Bayesian Inference of a Finite Population Mean Under Length-Biased Sampling
arxiv(2019)
摘要
We present a robust Bayesian method to analyze forestry data when samples are selected with probability proportional to length from a finite population of unknown size. Specifically, we use Bayesian predictive inference to estimate the finite population mean of shrub widths in a limestone quarry dominated by re-growth of mountain mahogany. The data on shrub widths are collected using transect sampling and it is assumed that the probability that a shrub is selected is proportional to its width; this is length-biased sampling. In this type of sampling, the population size is also unknown and this creates an additional challenge. The quantity of interest is average finite population shrub width and the total shrub area of the quarry can be estimated. Our method is assisted by using the three-parameter generalized gamma distribution, thereby robustifying our procedure against a possible model failure. Using conditional predictive ordinates, we show that the model, which accommodates length bias, performs better than the model that does not. In the Bayesian computation, we overcome a technical problem associated with Gibbs sampling by using a random sampler.
更多查看译文
关键词
finite population mean,bayesian inference,length-biased
AI 理解论文
溯源树
样例
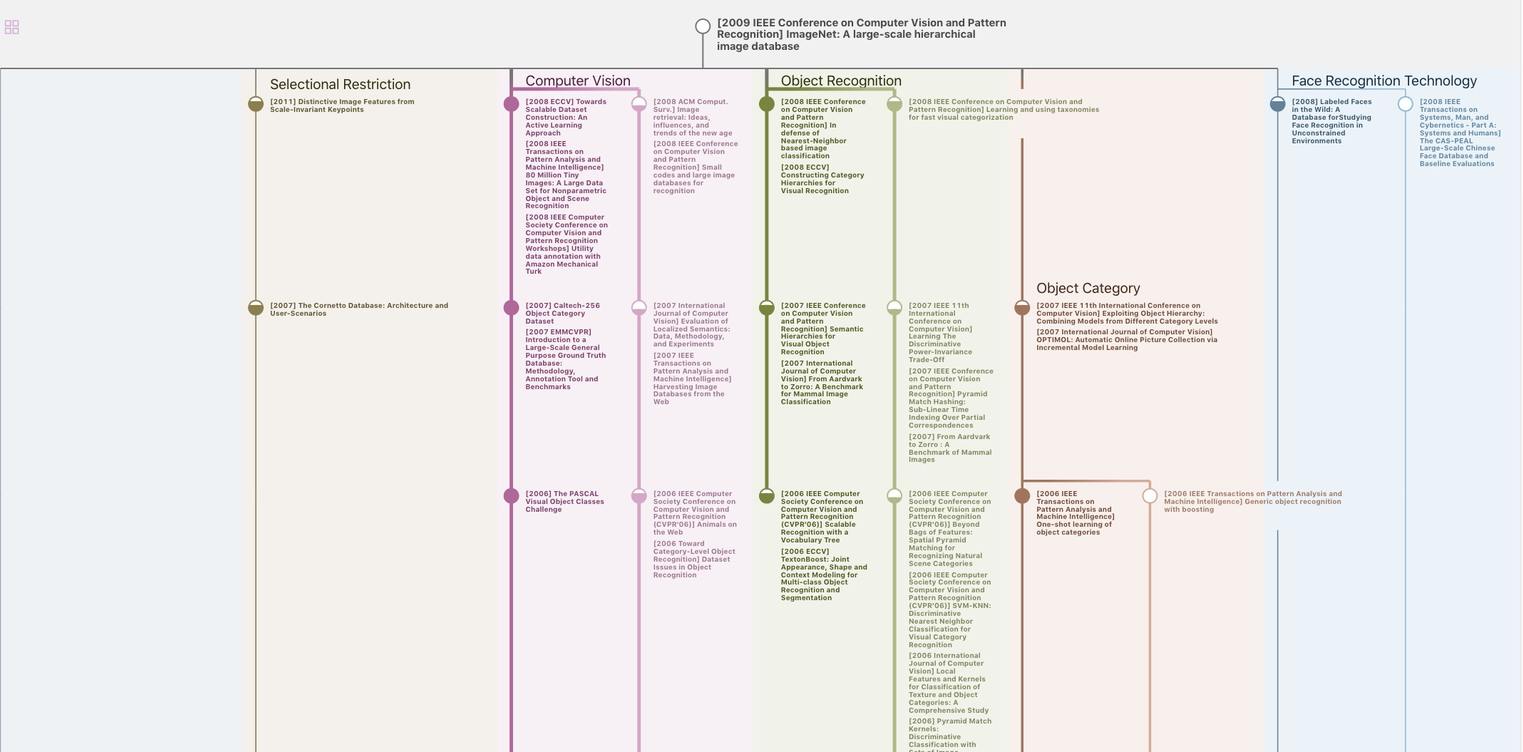
生成溯源树,研究论文发展脉络
Chat Paper
正在生成论文摘要