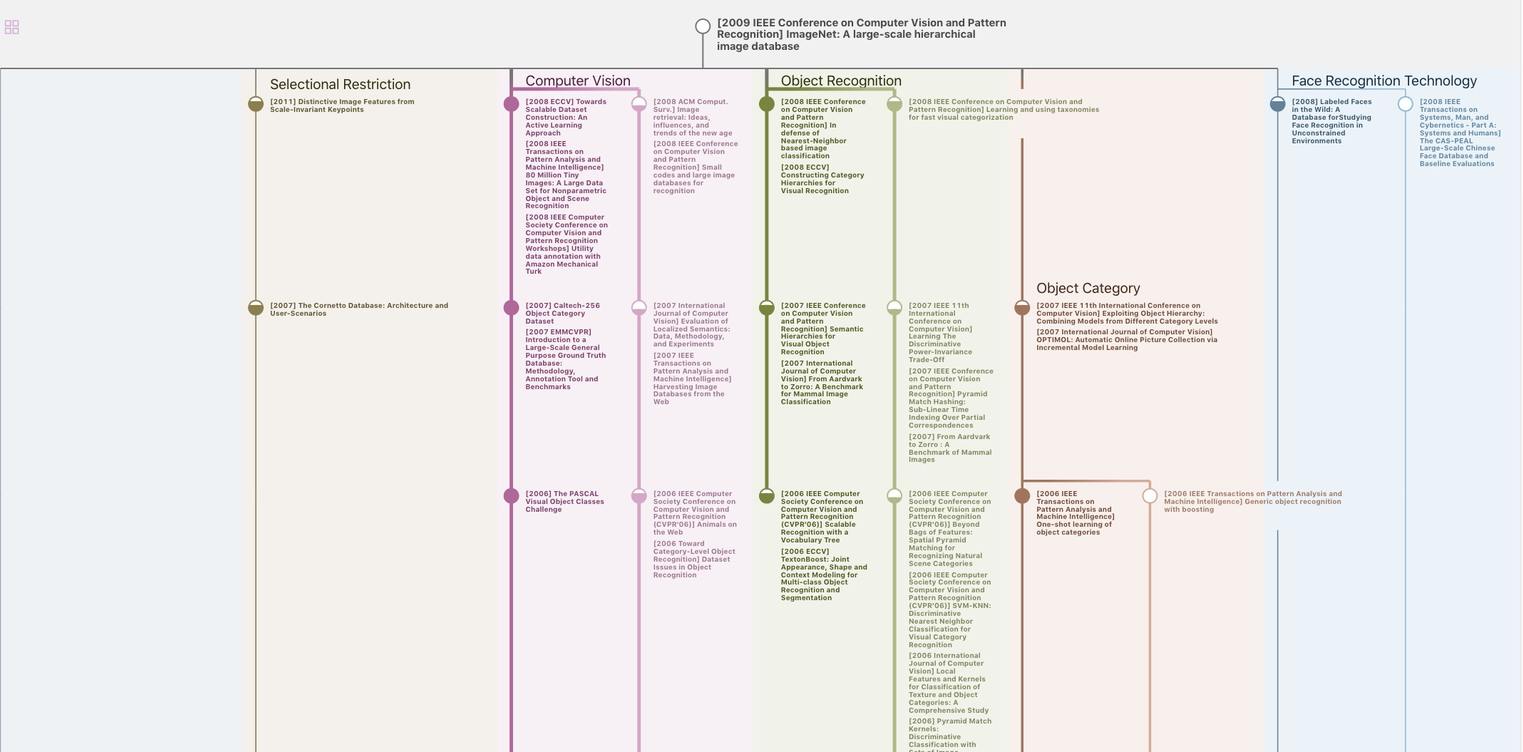
生成溯源树,研究论文发展脉络
Chat Paper
正在生成论文摘要