Deep Learning With Convolutional Neural Networks For Sleep Arousal Detection
2018 COMPUTING IN CARDIOLOGY CONFERENCE (CINC)(2018)
摘要
Sleep arousal influences the quality of sleep and causes health problems. Polysomnography (PSG), a group of biological signals, is often used to diagnose sleep arousal. But it is costly for sleep experts to identify sleep arousal via PSG. Thus, we designed an automatic algorithm to analyze PSG for sleep arousal identification.According to the nature of sleep arousal, we selected electroencephalogram (EEG) from PSG for further analysis. To extract frequency domain information, Welch algorithm was applied to EEG to obtain power spectral density (PSD). And then PSD was fed into a 34-layer convolutional neural network (CNN) for further feature extraction and classification. Shortcut connections were employed across every two convolutional layer to speed up the training process and realize identity mapping.Our model was trained on 900 subjects' PSGs and validated on 94 subjects' PSGs. And 989 subjects' PSGs were used as test set. Our method achieved an AUPRC of 0.10 on the full test set. There is some room for improvement comparing with other methods.
更多查看译文
关键词
convolutional neural networks,sleep arousal detection,PSG,sleep experts,sleep arousal identification,34-layer convolutional neural network,CNN,feature extraction,feature classification,AUPRC,EEG,electroencephalogram,power spectral density,frequency domain information extraction
AI 理解论文
溯源树
样例
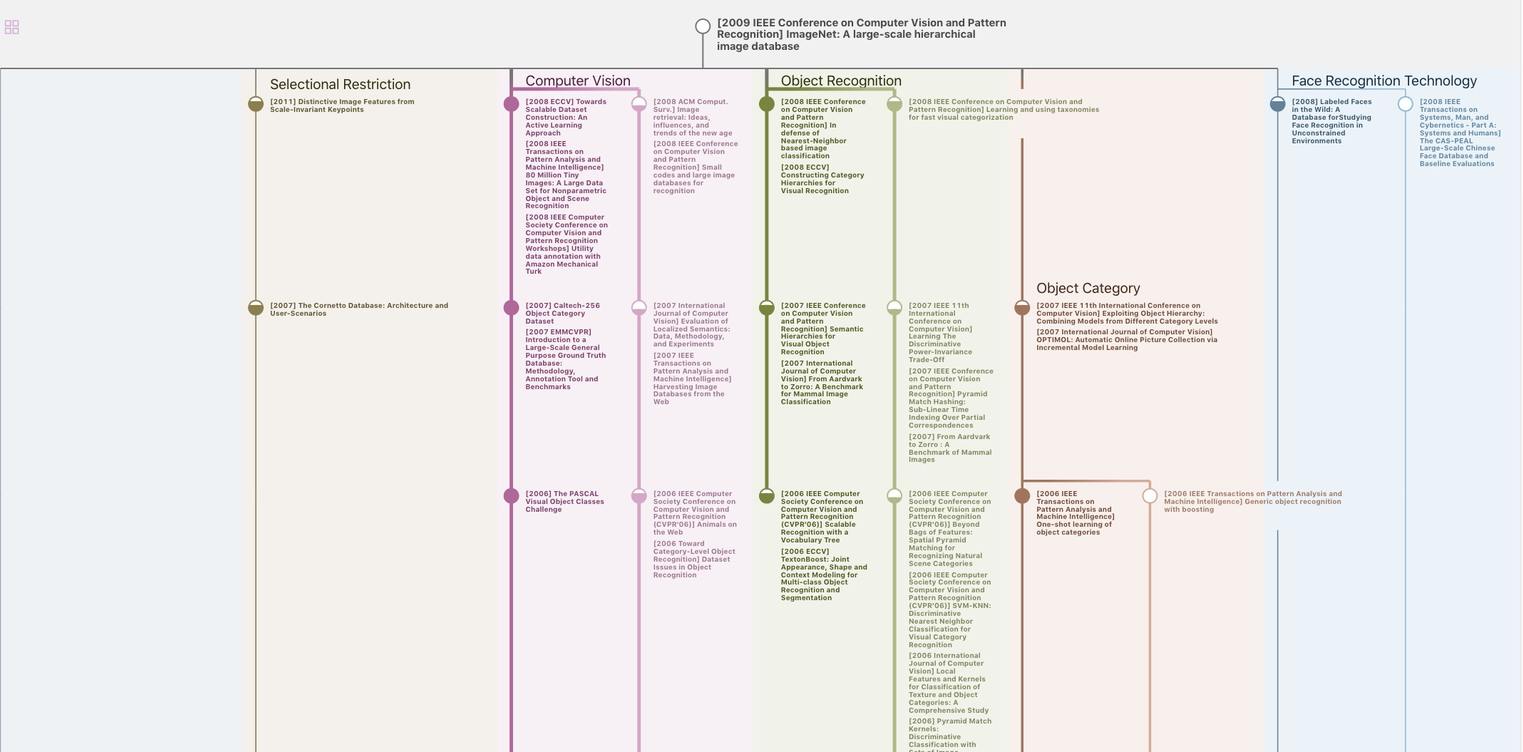
生成溯源树,研究论文发展脉络
Chat Paper
正在生成论文摘要