Interactively shaping robot behaviour with unlabeled human instructions
Autonomous Agents and Multi-Agent Systems(2020)
摘要
In this paper, we propose a framework that enables a human teacher to shape a robot behaviour by interactively providing it with unlabeled instructions. We ground the meaning of instruction signals in the task-learning process, and use them simultaneously for guiding the latter. We implement our framework as a modular architecture, named TICS (Task-Instruction-Contingency-Shaping) that combines different information sources: a predefined reward function, human evaluative feedback and unlabeled instructions. This approach provides a novel perspective for robotic task learning that lies between Reinforcement Learning and Supervised Learning paradigms. We evaluate our framework both in simulation and with a real robot. The experimental results demonstrate the effectiveness of our framework in accelerating the task-learning process and in reducing the number of required teaching signals.
更多查看译文
关键词
Interactive machine learning,Human–robot interaction,Shaping,Reinforcement learning,Unlabeled instructions
AI 理解论文
溯源树
样例
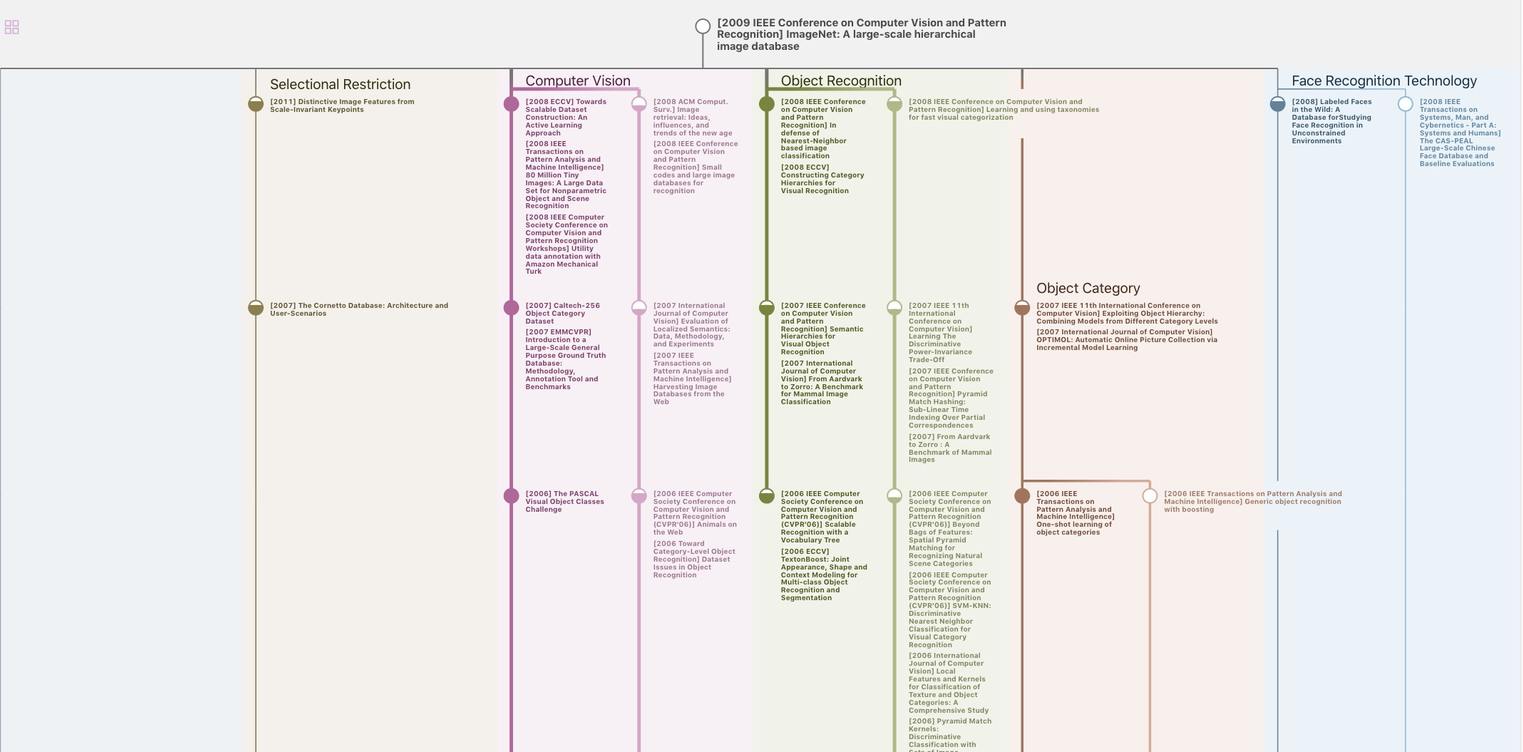
生成溯源树,研究论文发展脉络
Chat Paper
正在生成论文摘要