Effort Allocation and Statistical Inference for 1-dimensional Multistart stochastic Gradient Descent.
WSC(2018)
摘要
Multistart stochastic gradient descent methods are widely used for gradient-based stochastic global optimization. While these methods are effective relative to other approaches for these challenging problems, they seem to waste computational resources: when several starts are run to convergence at the same local optimum, all but one fail to produce useful information; when a start converges to a local optimum worse than an incumbent solution, it also fails to produce useful information. For problems with a one-dimensional input, we propose a rule for allocating computational effort across starts, Most Likely to Succeed (MLS), which allocates more resources to the most promising starts. This allocation rule is based on a novel Gaussian-Process-based statistical model (SGD-GP) for a start's limiting objective value. Unlike previously proposed statistical models, ours agrees with known convergence rates for SGD. Numerical results show our approach outperforms equal allocation of effort across starts and a machine learning method.
更多查看译文
关键词
convergence rates,Gaussian-process-based statistical model,most likely to succeed,machine learning method,allocation rule,computational resources,gradient-based stochastic global optimization,stochastic gradient descent methods,statistical inference
AI 理解论文
溯源树
样例
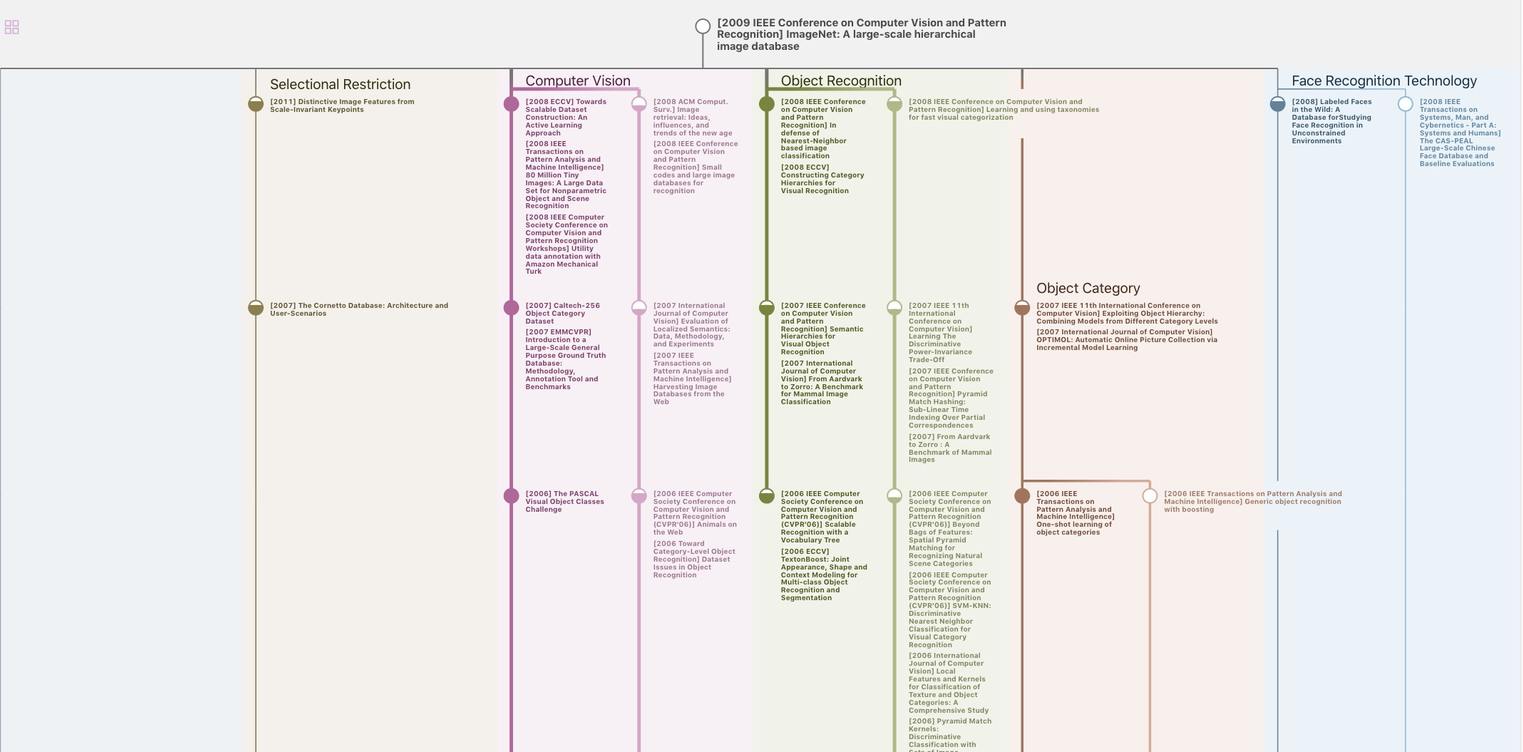
生成溯源树,研究论文发展脉络
Chat Paper
正在生成论文摘要