Online MEM Based Binary Classification Algorithm for China Mobile Imbalanced Dataset
2018 IEEE/CIC International Conference on Communications in China (ICCC)(2018)
摘要
Driven by a plethora of real machine learning applications, there have been many attempts at improving the performance of a classifier applied to imbalanced dataset. In this paper we propose a maximum entropy machine (MEM) based hybrid algorithm to handle binary classification problems with high imbalance ratios and large numbers of features in the datasets. At the training stage, we combine an efficient MEM algorithm with the SMOTE algorithm to build a classifier in a batch manner. At the application stage, the different-cost strategy is incorporated into the MEM algorithm to handle the imbalance learning problem in an online manner. Experiments are conducted based on various real datasets (including one China Mobile dataset and several other standard test datasets) with different imbalance ratios and different numbers of features. The results show that the proposed algorithm outperforms the state-of-the-art algorithms significantly in terms of robustness and overall classification performance.
更多查看译文
关键词
Micromechanical devices,Support vector machines,Training,Entropy,Robustness,Adaptation models,Prediction algorithms
AI 理解论文
溯源树
样例
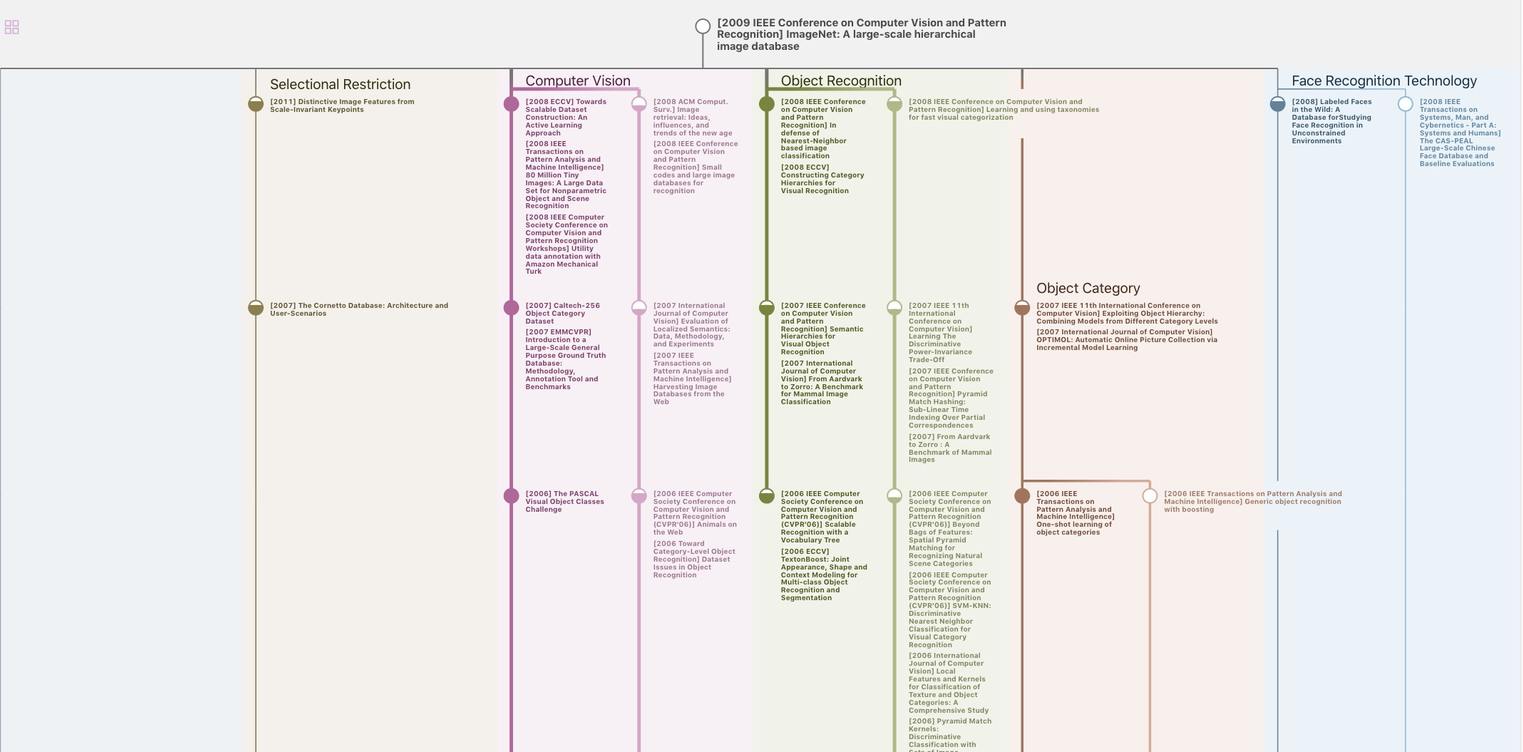
生成溯源树,研究论文发展脉络
Chat Paper
正在生成论文摘要