Optimal Nonparametric Inference via Deep Neural Network
JOURNAL OF MATHEMATICAL ANALYSIS AND APPLICATIONS(2021)
摘要
Deep neural network is a state-of-art method in modern science and technology. Much statistical literature have been devoted to understanding its performance in nonparametric estimation, whereas the results are suboptimal due to a redundant logarithmic sacrifice. In this paper, we show that such log-factors are not necessary. We derive upper bounds for the $L^2$ minimax risk in nonparametric estimation. Sufficient conditions on network architectures are provided such that the upper bounds become optimal (without log-sacrifice). Our proof relies on an explicitly constructed network estimator based on tensor product B-splines. We also derive asymptotic distributions for the constructed network and a relating hypothesis testing procedure. The testing procedure is further proven as minimax optimal under suitable network architectures.
更多查看译文
关键词
Deep neural network,Nonparametric inference,Tensor product B-splines,Optimal minimax risk bound,Asymptotic distribution,Nonparametric testing
AI 理解论文
溯源树
样例
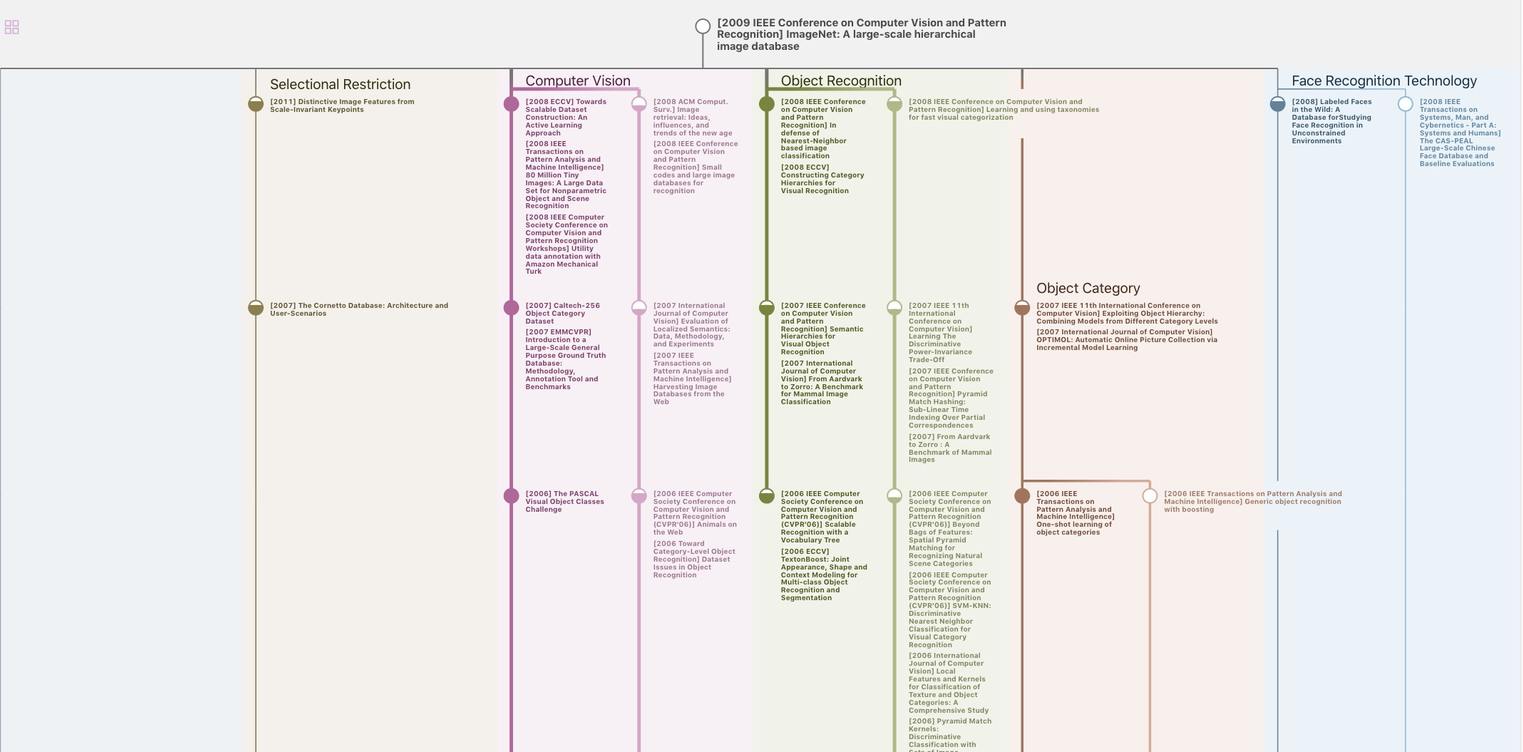
生成溯源树,研究论文发展脉络
Chat Paper
正在生成论文摘要