Fast Nonconvex Deconvolution Of Calcium Imaging Data
BIOSTATISTICS(2020)
摘要
Calcium imaging data promises to transform the field of neuroscience by making it possible to record from large populations of neurons simultaneously. However, determining the exact moment in time at which a neuron spikes, from a calcium imaging data set, amounts to a non-trivial deconvolution problem which is of critical importance for downstream analyses. While a number of formulations have been proposed for this task in the recent literature, in this article, we focus on a formulation recently proposed in Jewell and Witten (2018. Exact spike train inference via l(0) optimization. The Annals of Applied Statistics 12(4), 2457-2482) that can accurately estimate not just the spike rate, but also the specific times at which the neuron spikes. We develop a much faster algorithm that can be used to deconvolve a fluorescence trace of 100 000 timesteps in less than a second. Furthermore, we present a modification to this algorithm that precludes the possibility of a "negative spike". We demonstrate the performance of this algorithm for spike deconvolution on calcium imaging datasets that were recently released as part of the spikefinder challenge (http://spikefinder.codeneuro.org/). The algorithm presented in this article was used in the Allen Institute for Brain Science's "platform paper" to decode neural activity from the Allen Brain Observatory; this is the main scientific paper in which their data resource is presented. Our C++ implementation, along with R and python wrappers, is publicly available. R code is available on CRAN and Github, and pythonwrappers are available on Github; see https://github.com/jewellsean/FastLZeroSpikeInference.
更多查看译文
关键词
Calcium imaging, Changepoint detection, Neuroscience, Nonconvex optimization
AI 理解论文
溯源树
样例
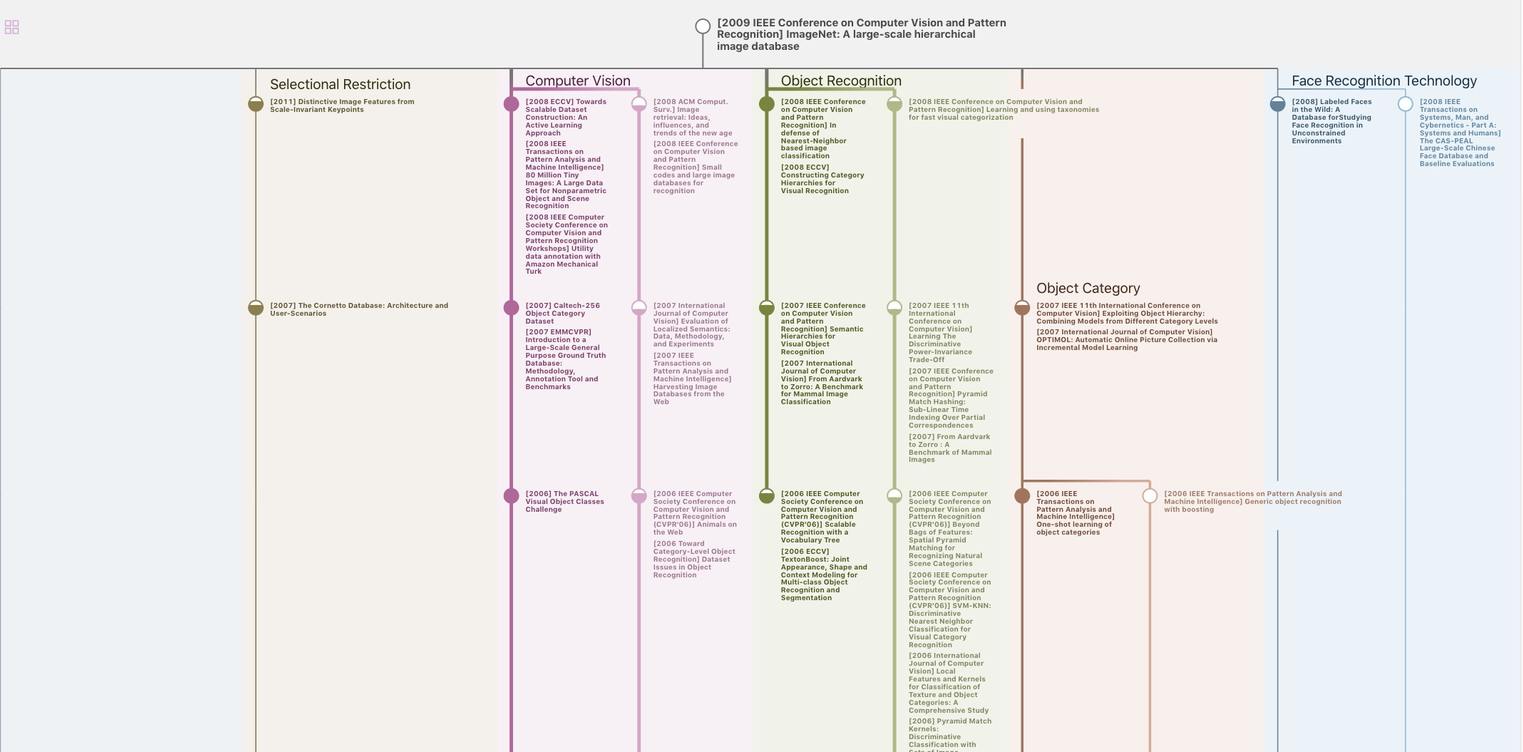
生成溯源树,研究论文发展脉络
Chat Paper
正在生成论文摘要