Communication Avoiding Multigrid Preconditioned Conjugate Gradient Method for Extreme Scale Multiphase CFD Simulations
2018 IEEE/ACM 9th Workshop on Latest Advances in Scalable Algorithms for Large-Scale Systems (scalA)(2018)
摘要
A communication avoiding (CA) multigrid preconditioned conjugate gradient method (CAMGCG) is applied to the pressure Poisson equation in a multiphase CFD code JUPITER, and its computational performance and convergence property are compared against CA Krylov methods. A new geometric multigrid preconditioner is developed using a preconditioned Chebyshev iteration smoother, in which no global reduction communication is needed, halo data communication is reduced by a mixed precision approach, and eigenvalues are computed using the CA Lanczos method. In the JUPITER code, the CAMGCG solver has robust convergence properties regardless of the problem size, and shows both communication reduction and convergence improvement, leading to higher performance gain than CA Krylov solvers, which achieve only the former. The CAMGCG solver is applied to extreme scale multiphase CFD simulations with $\sim 90$ billion DOFs, and it is shown that compared with a preconditioned CG solver, the number of iterations, and thus, All\_Reduce is reduced to $\sim 1/800$, and $\sim 11.6\times$ speedup is achieved with keeping excellent strong scaling up to 8,000 KNLs on the Oakforest-PACS.
更多查看译文
关键词
Convergence,Jupiter,Computational fluid dynamics,Poisson equations,Chebyshev approximation,Computational modeling,Iterative methods
AI 理解论文
溯源树
样例
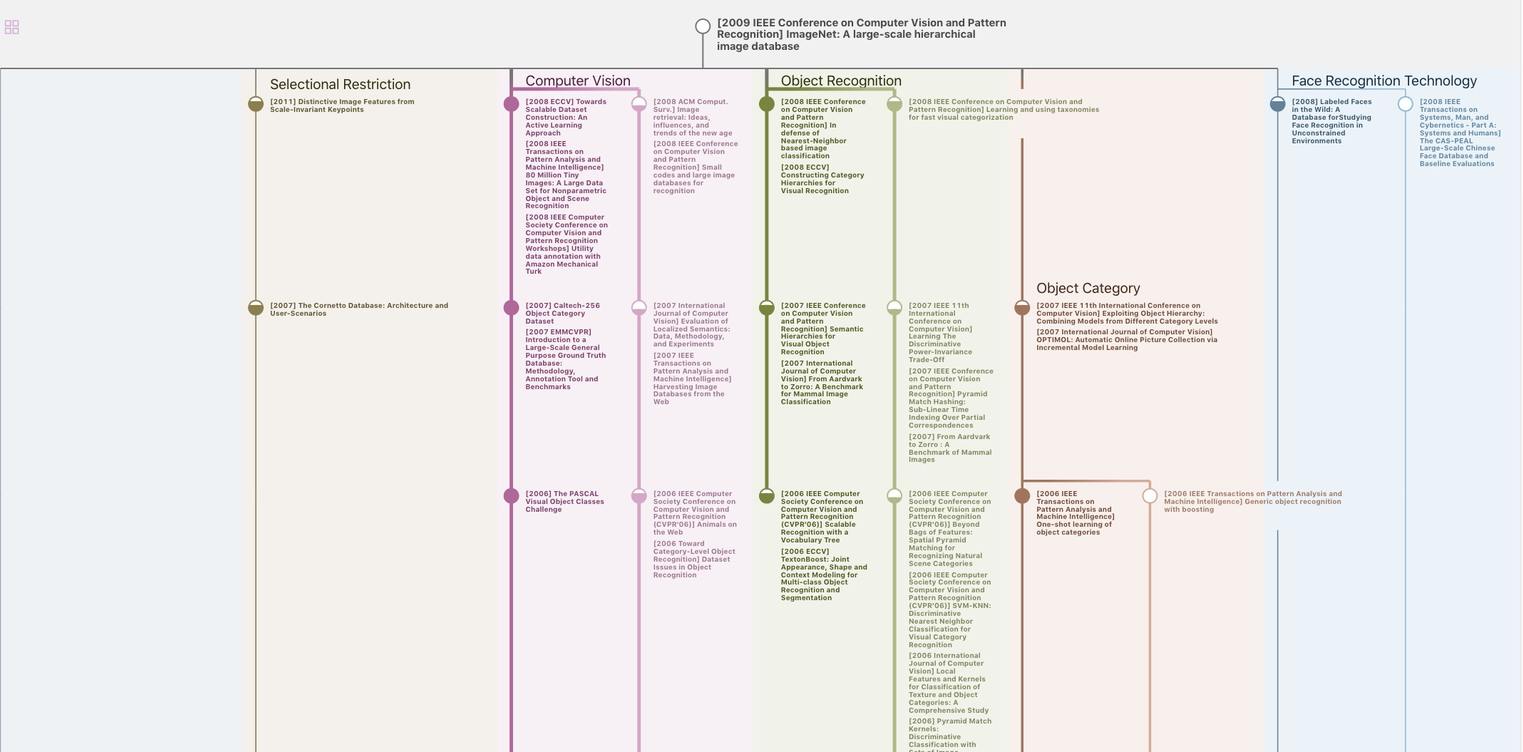
生成溯源树,研究论文发展脉络
Chat Paper
正在生成论文摘要