Adaptive Framework for the Delineation of Homogeneous Forest Areas Based on LiDAR Points.
REMOTE SENSING(2019)
摘要
We propose a flexible framework for automated forest patch delineations that exploits a set of canopy structure features computed from airborne laser scanning (ALS) point clouds. The approach is based on an iterative subdivision of the point cloud using k-means clustering followed by an iterative merging step to tackle oversegmentation. The framework can be adapted for different applications by selecting relevant input features that best measure the intended homogeneity. In our study, the performance of the segmentation framework was tested for the delineation of forest patches with a homogeneous canopy height structure on the one hand and with similar water cycle conditions on the other. For the latter delineation, canopy components that impact interception and evapotranspiration were used, and the delineation was mainly driven by leaf area, tree functional type, and foliage density. The framework was further tested on two scenes covering a variety of forest conditions and topographies. We demonstrate that the delineated patches capture well the spatial distributions of relevant canopy features that are used for defining the homogeneity. The consistencies range from R-2 = 0.84 to R-2 = 0.86 and from R-2 = 0.80 to R-2 = 0.91 for the most relevant features in the delineation of patches with similar height structure and water cycle conditions, respectively.
更多查看译文
关键词
forest structure,k-means clustering,iterative bi-partitioning,overlap merging,ecosystem processes,forestry
AI 理解论文
溯源树
样例
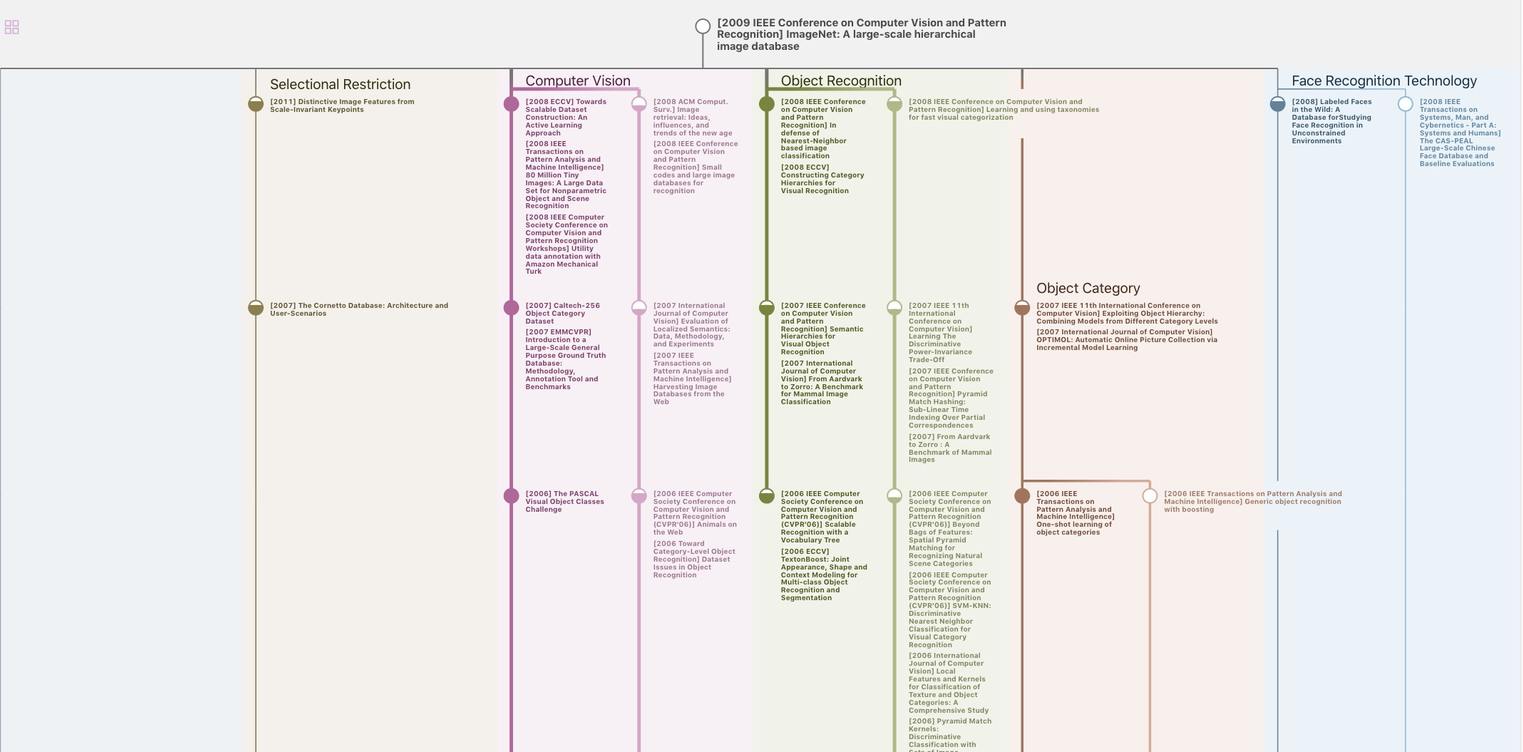
生成溯源树,研究论文发展脉络
Chat Paper
正在生成论文摘要