Deep Grid Net (Dgn): A Deep Learning System For Real-Time Driving Context Understanding
2019 THIRD IEEE INTERNATIONAL CONFERENCE ON ROBOTIC COMPUTING (IRC 2019)(2019)
摘要
Grid maps obtained from fused sensory information are nowadays among the most popular approaches for motion planning for autonomous driving cars. In this paper, we introduce Deep Grid Net (DGN), a deep learning (DL) system designed for understanding the context in which an autonomous car is driving. DGN incorporates a learned driving environment representation based on Occupancy Grids (OG) obtained from raw Lidar data and constructed on top of the Dempster-Shafer (DS) theory. The predicted driving context is further used for switching between different driving strategies implemented within EB robinos, Elektrobit's Autonomous Driving (AD) software platform. Based on genetic algorithms (GAs), we also propose a neuroevolutionary approach for learning the tuning hyperparameters of DGN. The performance of the proposed deep network has been evaluated against similar competing driving context estimation classifiers.
更多查看译文
关键词
Training,Real-time systems,Laser radar,Roads,Genetic algorithms,Robots
AI 理解论文
溯源树
样例
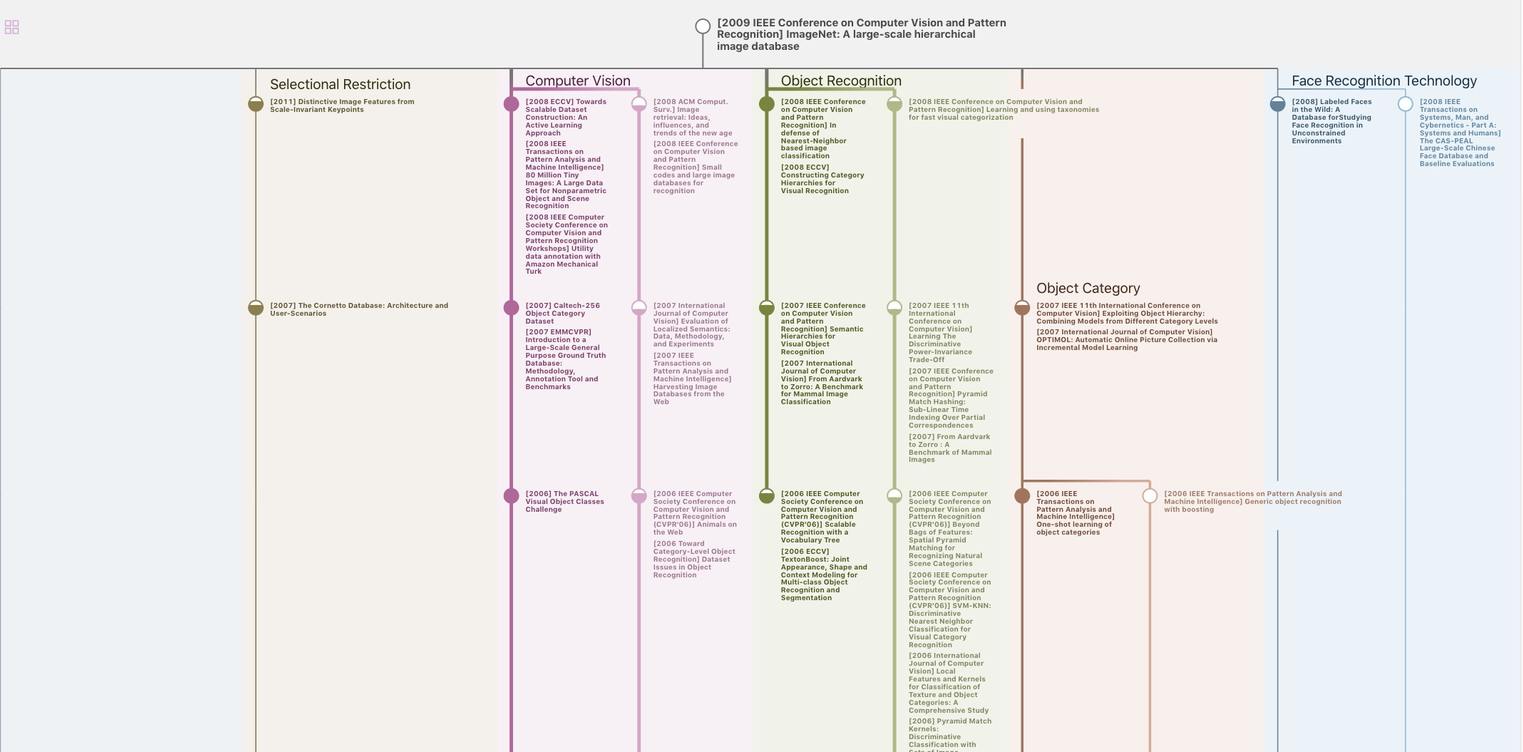
生成溯源树,研究论文发展脉络
Chat Paper
正在生成论文摘要