On Optimal Proactive Caching with Improving Predictions over Time
2018 56TH ANNUAL ALLERTON CONFERENCE ON COMMUNICATION, CONTROL, AND COMPUTING (ALLERTON)(2018)
摘要
This paper considers optimal proactive caching when future demand predictions improve over time as expected to happen in most prediction systems. In particular, our model captures the correlated demand pattern that is exhibited by end users as their current activity reveals progressively more information about their future demand. It is observed in previous work that, in a network where service costs grow superlinearly with the traffic load and static predictions, proactive caching can be harnessed to flatten the load over time and minimize the cost. Nevertheless, with time varying prediction quality, a tradeoff between load flattening and accurate proactive service emerges.In this work, we formulate and investigate the optimal proactive caching design under time-varying predictions. Our objective is to minimize the time average expected service cost given a finite proactive service window. We establish a lower bound on the minimal achievable cost by any proactive caching policy, then we develop a low complexity caching policy that strikes a balance between load flattening and accurate caching. We prove that our proposed policy is asymptotically optimal as the proactive service window grows. In addition, we characterize other non-asymptotic cases where the proposed policy remains optimal. We validate our analytical results with numerical simulation and highlight relevant insights.
更多查看译文
关键词
accurate caching,proactive caching policy,finite proactive service window,time-varying predictions,optimal proactive caching design,accurate proactive service,load flattening,prediction quality,static predictions,traffic load,service cost,correlated demand pattern,prediction systems
AI 理解论文
溯源树
样例
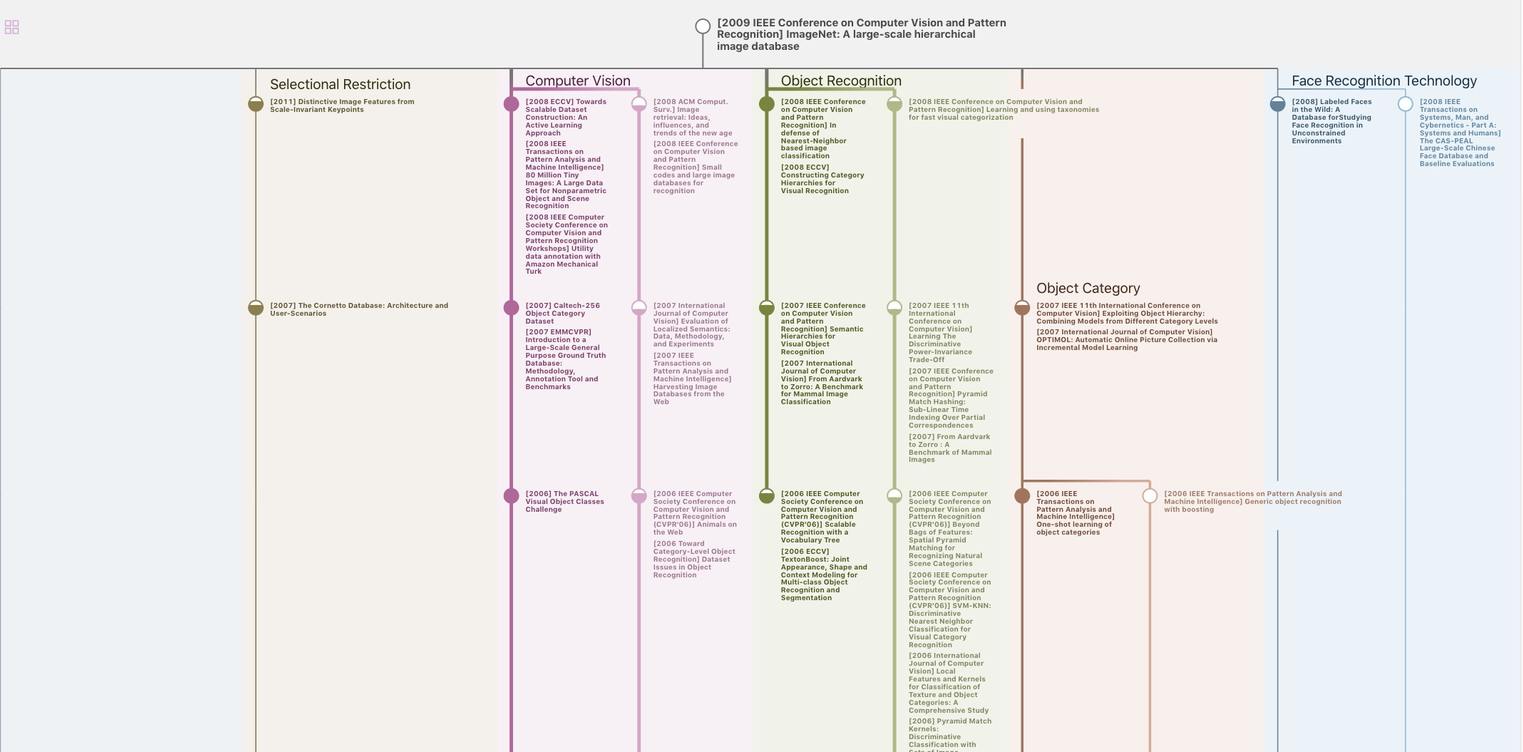
生成溯源树,研究论文发展脉络
Chat Paper
正在生成论文摘要