A non-parametric Approach to simulate Panel Data.
WSC(2018)
摘要
Real world multivariate data mostly contains correlation structure because generally some variables tend to have a similar behavior or some dependency structure. This is due to the nature of data generation process. The variables can contain cross-sectional correlation, correlation between variables at a given time stamp, and temporal correlation, correlation between various observations of a given variable at various timestamps. Also, there are datasets which contain both cross-sectional and temporal correlations. Modeling of such correlation structure in the data is important because it provides us the predictive power and affects the Machine Learning algorithms in various ways, due to relationships within variables. We propose a methodology to simulate a cyber activity dataset, containing both cross-sectional and temporal correlations, also called Panel dataset. The proposed methodology uses non-parametric approach to induce correlations among feature vectors and across time without disturbing the marginal distributions of features.
更多查看译文
关键词
Correlation,Matrix decomposition,Symmetric matrices,Data models,Standards,Machine learning algorithms,Economics
AI 理解论文
溯源树
样例
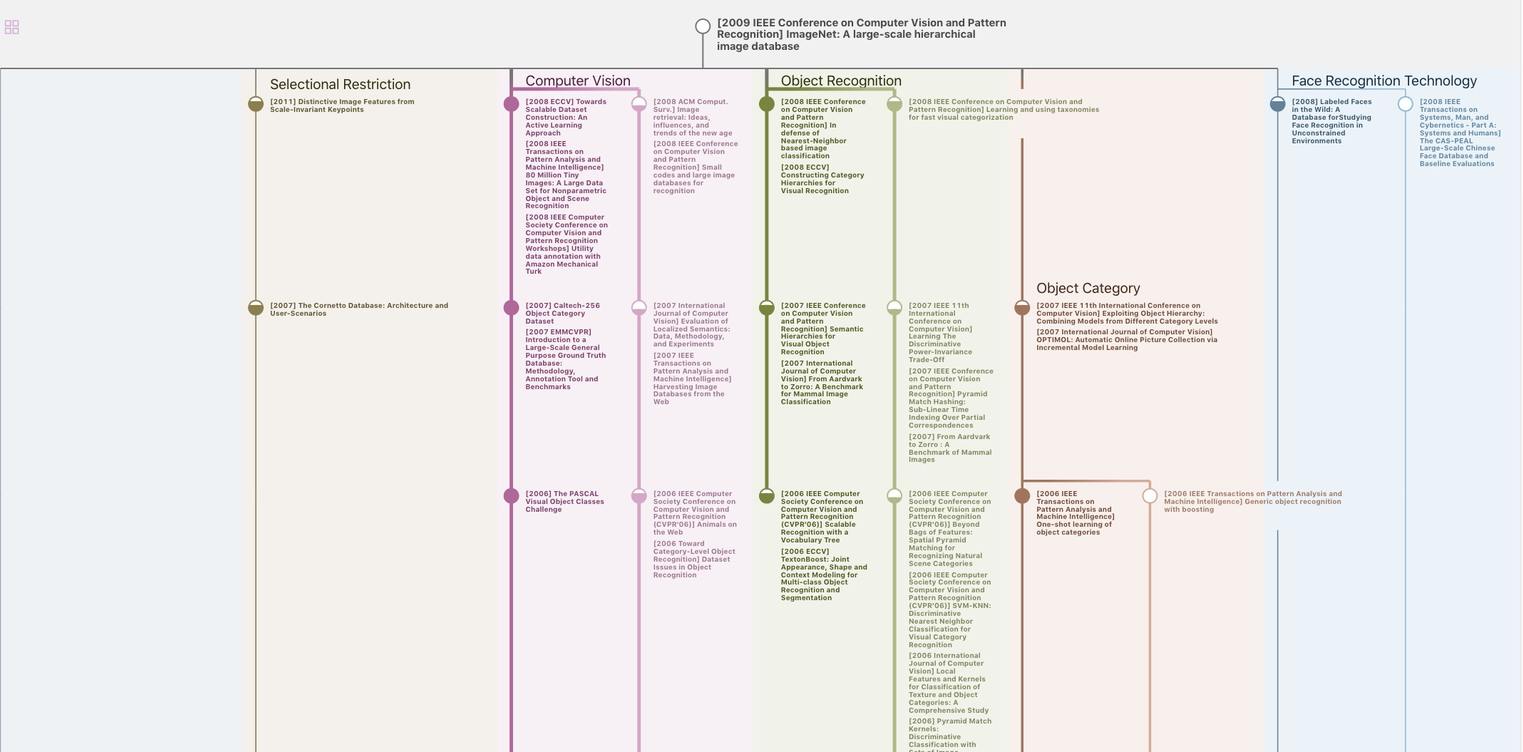
生成溯源树,研究论文发展脉络
Chat Paper
正在生成论文摘要