Improving Disturbance Storm Time Index Prediction Using Linear and Nonlinear Parametric Models: A Comprehensive Analysis
IEEE Transactions on Plasma Science(2019)
摘要
The earth’s magnetosphere is an intricate input–output system, where the inputs are the solar wind parameters and the output measure are the geomagnetic. The disturbance storm time (DST) index is one such quantity measure for the intensity of disturbance in the geomagnetic field due to the magnetic storm. The geomagnetic activities have catastrophic effects on several communication networks and harmful for biological livings. Therefore, it is significant to develop a model for the prediction of disturbance in the geomagnetic field. This paper presents linear as well as nonlinear parametric techniques to model the complex magnetosphere dynamics. Neural network (NN)-based modeling techniques, such as feed-forward NN (FFNN), NN integrated with nonlinear autoregression with exogenous (NARX) inputs, adaptive neuro-fuzzy inference system (ANFIS), and recurrent NN are developed for the prediction of the magnetic storm. Global search algorithms, such as particle swarm optimization and genetic algorithm, also train the developed FFNNs. All the models are trained on 15 years’ real-time series data of solar wind parameters and DST index on a frequency of 1 h. The accuracy of the predicted DST index for multiple intensity levels of the magnetic storm using all developed linear and nonlinear modeling techniques is presented, compared, and analyzed thoroughly. The performance of NN integrated with NARX inputs and ANFIS is higher than rest of methods developed in the paper. Both techniques have the capability to predict mild, moderate, and intense magnetic storm with high degree of accuracy. The comparative analysis with state of the art shows the enhanced accuracy and robustness of developed models in this paper.
更多查看译文
关键词
Indexes,Predictive models,Magnetosphere,Storms,Data models,Analytical models,Artificial neural networks
AI 理解论文
溯源树
样例
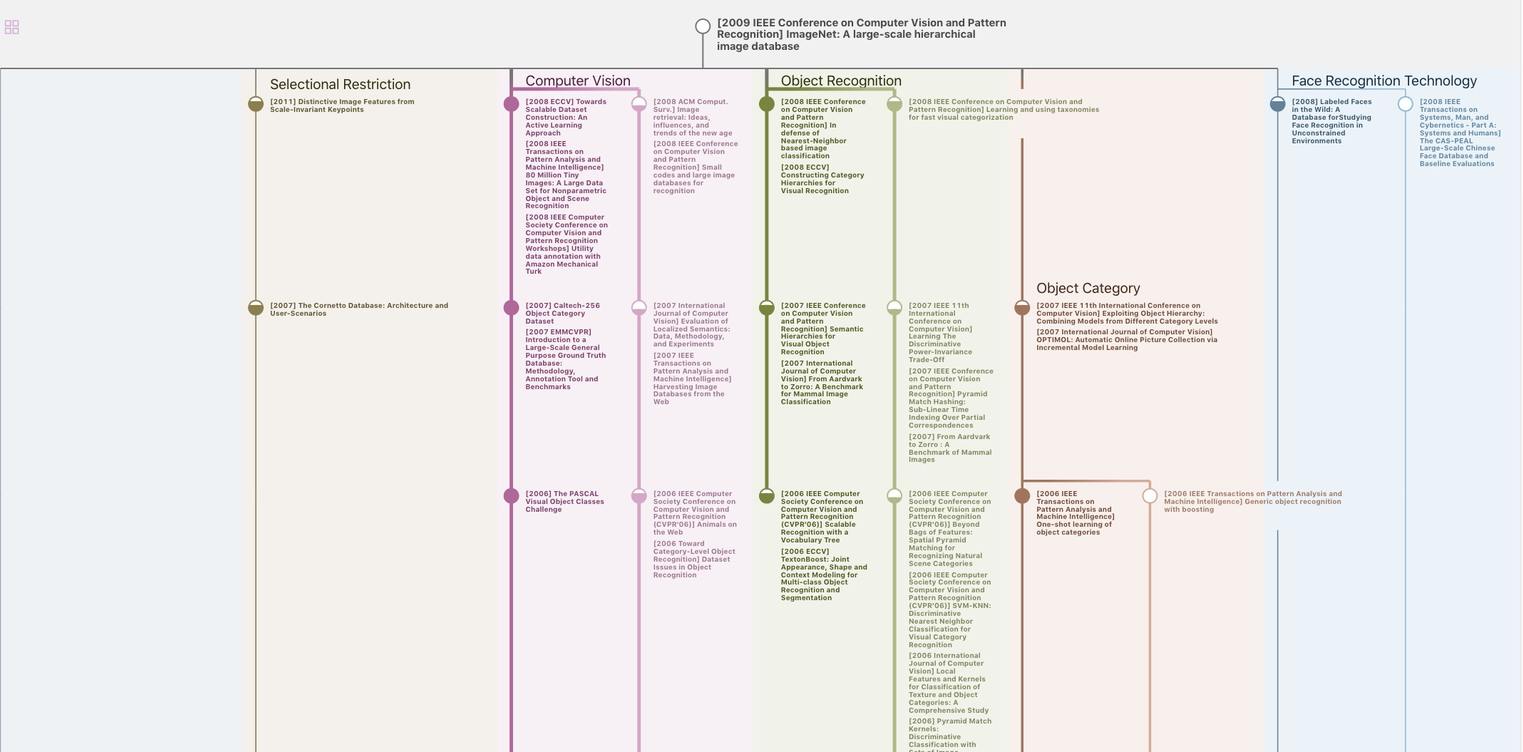
生成溯源树,研究论文发展脉络
Chat Paper
正在生成论文摘要